Elephant in the room: Balancing AI innovation with cloud projects
Voice of the CTO: While generative AI is today’s hot topic, bank technologists explain how they’ve spent years planning for this day, even if cloud had been the main focus. And to be sure, skeptics remain.
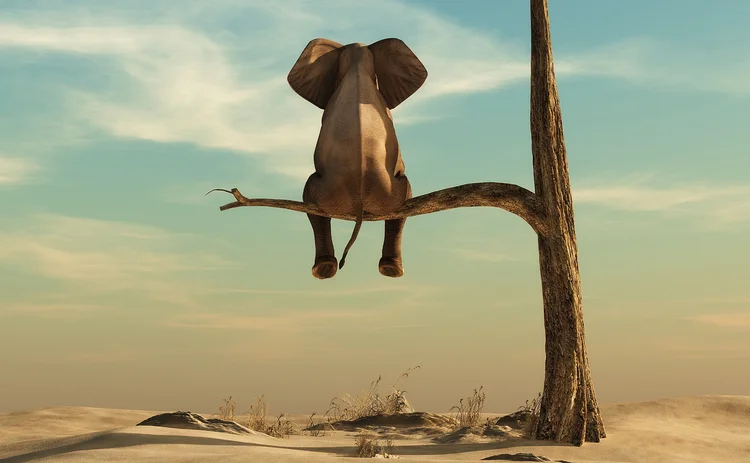
Voice of the CTO
This article is the third in our new, annual examination of the spending plans of bank technology leaders. Parts one and two of this five-part series can be found here.
The goal is to shine a light on opportunities that some of the world’s biggest banks are trying to grasp—and the obstacles that stand in their way. Some of those hurdles are internal—winning support from the business for important-but-unglamorous refits, for example. Others are external, such as keeping up with the sheer pace of change. And at the core of everything is data.
Part four, which will be published on Wednesday, February 14, will examine data management and governance issues. And part five will look at interoperability efforts across the front, middle, and back offices.
See the Methodology box below for more details on how the series was created.
In June of 2023, the most senior technologists at a global tier-one bank gathered before the firm’s board of directors. The aim was to lay out the team’s tech roadmap for 2024 and beyond.
According to the bank’s chief operating officer, in years past these meetings have traditionally revolved around talent retention and acquisition, shared services among the bank’s various siloes, cybersecurity, the cost of data, and regulation.
This latest get-together was not like the others.
“All the board wanted to talk about was cutting-edge artificial intelligence and, specifically, machine learning, large language models, and generative AI,” says the COO.
The head of technology and engineering at a second global tier-one bank sings a similar tune.
“I would have to say that for the year of 2023, AI was almost exclusive to everything we did,” the second executive says. “Of course, we’re delivering on regulatory obligations and long-term projects, but in tandem with that we’ve put an uber amount of focus on leveraging that data and taking in the advanced side of AI—i.e., generative, large language models, and cognitive compute aspects. We have accelerated that significantly in 2023, and it’s going to be a big story for 2024.”
As noted previously in this series, there’s always a push–pull effect on IT budgets between changing the bank and running the bank. And if you don’t get your enterprise architecture right and modernize legacy systems, there’s only so much that you can do on the AI innovation front. But if the C-suite is paying extra attention to genAI and LLMs, it’s worth exploring how some of the largest banks in the world are getting their information systems in line to take advantage of these new tools.
‘Build, buy, borrow’
The chief technology officer at a third global tier-one says their bank’s journey to AI innovation began in earnest about five years ago. When it comes to the firm’s capital markets tech projects, they say roughly 75% of its trading algorithms now incorporate various forms of machine learning. They note, though, that most forms of ML are not so-called “black boxes”.
For example, decision trees, linear regressions, and logistic regressions—and the decisions they make—are more easily explained to regulators, as opposed to deep-learning neural networks, which are, by definition, black boxes. But even here, genAI is helping with explainability, said Deutsche Bank’s chief innovation officer, Gil Perez, in an interview back in November. [Editor’s note: Perez was not interviewed for this series.]
The chief technology officer at the third bank notes that while AI has been a journey, the amount of attention these projects receive varies year-to-year—for 2024, a major focus will be on pairing commodities trading with ML algorithms, specifically for precious metals. Five years ago, commodities were not on the AI agenda.
“In credit, five years ago it was all manual, voice business; today, most of that business is electronic,” says the CTO. “So, for example, how do we distribute prices more effectively? How do we do portfolio trading in credit more effectively? A lot of the money we spend is on capabilities that emerge and evolve over time depending on how the market moves and client demands.”
