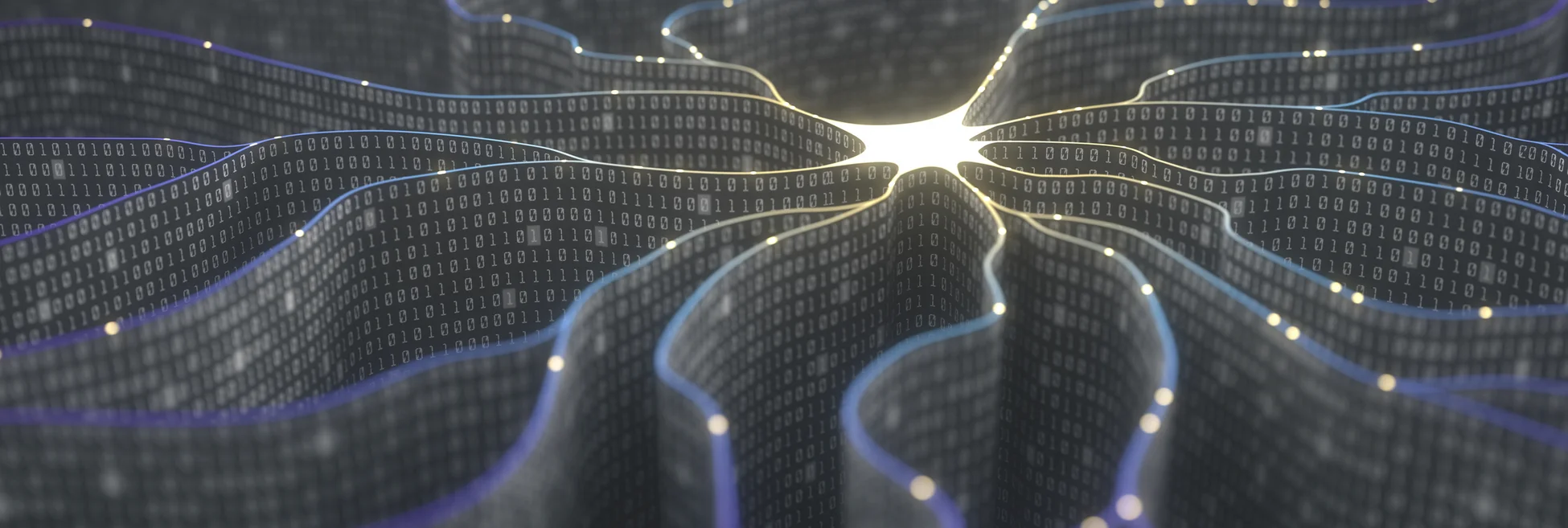
AI and Alternative Data: A Burgeoning Arms Race
By some estimates, investment in alternative datasets will exceed $7 billion by 2020. At the same time, machine learning and other AI techniques are evolving at a rapid pace. The combination of the two will be significant.
Herd mentality has long driven investing. From the 1600s and Tulip Mania to the dotcom boom of the 1990s to the US housing bubble that crippled the global economy this century, irrational exuberance tends to lead to adverse effects.
But what happens when algorithms are leading the herd? Some argue that flash crashes happen. And some believe that regulators need to step in to lessen the risk of chaos. But what if this is more of a data issue, and less of a technology and regulatory issue?
“Algos all depended on market data, which means they reacted to the same type of information or event, which reinforced trends in the market and that’s why you ended up with the Flash Crash,” says Axel Pierron, co-founder and managing director of consultancy Opimas. “Very quickly we’ve realized that the vast majority of the industry is using similar algos that reinforced the herd mentality.”
Alternative Instincts
One interesting phrase entering the lexicon of finance is “alternative data.” At its core, it’s all that data that goes beyond traditional prices and technical analytics. This information has always been out there, but now there are more sources of information available, and it’s easier to get this data, store it and run it through software that makes consuming this unstructured data more efficient.
In recent years, investing in social media signals and sentiment analysis has grown, with varying degrees of success. On the horizon, drone/satellite imagery, coupled with cellphone and credit card usage will continue to grow. Opimas predicts that spending in alternative datasets will exceed $7 billion by 2020.
But despite advancements, this is rough terrain. Waters’ sibling publication Inside Data Management recently looked at quantitative funds’ attempts to sift through the torrent of datasets populating the market. Wesley Chan of Acadian Asset Management—which manages $77 billion in assets and has about two dozen researchers dedicated to finding new signals in alternative datasets—says this is a challenging space to navigate for managers.
