Waters Wrap: Is Low-Code a Movement or a Mirage (Plus the ODRL Gambit & AI’s Afterthought Problem)
Anthony Malakian looks at the industry’s digital rights project and new tech platforms that aim to revolutionize the capital markets.
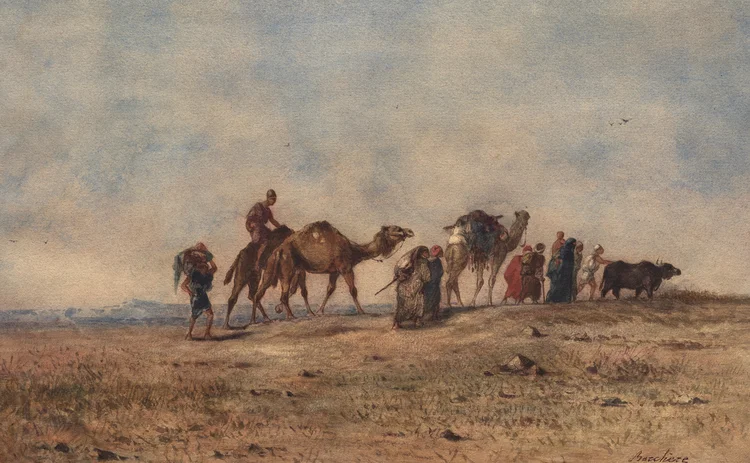
The inaugural WatersTechnology Innovation Exchange is almost over. What started on September 9 will conclude on Tuesday. All of the panels will be available on-demand through Tuesday, so if you haven’t already registered, there’s still time to sign up and check out all of the panels and presentations from the event. If anything, you can watch me expertly moderate panels on AI and on data management from my glorious flag room. Register here: https://events.waterstechnology.com/innovation-exchange/book-now
How Low Can You Go?
In 2017, according to eFinancial Careers, the average salary for a mid-level engineer at a bank was $150,000. I quickly reached out to a bank CTO to see if that number was right, and he said that generally it was, “but it goes up once you start talking about the machine-learning stuff and prop-trading stuff.” This is all to say that talented software engineers and data scientists do not come cheap.
Until the day comes that we live in either a dystopian or utopian society where the machines start coding, and building platforms on their own, we’re going to continue writing articles about how capital markets firms struggle to find top-tier technologists. But what if a certain technology itself, one that requires little technical expertise to use, could remedy that exact challenge?
As Reb Natale explains in her latest feature, this is the premise of the low-code movement that is slowly seeping into the world of finance. There’s an interesting blend of companies that have entered the space: there are startup fintech companies like Genesis and Unqork; Amazon Web Services has launched Honeycode; and even Morgan Stanley has developed an open-source product that can loosely fall into this space. By some estimates, the low-code application market will hit the $50 billion mark by 2026. And it’s certainly picking up skeptics and true believers on the way.
Reb explores the pros and cons for this evolving type of technology, but the main question is whether or not low-code applications can actually power high-performance trading platforms, or if they’re nice tools for simple, manual tasks, like workflows, surveys, and approval chains—useful in the same ways that robotic process automation (RPA) is useful.
As one source told Reb, in software engineering there are no free lunches—if you cut corners in the coding you will eventually pay for it later. To me, the near future will be about spending more—not less—on engineers and data scientists, but the benefit here is that the pool of people with programming skills is growing. Universities around the globe are churning out graduates with at least some sort of coding knowledge, and some firms are finding success in re-skilling their staff to build data analytics applications.
I just don’t see the low-code movement taking over the order and execution management space at banks and asset managers—workflow and non-proprietary, non-alpha-driving applications, sure, but when it comes to trading applications, the machines still need the humans to do the hardwiring. Think I’m wrong, please do let me know: anthony.malakian@infopro-digital.com.
What Right Do You Have?
A few months back I wrote about how Isda’s Common Domain Model (CDM) was struggling to gain bank buy-in because these institutions are having trouble making a case internally for a project that promises to produce savings on post-trade processes, but generates no revenue.
This week, Josephine Gallagher wrote a very deep examination of the Open Digital Rights Language (ODRL) initiative. ODRL is an open-sourced data model used for coding policy expressions. It was created by the World Wide Web Consortium (W3C), the international community that develops open standards aimed at making sure the web can continue to grow.
Capital markets firms including Goldman Sachs, JP Morgan, Deutsche Bank, Fidelity Investments, the Chicago Mercantile Exchange (CME), and Refinitiv have joined the W3C’s Rights Automation for Market Data Community Group. Together they are using the ODRL to develop a finance-specific digital rights language, which will later be used to build machine-readable technologies to help remove inefficiencies in data licensing and offer users more agility around their data consumption.
Just like with the CDM, the idea behind the ODRL is a good one: banks and asset managers have teams of people laboriously sifting through data licenses and interpreting usage rights—it’s a time consuming, heavily manual, and costly endeavor. If financial services firms can team up to bring some automation to this process, it will be a long-term win for the industry.
Unfortunately, there are already legal sticking points cropping up and there’s still no clear way forward for implementing the ODRL across the industry. And it’s also not clear just how much automation this will actually bring to the field of data licensing, as there will still be a need for expert market data professionals.
“Until there are tools to generate ODRL, and the language is sufficiently rich to allow the nuances of market data to be captured, then it’s a bit of a chicken-and-egg situation,” Michelle Roberts, vice president of market data strategy and compliance at JP Morgan, told Jo.
