Artificial Intelligence, Real Intel: Data Vendors Make Strides on AI
Data companies are investing in AI and machine learning in a concerted push toward innovations that reveal insights and alpha.
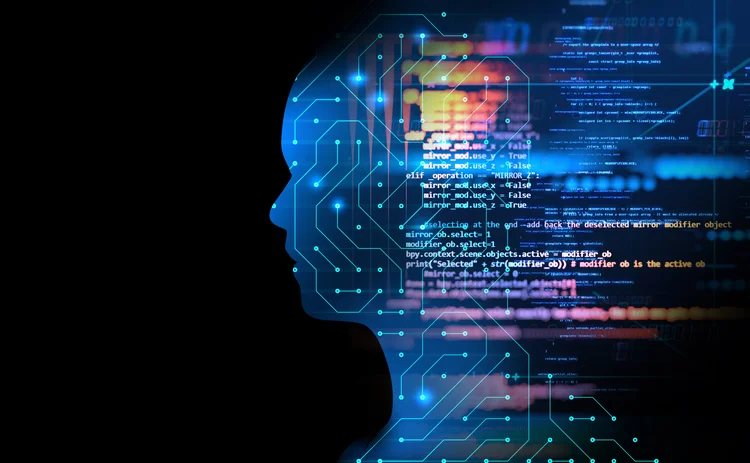
After largely lagging behind other industries to adopt artificial intelligence (AI) and machine-learning technology, the financial markets are playing catch-up with a vigor that borders on obsession. However, some of the major financial information providers that collect, manage and disseminate massive amounts of information and data have been developing and applying these technologies—though often behind the scenes—for several years.
Over the past two years in particular, there has been a
Only users who have a paid subscription or are part of a corporate subscription are able to print or copy content.
To access these options, along with all other subscription benefits, please contact info@waterstechnology.com or view our subscription options here: http://subscriptions.waterstechnology.com/subscribe
You are currently unable to print this content. Please contact info@waterstechnology.com to find out more.
You are currently unable to copy this content. Please contact info@waterstechnology.com to find out more.
Copyright Infopro Digital Limited. All rights reserved.
As outlined in our terms and conditions, https://www.infopro-digital.com/terms-and-conditions/subscriptions/ (point 2.4), printing is limited to a single copy.
If you would like to purchase additional rights please email info@waterstechnology.com
Copyright Infopro Digital Limited. All rights reserved.
You may share this content using our article tools. As outlined in our terms and conditions, https://www.infopro-digital.com/terms-and-conditions/subscriptions/ (clause 2.4), an Authorised User may only make one copy of the materials for their own personal use. You must also comply with the restrictions in clause 2.5.
If you would like to purchase additional rights please email info@waterstechnology.com
More on Data Management
New FPGA component aims to curb co-lo costs
Hardware ticker plant provider Exegy is working on a new FPGA solution that it says will free up costly processing power on firms’ existing co-lo servers.
Market data woes, new and improved partnerships, acquisitions, and more
The Waters Cooler: BNY and OpenAI hold hands, FactSet partners with Interop.io, and trading technology gets more complicated in this week’s news round-up.
Asset manager Fortlake turns to AI data mapping for derivatives reporting
The firm also intends to streamline the data it sends to its administrator and establish a centralized database with the help of Fait Solutions.
New study reveals soaring market data spend led by trading terminals
The research finds that 2024 was a record year for overall market data spend, supported by growth in terminal use, new license schemes by index providers, and great price variation among ratings agencies.
The murky future of buying or building trading technology
Waters Wrap: It’s obvious the buy-v-build debate is changing as AI gets more complex, but Anthony wonders how trading firms will keep up.
‘I recognize that tree’: Are market data fees defying gravity?
What do market data fees have in common with ‘Gilmore Girls’ and Samuel Beckett? Allow Reb to tell you.
When it comes to data inventory management, asset managers need a ‘rescue’ plan
The IMD Wrap: Inventory management may be a necessity, but it doesn’t need to be a chore. A little innovation can turn this cost center into a value generator.
How a Chinese AI firm shook the tech world
DeepSeek’s AI model is the very ethos of doing what you can with what you have.