“You’re going to have to investigate 90 different things to get 10 that are good,” says Chan, who is the director of stock selection research at Acadian. “A lot of people who aren’t used to those odds will walk away in disappointment, thinking the whole thing is a failure—it’s going to be a lot of waste.”
Another problem facing the sector is that there is already crowding that’s making some firms rethink their strategies. From that IDM story:
The intraday foreign currencies trader has already abandoned the use of weather and agricultural data due to crowding, while the location and load of an oil tanker—used to generate prices by being able to predict supply—is now regarded as a “normal” input of futures and options traders’ oil price or gas price predictions, said Ernest Chan, managing member of quantitative investment management at Canada’s QTS Capital Management.
“Any known information is being exploited by more and more people, so we always constantly have to look for new sources of information to maintain the edge.”
There are, and will continue to be, limiting factors facing the use of alternative datasets, not to mention that these qualitative datasets produce only a handful of data points when compared to quantitative datasets—hence, the need for heavy investment in data scientists to extract value from outputs.
But looking forward, advancements in artificial intelligence (AI) and machine learning will help to augment what a data scientist can do with this information. It can also—potentially—cut down on some of the herd mentality created via the widespread use of similar algos. As deep-learning techniques improve and neural networks become more elegant, Opimas’ Pierron says he believes that differentiation will become more easily achieved because, like a brain, no two neural networks will be the same and the information being fed into those networks—and how the machines are trained—will be similar in some ways, but vastly different in others.
“With AI, you have a very different situation because data sources are much broader,” Pierron says. “The AI you’re using probably won’t react to the same event as someone using other sources of data. With AI, it’s a very different situation because, first of all, data sources are much broader. This means the AI you’re using probably won’t react to the same event as someone else using other sources of data. After that, you have the whole machine learning process to train the AI, which will be different from one another. So you have different sources of data and a trading strategy that’s implemented differently as well. So I think AI will diversify the trading strategies that are going out into the market, rather than have the herd mentality that existed in the past.”
Only users who have a paid subscription or are part of a corporate subscription are able to print or copy content.
To access these options, along with all other subscription benefits, please contact info@waterstechnology.com or view our subscription options here: http://subscriptions.waterstechnology.com/subscribe
You are currently unable to print this content. Please contact info@waterstechnology.com to find out more.
You are currently unable to copy this content. Please contact info@waterstechnology.com to find out more.
Copyright Infopro Digital Limited. All rights reserved.
As outlined in our terms and conditions, https://www.infopro-digital.com/terms-and-conditions/subscriptions/ (point 2.4), printing is limited to a single copy.
If you would like to purchase additional rights please email info@waterstechnology.com
Copyright Infopro Digital Limited. All rights reserved.
You may share this content using our article tools. As outlined in our terms and conditions, https://www.infopro-digital.com/terms-and-conditions/subscriptions/ (clause 2.4), an Authorised User may only make one copy of the materials for their own personal use. You must also comply with the restrictions in clause 2.5.
If you would like to purchase additional rights please email info@waterstechnology.com
More on Regulation
Big questions linger as DORA compliance approaches
The major EU regulation will go live tomorrow. Outstanding clarifications and confusion around the definition of an ICT service, penetration testing, subcontracting, and more remain.
Insurance: The role of risktech in effectively managing emerging risks and driving competitive edge
This whitepaper covers the global survey, conducted by Chartis Research and TCS, of banking, financial services and insurance firms, which found that insurers are struggling to adapt to evolving risks and regulatory requirement increases. Chartis offers…
FX automation key to post-T+1 success, say custodians
Custody banks saw uptick in demand for automated FX execution to tackle T+1 challenges.
Observations and lessons to learn from the move to T+1
The next few years will see other jurisdictions around the world look to North America for guidance on transitioning to shorter settlement cycles.
Expanded oversight for tech or a rollback? 2025 set to be big for regulators
From GenAI oversight to DORA and the CAT to off-channel communication, the last 12 months set the stage for larger regulatory conversations in 2025.
DORA flood pitches banks against vendors
Firms ask vendors for late addendums sometimes unrelated to resiliency, requiring renegotiation
In 2025, keep reference data weird
The SEC, ESMA, CFTC and other acronyms provided the drama in reference data this year, including in crypto.
Waters Wavelength Ep. 299: ACA Group’s Carlo di Florio
Carlo di Florio joins the podcast to discuss regulations.