The fact that Goldman, JP, Deutsche Bank, Fidelity, and the CME—among other heavyweights—are coming to the table is a good thing. Is the ODRL the way forward? I have no idea, but even though the W3C group is hoping to introduce the first version of the digital rights language before the end of the year, the only thing that is clear is that there’s still a long road ahead for true, industry-wide ODRL acceptance.
Give it Some Thought
During a panel at the aforementioned WatersTechnology Innovation Exchange, Eric Tham, a senior lecturer at the National University of Singapore, had this to say: “We know the [machine learning] models in place are usually an afterthought, and [evaluated] largely on feature importance. Most [machine learning models] differ by how they’re obtained, the computation, the derivation, but it all goes down to the fact that they all highlight which feature is important. It still doesn’t quite explain why AI models work in finance.”
He contended that if financial services firms wanted to solve AI’s explainability barrier, firms need to “infuse” AI models with financial theory. “If you recognize that AI is about discovering relationships, then we have to go into it a bit deeper,” he said. “What are these relationships in finance? AI does this in a data-driven manner; it allows you to find patterns in finance. But to understand it deeper, you have to understand financial theory.”
Tham gets into more detail in Wei-Shen Wong’s story here, and he discusses how exactly firms can infuse that theory into machine-learning models, but it is an interesting thought. Banks tend to come off as hoary institutions. While they like to talk a big game when it comes to AI and machine learning, bank bureaucracy—and the fact that there’s strict regulatory oversight of financial services institutions—tends to scare off the top engineers and data scientists (perhaps bringing this conversation back full-circle with low-code applications). What can happen, then, is patchwork solutions are developed—or third-party tools are bolted onto an existing analytics or trading platform—but is real thought being given to the financial theory that underpins the model’s actual directive?
Sumit Kumar, head of trade execution technology and lead architect for equities, Asia Pacific at Credit Suisse, agreed with Tham’s idea that sometimes banks do approach AI as an afterthought—but that’s the case for legacy tech.
“In all honesty, that’s for the existing projects where we’re doing an enhancement; but when we start something from scratch, then the way it is approached is quite different,” Kumar said. “AI would be looked at as nothing more than glorified statistics. So effectively, the explainability part when you’re doing it from scratch is accounted for when you’re developing it. But then, the thing is that we have a huge amount of software exposure that’s running currently in production and you have to make it work together with that. That’s where the challenge comes [from].”
The fact that Cobol is still so prevalent inside of banks shows that banks can only move so fast when incorporating new technologies. But I think that what Tham is saying is that you can’t cut corners when playing catch-up in the AI arms race. Machine-learning models need to be taught and it’s imperative for humans to infuse those financial theories that exist inside the human’s brain into the model itself. If you can’t do that, then how can you truly explain the model to regulators or clients?
Only users who have a paid subscription or are part of a corporate subscription are able to print or copy content.
To access these options, along with all other subscription benefits, please contact info@waterstechnology.com or view our subscription options here: http://subscriptions.waterstechnology.com/subscribe
You are currently unable to print this content. Please contact info@waterstechnology.com to find out more.
You are currently unable to copy this content. Please contact info@waterstechnology.com to find out more.
Copyright Infopro Digital Limited. All rights reserved.
As outlined in our terms and conditions, https://www.infopro-digital.com/terms-and-conditions/subscriptions/ (point 2.4), printing is limited to a single copy.
If you would like to purchase additional rights please email info@waterstechnology.com
Copyright Infopro Digital Limited. All rights reserved.
You may share this content using our article tools. As outlined in our terms and conditions, https://www.infopro-digital.com/terms-and-conditions/subscriptions/ (clause 2.4), an Authorised User may only make one copy of the materials for their own personal use. You must also comply with the restrictions in clause 2.5.
If you would like to purchase additional rights please email info@waterstechnology.com
More on Data Management
Tech VC funding: It’s not just about the money
The IMD Wrap: It’s been a busy year for tech and data companies seeking cash to kick-start new efforts. Max details how some are putting the fun into fundraising.
BNY uses proprietary data store to connect disparate applications
Internally built ODS is the “bedrock” upon which BNY plans to become more than just a custodian bank.
Waters Wavelength Ep. 296: Questions about data quality
It’s all about the data, data, data.
The AI boom proves a boon for chief data officers
Voice of the CDO: As trading firms incorporate AI and large language models into their investment workflows, there’s a growing realization among firms that their data governance structures are riddled with holes. Enter the chief data officer.
FactSet launches conversational AI for increased productivity
FactSet is set to release a generative AI search agent across its platform in early 2025.
If M&A picks up, who’s on the auction block?
Waters Wrap: With projections that mergers and acquisitions are geared to pick back up in 2025, Anthony reads the tea leaves of 25 of this year’s deals to predict which vendors might be most valuable.
ICE Connect adds data integration capabilities for proprietary data
Intercontinental Exchange’s desktop platform is collaborating with CloudQuant to allow customers to integrate in-house data and analytics with the datasets found on its ICE Connect platform.
MIAX taps DataBP for exchange data licensing, custom contracts
To support planned growth of its data business, the exchange group has implemented DataBP’s platform to strengthen its licensing process and scale up its distribution capabilities in anticipation of end-user demand.