Rise of the Machines: How Machine Learning is Changing the Capital Markets
Machine learning within the buy side is only set to grow, but getting data right is just the start.
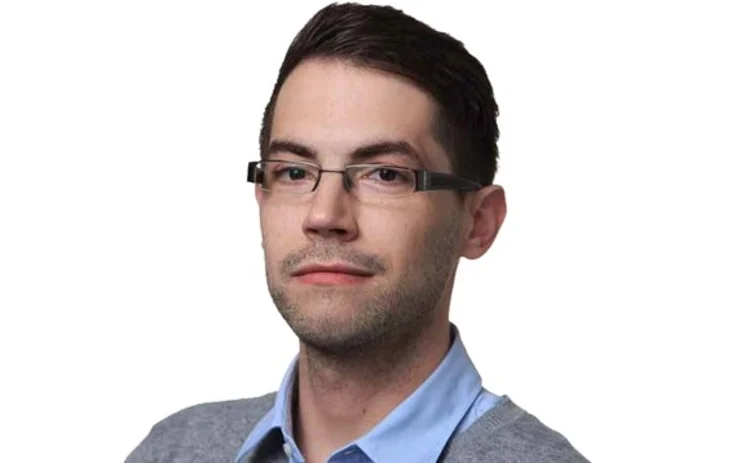
The technology-minded capital markets participants do love a good trend. If 2014 was centered around “IBOR-mania” and 2015 was the year that the blockchain exploded into the hyperbole stratosphere, then the major trend of 2016 will arguably be machine learning.
It may sound like the sexy new foundation upon which so much of asset management’s future can be built, but at heart, machine learning is a data efficiency play, one that many on the buy side are still unable to get right for a variety of reasons.
While no one wants to get left behind when it comes to the application of new technology, in this case it really will be those firms that have come to grips with the questions posed by big data management that will have the head start in machine learning.
One of the key distinctions to make on this subject is the differentiation between machine learning and artificial intelligence; the former is concerned with automating processes and associated troubleshooting, such as documentation completion or trade confirmation, while the latter mainly concerns the application of that automation—for example, sending out the right information to investors or clients based on historic trends.
As our US editor Anthony Malakian detailed in his March feature Machine Learning: Hype vs. Reality, the growing interest in this space has led to a related hype bubble that obscures both the current state of machine learning technologies and the potential application within the capital markets.
It may seem like a daunting task, but there is clear potential for the buy side through the application of machine learning.
In June this year, the sixth annual New York FinTech Innovation Lab Demo Day was held at Bank of America, concluding a 12-week development program as the eight participating start-ups pitched their products to a number of institutions.
Of the eight firms involved, three focused on machine learning, covering specifics including automating risk controls and certification compliance, the simplification of unstructured legal debt documents, and a hiring platform that places technologists with hiring managers using an ever-evolving algorithm.
At the time, Maria Gotsch, president and CEO of the Partnership Fund for New York, told Waters that the theme of artificial intelligence developments is “an indication of how the technology has matured to the point where it can be used within an enterprise setting by a young company.”
Big Data Bigger
There are a number of researchers and specialist vendors out there that are making huge advancements in this space; for example, in February this year researchers at the UK’s University of Southampton created a five dimensional (5D) digital data recording and retrieval process using nanostructured glass that has incredibly far-reaching implications on the way that data can be stored in the future.
While that may sound like so much science fiction it underlines the rate of progress that is being made. That element of sci-fi movie mumbo-jumbo has been a stumbling block for some, who are unable to separate fact from fiction and instead look to more traditional automation processes.
The bottom line here is that machine learning systems won’t be for everyone. Larger, institutional asset managers may find it a much harder task to either develop or integrate a piece of machine learning software as part of or into its existing infrastructure without a resulting headache, in part due to the siloed nature of its data storage capacity.
For the time being at least, it is the smaller, nimbler players that are best positioned to take advantage of the potential that machine learning development can offer. There also needs to be a degree of faith placed in technology, particularly when it comes to short-term scale applications, as decisions made based on artificial intelligence need to be seen through to the conclusion to actually reap any benefit. And then there’s the costly matter of hiring the right people to build these systems.
It may seem like a daunting task, but there is clear potential for the buy side through the application of machine learning; you just need to have a little faith and trust in the machines. Sorting out your data helps, too.
Only users who have a paid subscription or are part of a corporate subscription are able to print or copy content.
To access these options, along with all other subscription benefits, please contact info@waterstechnology.com or view our subscription options here: https://subscriptions.waterstechnology.com/subscribe
You are currently unable to print this content. Please contact info@waterstechnology.com to find out more.
You are currently unable to copy this content. Please contact info@waterstechnology.com to find out more.
Copyright Infopro Digital Limited. All rights reserved.
As outlined in our terms and conditions, https://www.infopro-digital.com/terms-and-conditions/subscriptions/ (point 2.4), printing is limited to a single copy.
If you would like to purchase additional rights please email info@waterstechnology.com
Copyright Infopro Digital Limited. All rights reserved.
You may share this content using our article tools. As outlined in our terms and conditions, https://www.infopro-digital.com/terms-and-conditions/subscriptions/ (clause 2.4), an Authorised User may only make one copy of the materials for their own personal use. You must also comply with the restrictions in clause 2.5.
If you would like to purchase additional rights please email info@waterstechnology.com
More on Trading Tech
Agentic AI comes to Bloomberg Terminal via Anthropic protocol
The data giant’s ubiquitous terminal has been slowly opening up for years, but its latest enhancement represents a forward leap in what CTO Shawn Edwards calls, “the way we should talk to the world.”
M&G Investments braves cost headwinds in pursuit of AI
The UK asset manager’s AI ambitions started with the creation of a data lake to ensure high-quality data is being fed into models.
Asic probe piles pressure on ASX to deliver Chess replacement
But market insiders think late intervention by regulators could even slow down implementation.
Stakes raised for UK bond, EU derivatives tapes after Ediphy clinches win
The pressure is on for TransFICC, Etrading, Finbourne, and Propellant Digital, who are still vying to provide the UK’s fixed income consolidated tape after Esma awarded the EU’s tape to Ediphy and its partners.
Exchange M&A, US moratorium on AI regs dashed, Citi’s “fat-finger”-killer, and more
The Waters Cooler: Euronext-Athex, SIX-Aquis, Blue Ocean-Eventus, EDM Association, and more in this week’s news roundup.
LSEG officially sunsets Eikon
The exchange operator withdrew the platform from its product lineup this week.
Cloud Wars: Are EU and APAC firms really pining for homegrown options?
Waters Wrap: In the wake of tariffs and regional instability, there’s chatter about non-US firms lessening their dependency on the major hyperscalers. Anthony is not buying it.
Bloomberg, MTS expand portfolio trading to EGBs
The platform providers will follow Tradeweb with the extension of the popular credit protocol.