Generative AI is here to stay, even if the path forward is unclear
Chatbots and large language models dominated the AI conversation this year with the expectation that 2024 will bring more innovation and use cases to the forefront.
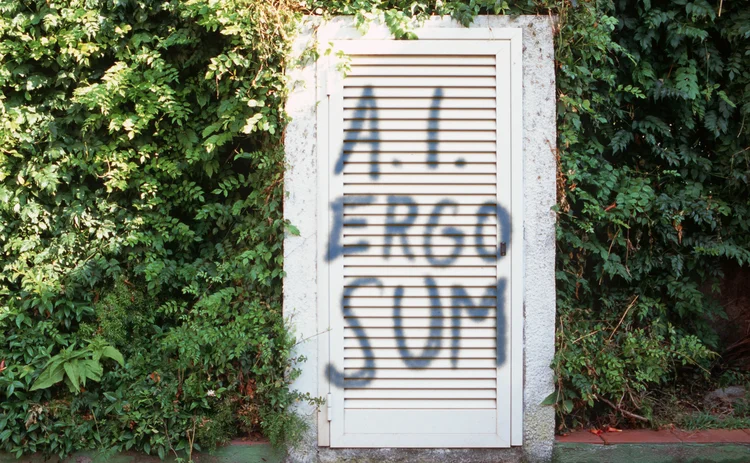
If you’ve been under a rock for the last year, then you’ve missed out on a year that will likely go down in the history books as a defining year for artificial intelligence. Off the heels of OpenAI showcasing ChatGPT late in Q4 2023, you couldn’t scroll LinkedIn and Twitter (now known as X) feeds without seeing some discourse around the chatbot and the technology that underpins it. Since then, firms and vendors across capital markets have been looking to implement generative AI and large language models across their tech stacks.
Here’s a quick synopsis of the technology. Generative AI is simply a subset of AI that can create text, images, and other forms of content. OpenAI’s ChatGPT offers a conversational interface where it can be asked a question, and it will answer with a human-speech-like response that it generates from connecting patterns based on its training data. Large language models are AI models trained on huge swaths of text with the goal of being able to make connections and recognize patterns that will allow it to do specific tasks.
ChatGPT was built atop the the GPT-3.5 large language model (LLM) developed by OpenAI and has been trained using both supervised and reinforcement learning techniques. GPT-3.5’s predecessor, GPT-3 or Generative Pre-trained Transformer 3, was trained on a gargantuan dataset—175 billion machine-learning parameters. In March, GPT-4 was rolled out, and with each iteration, the machine’s “cognitive” abilities improve.
One of the early observations among capital markets industry observers and participants in January was that we could see large language models being trained on industry-specific data. “The solution [ChatGPT] as it is today, it’s built upon a large amount of public information,” Tim Baker, founder of consultancy Blue Sky Thinking and financial services practice lead at software provider Expero, told me in January. “And I think what we’re going to see is the same technology being trained on both industry data—or industry vertical data—and also private data, so that it can become a much deeper expert in certain areas—like, for example, financial services.”
Those industry-specific models started to pop up this year. In March, Bloomberg released the research paper for its large language model, BloombergGPT. BloombergGPT, while not a chatbot, will help to assist—and provide more depth to—the Terminal’s sentiment analysis, named-entity recognition (NER), news classification, charting and question-answering capabilities, among other functions.
Shawn Edwards, Bloomberg’s CTO, told WatersTechnology in March that, initially, the enhancements to the Terminal will be more behind the scenes. “This is all very early days and we’re just scratching the surface—this is really Day One,” he said. “But the dream—and we’ve been working on this for years—has been for a user to walk up to the Terminal and ask any question and get an answer or lots of information; to get you to the starting point of your journey.”
Other data and information vendors like Moody’s and FactSet have also dipped their toes in the generative AI pool. In June, Moody’s announced a partnership with Microsoft focused on generative AI that will look to enhance its current offerings, one of which is through Moody’s CoPilot, an internal tool that will combine proprietary data and research under Moody’s Analytics with LLMs and AI technology that sits under Microsoft. Sergio Gago Huerta, managing director of AI and machine learning at Moody’s Analytics, told WatersTechnology in July that the platform will offer a secure way to leverage LLMs. Posing questions related to financial markets to ChatGPT can be difficult due to the chatbot’s knowledge cutoff and the fact that it can “hallucinate” false information. “That’s exactly why we call these things co-pilots and not pilots. It’s critical that there’s a human in the loop, validating and verifying the content,” Huerta said.
