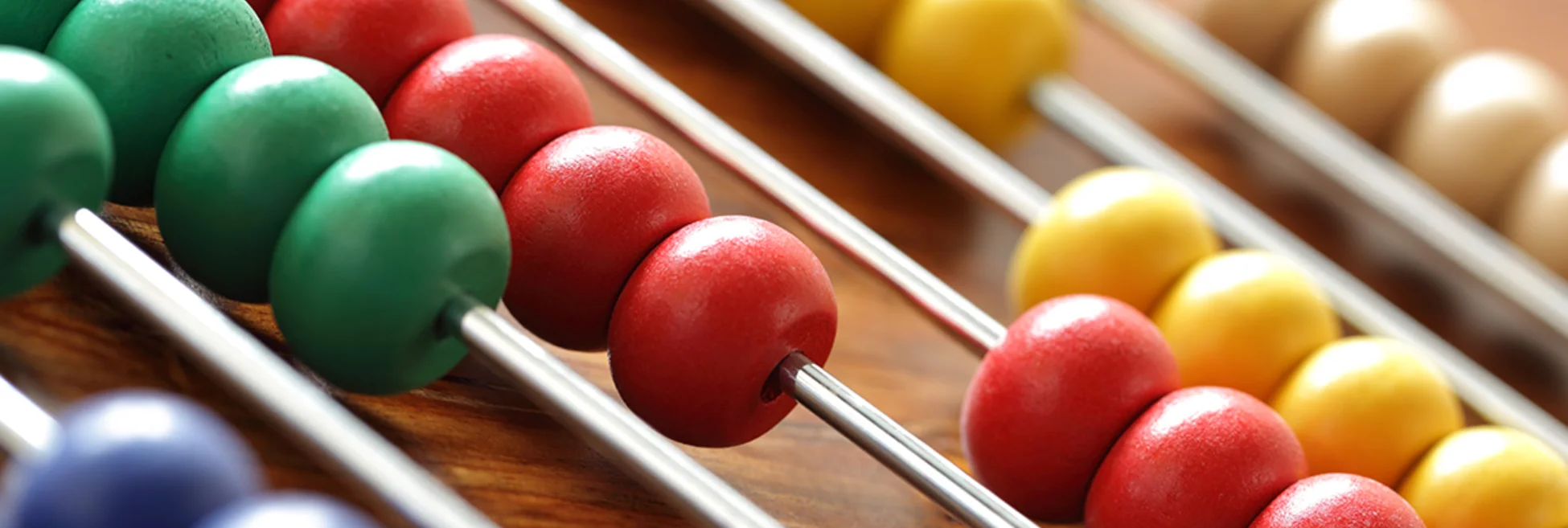
Firms Eye Machine Learning for Liquidity Risk Models
Many US mutual funds are expected to rely on vendor tools to comply with the SEC’s rule that they establish a formal liquidity risk management program that includes classifying the liquidity of their investments. These tools have the potential to improve the accuracy of firms’ models. But some see them as overly complex, and are seeking simpler approaches to compliance, reports Faye Kilburn.
However, some providers are taking advantage of recent advances in machine learning to develop these tools, and are now in the early stages of applying advanced statistical techniques to large, unstructured datasets that previously could not have been incorporated into the models.
Machine learning allows funds to account for the many factors that impact liquidity and account for very complex, non-linear relationships. Get it right, and the more sophisticated models promise value beyond the scope of just regulatory compliance.
“If you don’t have trade data to measure the liquidity of a security, everything relies on inferences to other trades. Machine learning will make the models more adaptive to new data to create those linkages, whereas a top-down approach requires periodic updates to the models’ correlations,” says a director of risk management at a US asset manager.
At this stage, the application of machine-learning techniques to modeling liquidity risk is in its infancy: There is no comprehensive list of the variables that impact the liquidity of a security, so firms are operating partly in the dark. Their ambitions are big, though.
BlackRock, for example, is building an end-to-end liquidity risk management framework that not only aims to satisfy the SEC’s liquidity rule, but will also feed information to the firm’s portfolio management, trading and risk management functions.
Stefano Pasquali, BlackRock’s head of liquidity research, says it has been known since the early 1980s that machine learning would improve liquidity risk modeling, but that until now, a lack of computational power, the scarcity of data, and limitations on storage meant that this was impossible for most quants to achieve.
The work under way now on machine learning puts mutual funds somewhat in limbo. On one hand, they might want to see if these experiments in liquidity risk modeling pay off. On the other, they are running out of time to fully test the systems they select or to secure board approval for their risk management programs.
Partly as a result, asset managers and industry groups are calling for the SEC to put the brakes on its new regulation to give firms more time to assess these new offerings.