But the CTO is excited about genAI due to its potential to remove the donkey work from mundane, but necessary, tasks. For instance, if there are 50,000 term sheets inside the bank, and someone wants to find all of the clients that have a particular clause in a term sheet, genAI will help pull that information forward for a human to verify. If you want to use genAI to do parts of coding, that can create a 30% productivity boost among the engineering core, they estimate.
The CTO warns, though, that genAI and LLMs are not panaceas—bad data in, bad data out. So for every “AI project” the bank has planned, the first step has to be modernizing/re-platforming legacy systems. They also explore whether more traditional forms of AI are better equipped—and, ideally, cheaper—to solve the problem. Wrapping that all together are well-defined data governance practices.
Additionally, (as everyone likes to say) the bank’s technologists work with the business, which will articulate a problem, such as the need to price an illiquid market. If that’s the problem they’re trying to solve, there are many ways to do so, says the CTO. “One, we could take historical prices, and through very simplistic math publish that back. Or, we can use ML techniques that allow us to target a certain risk ratio or client base. So in rates, we use ML to solve that problem.
“For us, machine learning is just seen as a tool; we don’t go after it because it’s machine learning. We look at a problem and say, ‘What’s the best way we can solve that problem?’ and then we apply the best technique to it.”
For the CTO, the strategy comes down to the “three Bs”: build, borrow, and buy. What is the bank’s internal tech team most competent in? Well, the team will build that. If that particular team doesn’t have the competency to build something, but another part of the bank has already solved for the problem, it gets “borrowed”. And, finally, rather than be a hammer looking for a nail, if the expertise doesn’t exist in the bank, they go out and buy it rather than waste money creating a bootleg version of something a vendor has already solved for. Sometimes those tools are based on AI; other times simply turning to low-code development will solve the issue. The question is always: What is the best tool?
“The only place where we will force a build is where we think we have very strong IP,” says the CTO. “We have some algorithms, some quantitative model we are applying—in those scenarios we will go for an internal build.”
The skeptics
“When I read articles about this or that bank using machine learning, in the back of my head, I think, if they’re being honest, they’re talking about glorified robotic process automation,” says the CIO of corporate and investment banking technology at a fourth tier-one bank. “Maybe the very largest banks can poach elite engineering talent, but I even doubt that.”
It’s an age-old lament for bank technologists; there’s too much red tape, and the list of regulations seemingly grows by the day, which makes it harder to attract top-tier talent. If you’re a talented AI engineer, are you going to work under those conditions? Would you rather work at Google or partner with Google?
They acknowledge that for analytics and customer service, AI is vital, but the bank outsources a large piece of that—“90%, but that’s also an impossible number to put an accurate figure around.”
And unlike that first tier-one technologist mentioned at the start, this tech exec hasn’t seen the budgetary faucets open up for advanced forms of AI. “It’s not like, ‘Oh, I have a budget to use GPTs (generative pre-trained transformers) or LLMs,’—no, not at all,” they say. “I mean, there’s nothing wrong with budgeting that—especially if you have the luxury of having a bit more of a ‘riskier budget’—but it’s an R&D budget.”
At the end of the day, the most important thing is “contextualization and calling a spade a spade,” they say. “That’s why in every single presentation I give to the C-suite or vendors, I only ever want to talk about data. I always say AI has nothing to do with data, much to the dismay of the vendors of data and AI.”
What they mean is that, yes, it’s all about achieving a business objective, but that usually means breaking down siloes across the organization, improving data governance, and creating interoperability between the bank’s internal and third-party systems.
For the CIO at a fifth tier-one bank, they’ve found that the AI conversation is pulling the firm away from less sexy enterprise architecture projects and what they see as the bank’s most pressing need—getting as many applications as possible onto the cloud in an expedient—but safe—manner.
Since the Financial Crisis, banks have been on a “cost-reduction journey—I think we’ve kind of run out of road,” they say, adding that banks often take their eyes off the ball depending on which way the wind blows. One needs to only look at the layoffs on Wall Street over the past year—all while touting that they’re tech companies and AI-enabled—as proof.
Every bank has too many applications where there isn’t interoperability or where there’s overlap. Sources say that for all the talk of AI, there are still far too many manual workflows, often at the cost of the user experience, both internal and external. Cloud forms the foundation for being able to incorporate new tools, whether that’s machine learning, APIs, open-source, or cutting-edge analytics systems.
While it’s important to have buy-in from the business, says the CIO at the fifth bank, cloud is a tougher sell: “Hey, we’re migrating applications to Google Cloud, isn’t that great?!” The answer is often, “What’s the difference?”