Last month, FactSet outlined its generative strategy. The vendor is taking a three-pillar approach to AI: mile-wide discoverability, mile-deep workflow automation, and mile-high innovation acceleration. FactSet will look to include a new conversational experience for users within its FactSet Workstation offering where a user can ask a question and have FactSet surface the answer or guide the user to the right place. If a user is looking for data that FactSet might not have, the interface can also answer with multiple datasets to give a more comprehensive answer. This month, the vendor rolled out the beta release of FactSet Mercury, a large language model-based knowledge agent.
Outside of data providers, generative AI and LLMs are also attracting attention in the trading space. Broadridge’s LTX electronic bond trading platform introduced its BondGPT offering this year. The generative AI-powered tool aims to answer traders’ bond-related questions and assist them in the identification process of corporate bonds on the platform. Jim Kwiatkowski, chief executive officer of LTX, told WatersTechnology in October that the platform was the byproduct of internal conversations and requests from clients to create a dashboard that provided them with more data while taking up minimal screen real estate.
Others are still in a testing phase with the technology. Tradeweb is currently in the pilot phase of its smart dealer-selection tool, AiSNAP, which leverages generative AI to help clients select liquidity providers to reduce the resulting transaction cost of an RFQ. Using AiSNAP, clients can improve execution quality and reduce transaction costs. Taking deep learning models trained on Tradeweb’s extensive historical trade information, in combination with proprietary optimization algorithms, AiSNAP was able to suggest a better dealer than the best streaming dealer included in 59% of cases across a sample of RFQs from the fourth quarter of 2022.
The Big Tech question
Just as capital markets firms have looked to Big Tech companies to power their cloud strategies, it’s also become clear this year that they are largely doing the same for generative AI. While Microsoft clearly had a head start with their OpenAI partnership (more on that later), Amazon Web Services (AWS), Google, and IBM have all rolled out their own generative AI offerings in the last few months. Second quarter earnings calls from all four laid bare that they see generative AI as an important component of their strategies going forward.
Monica Summerville, head of capital markets technology research at consultancy Celent, told me in August that this move makes sense, and it can be tied back to one simple thing—data. “As a cloud provider, I think this is absolutely something they’re going to have to do. At the end of the day, they’re trying to increase the amount of compute and services they offer that runs through the cloud,” she said.
From a bird’s eye view, all four providers appear to be doing the same thing: providing models for their clients to be able to build tools on. I spoke to three of the providers over the summer (AWS, IBM, and Google) and at the center of all three providers’ offerings is the idea of optionality. Foundational models, as they’ve commonly become known as, allow users to build their own AI tools, whether it’s a chatbot or a digital assistant.
In April, AWS launched Bedrock, a platform that allows the scaling and building of AI applications from foundation models provided by both Amazon and third-party providers. Those foundational models are accessed through an API, and users can then connect their data to these models without training them. The models that are currently available include Amazon’s own Titan, as well as others from AI startups like Anthropic, Stability Diffusion, and AI21 Labs. Google’s Vertex AI experimentation platform offers users what they call a model garden with Google proprietary models, open-source models, and models from third-parties. Google’s models include PaLM for both text and chat, Imagen for image generation, and Codey for code completion. Open-source models include BERT, among others. IBM is building on the AI legacy of Watson with the watsonx platform, which offers IBM-trained models as well as models from HuggingFace.
All three have recognized that giving choice is the best strategy—the notion that there will be one model to rule them all is unlikely to transpire. That notion couldn’t be clearer after the events that transpired at OpenAI in November.
Most of the world was gob smacked on the evening of November 17 when news broke that the OpenAI board had ousted its co-founder and chief executive officer Sam Altman. Altman had become the face of the startup since ChatGPT’s release and had become synonymous with AI’s newest wave. The four board members announced the move in a blog post and shared that after “a deliberative review process by the board,” they had concluded that Altman was not “consistently candid” in his communications with the board. Mira Murati, who was serving as the company’s CTO, would become interim CEO.
Chaos quickly ensued. Reporting quickly emerged that Microsoft, OpenAI’s notable big tech partner and biggest champion, were only made aware of the leadership change minutes before the world was. In January, Microsoft and OpenAI extended their existing partnership with reports that the tech giant has a $13 billion investment and 49% stake in the company. Despite all this, Microsoft did not have a seat on the board.