“[As things stand] we will have to stick our neck out, and it’s going to be pretty subjective without any kind of reliable quantitative tool,” says a chief investment officer at a US mutual fund manager. “If the [vendors] are on the cusp of getting something done that would remove some of the burden from us, I would support an extension.”
The Data Problem
A central requirement of the SEC’s liquidity risk management rule is that fund managers must classify their investments into four liquidity buckets—highly liquid, moderately liquid, less liquid and illiquid—based on the number of days it takes for an investment to be converted into cash without having a material impact on the market.
The SEC initially planned to mandate nine datasets that funds would need to consider to classify the liquidity of a security, including market depth, price impact, trading volume, bids and asks, and dealers making markets. In the end, the regulator took a more flexible approach, simply requiring funds to take into account “relevant market, trading, and investment-specific considerations” when making their assessment.
Trade data would be the obvious way to assess the liquidity of a security, but its availability varies dramatically across asset classes and in over-the-counter markets. For global equities and US fixed income, the data is highly accessible; for emerging market debt and exotic structured products, for example, the data is sparse.
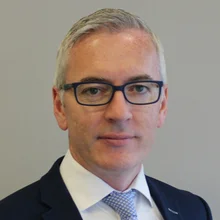
“We’re using as much data as is available in the marketplace, but there will be some asset classes where the data just doesn’t exist,” says Mark McKeon, global head of investment analytics at State Street Global Exchange.
Like BlackRock, State Street is building a fully SEC-compliant liquidity risk management framework within its truView proprietary risk analytics engine. But State Street is following a different, simpler approach to filling gaps in the data.
For example, to estimate the liquidity of bonds, State Street has implemented a “waterfall” approach that calls on different quantitative models depending on what data is available.
If a bond has not traded over the past calendar month, State Street uses an issuer model based on data for bonds with similar characteristics from the same issuer. If bonds from the issuer are seldom traded, a sector model is used to look at bonds in the same sector. When there is insufficient trading data in the sector, an asset class model is used that looks at broader characteristics of the bond such as rating and maturity—a method endorsed by the SEC in its final rules.
Intercontinental Exchange, which is also selling liquidity risk tools to asset managers, uses a similar waterfall approach for its Liquidity Indicators service, which covers more than 2.4 million global fixed-income securities and was expanded in August to include global equities.
“It’s always helpful to have more information in the form of transactional data to help validate our models,” says Rob Haddad, head of product strategy and innovation at ICE Data Services. “It is so critical to leverage observable market information and effectively model behavior to inform a viewpoint on the future potential tradability of securities across the market.”
However, with less than 2 percent of the fixed-income universe trading on any given day, firms can’t rely on there always being observable information available.
While these models are technically compliant with the SEC’s rule—and are live and in use today—there are drawbacks to the methodology: “You need to have a certain number of data points for it to work. If it trades once or twice in a few months, that’s not enough data points,” says State Street’s McKeon.
It is cases like this where scope exists to incorporate the use of machine learning. Index provider MSCI is among those looking at applying machine-learning technologies to analyze sparse, unstructured datasets, particularly in the fixed-income space—though the research is still in the embryonic stage.
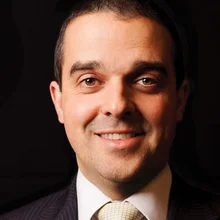
“You can have the world’s best model, but if you don’t have the data to power it, it’s going to serve no purpose,” says Carlo Acerbi, executive director for risk research at MSCI. “People who have worked on liquidity risk in equities where [they have] tick-by-tick data or closing prices every day are spoiled. In fixed income, blanks are the majority of the data, and making good use of what is not a blank is a challenging exercise. It would require infinite time for a human to crunch these numbers, so we are trying to use machine-learning techniques to parse large amounts of data and distill information.”
Bloomberg, meanwhile, has already made progress applying supervised-learning techniques to improve the liquidity score of its Liquidity Analytics service, launched in March 2016.
Of the million different securities in the municipal bond market, for example, only between 1 percent and 10 percent actually trade in institutional size, so the firm applies a neural network to extend its models for liquid muni bonds to cover illiquid ones.
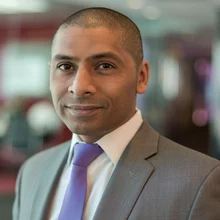
“We identify and evaluate the liquidity parameters of the 10 percent of bonds for which we have recent transactions. We then train the neural net to relate some 50 features of this 10 percent of bonds to the other 90 percent of the universe that doesn’t have a liquidity model. We start with what we know about how the 10 percent of the bonds trades and transpose this to the population of bonds for which we don’t have transaction data,” says Naz Quadri, head of liquidity analytics at Bloomberg.