So if a bank decides to spend $2 million to migrate its matching engine from running on-prem to the cloud, it’s not always easy to explain the tangible benefits to the business. On the other hand, that $2 million can be spent on developing a chatbot using genAI underpinned by an LLM that allows someone on the business side to more easily chat with a customer and make recommendations based off of previous decisions made at the bank.
“It’s a hard sell,” says the fifth bank’s technologist. “Moving to the cloud doesn’t give you anything that’s tangible in terms of capability. You have to sell the fact that it’s more scalable. If you have a really busy day, you can scale up just like that, compared to before, where you’d have to buy 10 more servers and you’d have to wait six months. It’s about scalability and stability.”
Safety and scalability versus client experience and efficiency. While they articulate the issue in different ways, the sources for this story agree that these are not mutually exclusive—but can you convince the pullers of the purse strings of that? The issue, they say, is that you have to manage expectations around exploring new technologies, versus explaining the long-term benefits of modernizing legacy systems, establishing proper data governance structures, and improving the bank’s enterprise architecture.
Going back to the COO at the first tier-one bank whose board only wanted to talk about AI, they also note that there was a time when AI was an unusual topic for the board to digest. And similarly, it wasn’t that long ago that the idea of using one of the major public cloud providers for sensitive client information was anathema to the C-suite.
What’s clear is that the rate of technological evolution is increasing exponentially. If you want your house to incorporate the latest-and-greatest additions and features, you had better make its foundation invulnerable.
Methodology
The “Voice of the CTO” series is based on interviews conducted by WatersTechnology with eight CTOs from a selection of tier-one international banks that took place between October and December of last year. For clarity, the term “CTO” is a catchall that includes chief information officers and various other global heads of capital markets technology—people whose focus is on the corporate and investment bank and who handle a budget. In the story, we will address the individual’s job title, but have granted them anonymity so that they can speak openly about their organization and beliefs.
The series also draws on a limited-circulation survey of handpicked technology end-users, and incorporates new market sizing work by WatersTechnology sibling brand Chartis Research.
We plan to repeat the exercise later this year, and are looking for feedback. If you have any comments or questions, please get in touch: anthony.malakian@infopro-digital.com
Only users who have a paid subscription or are part of a corporate subscription are able to print or copy content.
To access these options, along with all other subscription benefits, please contact info@waterstechnology.com or view our subscription options here: http://subscriptions.waterstechnology.com/subscribe
You are currently unable to print this content. Please contact info@waterstechnology.com to find out more.
You are currently unable to copy this content. Please contact info@waterstechnology.com to find out more.
Copyright Infopro Digital Limited. All rights reserved.
As outlined in our terms and conditions, https://www.infopro-digital.com/terms-and-conditions/subscriptions/ (point 2.4), printing is limited to a single copy.
If you would like to purchase additional rights please email info@waterstechnology.com
Copyright Infopro Digital Limited. All rights reserved.
You may share this content using our article tools. As outlined in our terms and conditions, https://www.infopro-digital.com/terms-and-conditions/subscriptions/ (clause 2.4), an Authorised User may only make one copy of the materials for their own personal use. You must also comply with the restrictions in clause 2.5.
If you would like to purchase additional rights please email info@waterstechnology.com
More on Trading Tech
Hyperscalers and capacity issues, Bridgewater’s CEO on AI, the UK’s Pisces platform, and more
The Waters Cooler: AI and cloud—shockingly—were major talking points this week…as they were the weeks before, and likely the weeks to come.
Orchestrade resists SaaS model in favor of customer flexibility
Firms like Orchestrade are minimizing funds and banks’ risks with different approaches to risk management.
Pisces season: Platform providers feed UK plan for private stock market
Several companies in the US and the UK are considering participating in a UK program to build a private stock market composed of separate trading platforms.
Hyperscalers to take hits as AI demand overpowers datacenter capacity
The IMD Wrap: Max asks, who’s really raising your datacenter costs? And how can you reduce them?
New FPGA component aims to curb co-lo costs
Hardware ticker plant provider Exegy is working on a new FPGA solution that it says will free up costly processing power on firms’ existing co-lo servers.
Market data woes, new and improved partnerships, acquisitions, and more
The Waters Cooler: BNY and OpenAI hold hands, FactSet partners with Interop.io, and trading technology gets more complicated in this week’s news round-up.
Asset manager Fortlake turns to AI data mapping for derivatives reporting
The firm also intends to streamline the data it sends to its administrator and establish a centralized database with the help of Fait Solutions.
The murky future of buying or building trading technology
Waters Wrap: It’s obvious the buy-v-build debate is changing as AI gets more complex, but Anthony wonders how trading firms will keep up.