The board also removed Greg Brockman, who was co-founder and president, as chairman of the board. He quickly resigned as president, and this was followed by additional resignations in the research team. By the next day, it was reported that Altman was in negotiations to return but 24 hours later those conversations failed and another interim CEO was named. On November 20, Microsoft CEO Satya Nadella announced that Altman and Brockman were joining Microsoft to lead a research team for advanced AI and that the company was still committed to OpenAI. But that same day, almost 95% of OpenAI’s employees threatened to quit and join Microsoft if Altman wasn’t reinstated. OpenAI’s very existence hung in the balance.
But that fate only dangled for so long as Altman and Brockman returned to OpenAI on November 21 with a new board. On November 29, Microsoft joined the OpenAI board as a non-voting member.
For the firms who have built new tools and platforms on OpenAI’s models over the last year, I have to imagine that those four days had to be nerve wracking. Here was a tech provider that numerous companies had invested in, coming undone at the seams out of nowhere. Yes, it had Microsoft’s backing and support, but it was still showing significant cracks. If optionality is going to be a pinnacle of how companies approach AI, here is a real-life scenario playing out to prove how important that will be going forward.
Many of the people I’ve talked to about generative AI and large language models have largely said a version of the same thing: it’s early days on this stuff. Yes, the Big Tech providers are showing off their shiny new tools and are looking to entice firms to choose their models over their competitors. And yes, some of the biggest vendors in this space are flexing their muscles in building out genAI and LLM powered platforms. But caution and care are as important as ever.
Jeremy Stierwalt, senior partner and US head of data and analytics at Capco, told me in November that starting small might be the optimal road to take. “Much like any other new capability, fail fast and fail cheap because we’ve seen clients that are broadly going after it in a much larger capacity and not put the right controls in place,” he said.
In other words, slow and steady likely wins the race.
Only users who have a paid subscription or are part of a corporate subscription are able to print or copy content.
To access these options, along with all other subscription benefits, please contact info@waterstechnology.com or view our subscription options here: http://subscriptions.waterstechnology.com/subscribe
You are currently unable to print this content. Please contact info@waterstechnology.com to find out more.
You are currently unable to copy this content. Please contact info@waterstechnology.com to find out more.
Copyright Infopro Digital Limited. All rights reserved.
As outlined in our terms and conditions, https://www.infopro-digital.com/terms-and-conditions/subscriptions/ (point 2.4), printing is limited to a single copy.
If you would like to purchase additional rights please email info@waterstechnology.com
Copyright Infopro Digital Limited. All rights reserved.
You may share this content using our article tools. As outlined in our terms and conditions, https://www.infopro-digital.com/terms-and-conditions/subscriptions/ (clause 2.4), an Authorised User may only make one copy of the materials for their own personal use. You must also comply with the restrictions in clause 2.5.
If you would like to purchase additional rights please email info@waterstechnology.com
More on Emerging Technologies
On GenAI, Citi moves from firm-wide ban to internal roll-out
The bank adopted three specific inward-facing use cases with a unified framework behind them.
How a Chinese AI firm shook the tech world
DeepSeek’s AI model is the very ethos of doing what you can with what you have.
To unlock $40T private markets, Hamilton Lane embraced automation
In search of greater transparency and higher quality data, asset managers are taking a tech-first approach to resource gathering in an area that has major data problems.
Waters Wavelength Ep. 304: Nice Actimize’s Lee Garf
Lee joins to discuss changes in communication platforms over the last few years.
DTCC revamps data distribution, collection efforts with cloud, AI
The US clearinghouse is evaluating the possibilities that cloud and AI offer to streamline the processes by which it collects and makes data available to market participants.
Agentic AI and big questions for the technologists
Waters Wrap: Much the same way that GenAI dominated tech discussions over the last two years, the road ahead will feature a lot of agentic AI talk—and CIOs and CTOs better be prepared.
Waters Wavelength Ep. 302: Connectifi’s Nick Kolba
Nick joins the show to give his views on trends in the interoperability space and the FDC3 standard.
AI co-pilot offers real-time portfolio rebalancing
WealthRyse’s platform melds graph theory, neural networks and quantum tech to help asset managers construct and rebalance portfolios more efficiently and at scale.