AFTAs 2018: Best Analytics Initiative—Credit Suisse
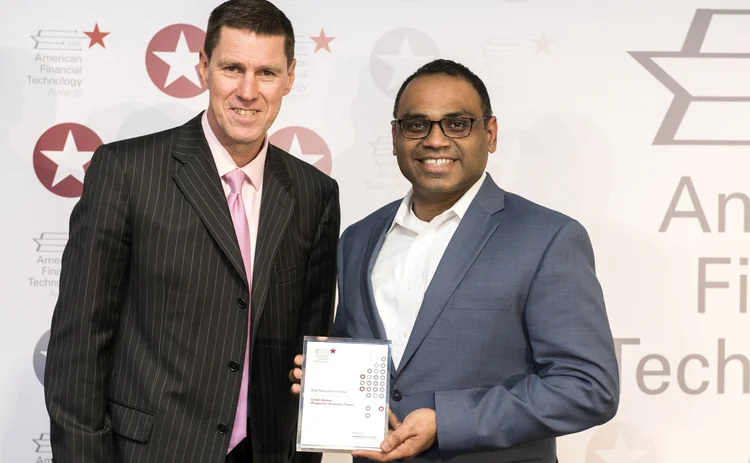
Artificial intelligence (AI) may be all the rage at the moment, but less-often mentioned is a subset-of-a-subset on the bleeding edge of technological development—deep learning. Here, Credit Suisse shines for delivering actionable insights based on its work in natural-language processing and deep learning, via its analytics platforms, and wins the AFTA for its efforts.
One of the major problems facing equities research analysts isn’t necessarily information shortage—it’s too much information. The volume of emails and notes both generated and received by staff on these teams is immense—in Credit Suisse’s case, its Global Markets Equities Research Team has to deal with over 3 million emails and 500,000 meeting notes—but finding an efficient way to analyze these client communications has always been challenging.
Enter AI. The goal of the project was to deliver insights around four main areas: topic modeling, in which potential sales ideas could be generated around stocks or bonds that may be potentially of interest to clients; ticker tagging, in order to identify said instruments for future reference; contact-level sentiment analysis, to determine overall perception of products and services; and auto-summarization, which would enable the machine-learning algorithm to generate commentary on a client.
Implementing this required an element of trial-and-error at first, partly because this is breaking such new ground within actual implementations of machine-learning technology. “We tried various models, such as random forest and a few others, and we obviously wanted to give deep learning a shot because we had enough data to try this out,” says Paras Parekh, head of the predictive analytics team within the global markets technology group at Credit Suisse. “It happens that the deep learning model works fairly well compared to random forest.”
The work so far has resulted in the development of two dashboards which, the bank says, have generated “actionable insight.” The first displays an email or meeting note which has been through the algorithm, cleaned and tagged appropriately, along with sentiment. The second displays time-series data at a contact level, and the top entities by mention, as well as trends by sentiment score. All of this, too, while the platform is still effectively being built.
“We’re still fine-tuning the model. It’s giving us enough insight, but there’s still some work needed, especially when we’re looking to find cross-selling opportunities,” says Parekh. “But if we had to look for investment ideas for stocks that the client may be interested in, that’s working quite well. We’re also able to do a fair bit of entity recognition and trying to understand all the various entities involved for an individual or within a group at a client level or a macro level.”
Only users who have a paid subscription or are part of a corporate subscription are able to print or copy content.
To access these options, along with all other subscription benefits, please contact info@waterstechnology.com or view our subscription options here: http://subscriptions.waterstechnology.com/subscribe
You are currently unable to print this content. Please contact info@waterstechnology.com to find out more.
You are currently unable to copy this content. Please contact info@waterstechnology.com to find out more.
Copyright Infopro Digital Limited. All rights reserved.
As outlined in our terms and conditions, https://www.infopro-digital.com/terms-and-conditions/subscriptions/ (point 2.4), printing is limited to a single copy.
If you would like to purchase additional rights please email info@waterstechnology.com
Copyright Infopro Digital Limited. All rights reserved.
You may share this content using our article tools. As outlined in our terms and conditions, https://www.infopro-digital.com/terms-and-conditions/subscriptions/ (clause 2.4), an Authorised User may only make one copy of the materials for their own personal use. You must also comply with the restrictions in clause 2.5.
If you would like to purchase additional rights please email info@waterstechnology.com
More on Awards & Rankings
Buy-Side Technology Awards 2024 winner’s interview: SimCorp
SimCorp won the Best buy-side investment book of record (Ibor) platform category in this year’s Buy-Side Technology Awards. Christian Kromann, CEO of SimCorp, discusses the win.
BST Awards 2024: Best buy-side data management product—NeoXam
Product: NeoXam DataHub
BST Awards 2024: Best buy-side execution venue—360T
Product: 360T
Buy-Side Technology Awards 2024: All the winners and why they won
The 39 category winners of the 2024 Buy-Side Technology Awards.
BST Awards 2024: Best buy-side performance measurement and attribution product—FactSet
Product: FactSet Performance Solutions
BST Awards 2024: Best buy-side newcomer (vendor or product)—Exegy
Product: nxFramework
BST Awards 2024: Best buy-side managed data service—S&P Global Market Intelligence
Product: iLEVEL Managed Data Services