The fit was so good on the model’s first run, Quadri had his team run it five more times with different variations just to be sure of the results. “Even though it is a very highly dimensional dataset, there are some underlying patterns that are really quite clear. We pulled out, in some way, shape or form, exactly the relationship muni traders have in their minds.”
Meanwhile, Bloomberg is also using unsupervised-learning techniques including anomaly detection—looking for outliers and deviations from the norm—to identify whether a trade was executed at a fair market price.
And yet, because machine learning is data-intensive, there are drawbacks also to applying these techniques.
One drawback is the cost associated with the data and technology involved. For example, sources say Bloomberg’s tool is markedly more expensive than competitors’ offerings that do not currently use machine learning. One asset manager says the Liquidity Analytics service is up to three times more expensive than rival products, and is more complex to implement.
Furthermore, non-machine-learning models, such as those of ICE and State Street, tend to have broader coverage.
“At the very top line, State Street and ICE have better coverage because they do take a little bit more of a rules-based approach when they don’t have good data. Fundamentally they’re the same building blocks of data, but the machine-learning models are just going to be more dynamic,” says a risk manager at a US asset manager.
ICE officials say the exchange uses advanced statistical techniques, but stops short of machine learning. State Street, meanwhile, says its rule-based approach is endorsed by the SEC and argues that it is more important to build a solution that gives fund managers full coverage across all asset classes.
“We will continue to test different approaches around artificial intelligence and see if it can help, but so far we haven’t been able to come to a conclusion where this would improve the model above having the actual underlying data and using that,” McKeon says.
Bloomberg’s Quadri acknowledges that machine learning needs to be done intelligently. “Otherwise, it’s akin to throwing darts at the wall,” Quadri says. However, he says the industry is overlooking that modeling liquidity risk has applications beyond regulatory compliance.
BlackRock officials say that its end-to-end liquidity risk management framework—which the firm plans to sell to other asset managers via its Aladdin platform—will also inform portfolio construction, best execution, order routing, and liquidity-adjusted risk management activities. BlackRock is also working to apply machine-learning techniques to more accurately calculate the cost of liquidating fund positions in the case of redemptions—another element of the SEC’s liquidity risk management rule.
As asset managers weigh the merits of these various approaches, the SEC’s deadline draws closer. Industry bodies the Securities Industry and Financial Markets Association (Sifma) and the Investment Company Institute (ICI) have called on the regulator to delay the rules to allow vendors to fully develop their tools. There has been no indication of a positive response so far.
The vendors, meanwhile, are less bothered about a delay. “I don’t know if I’d be happy to be told the deadline is extended. It’s pressure that makes diamonds,” MSCI’s Acerbi says.
Only users who have a paid subscription or are part of a corporate subscription are able to print or copy content.
To access these options, along with all other subscription benefits, please contact info@waterstechnology.com or view our subscription options here: http://subscriptions.waterstechnology.com/subscribe
You are currently unable to print this content. Please contact info@waterstechnology.com to find out more.
You are currently unable to copy this content. Please contact info@waterstechnology.com to find out more.
Copyright Infopro Digital Limited. All rights reserved.
As outlined in our terms and conditions, https://www.infopro-digital.com/terms-and-conditions/subscriptions/ (point 2.4), printing is limited to a single copy.
If you would like to purchase additional rights please email info@waterstechnology.com
Copyright Infopro Digital Limited. All rights reserved.
You may share this content using our article tools. As outlined in our terms and conditions, https://www.infopro-digital.com/terms-and-conditions/subscriptions/ (clause 2.4), an Authorised User may only make one copy of the materials for their own personal use. You must also comply with the restrictions in clause 2.5.
If you would like to purchase additional rights please email info@waterstechnology.com
More on Regulation
FCA: Consolidated tape for UK equities won’t happen until 2028
At an event last week, the FCA proposed a new timeline for the CT, which received pushback from participants, according to sources.
Cusip Global Services wants to know, ‘What’s your damage?’
The evidence and discovery phase of the case against the identifier bureau is set to expire in March, bringing an anticipated jury trial one step closer.
Big questions linger as DORA compliance approaches
The major EU regulation will go live tomorrow. Outstanding clarifications and confusion around the definition of an ICT service, penetration testing, subcontracting, and more remain.
Insurance: The role of risktech in effectively managing emerging risks and driving competitive edge
This whitepaper covers the global survey, conducted by Chartis Research and TCS, of banking, financial services and insurance firms, which found that insurers are struggling to adapt to evolving risks and regulatory requirement increases. Chartis offers…
FX automation key to post-T+1 success, say custodians
Custody banks saw uptick in demand for automated FX execution to tackle T+1 challenges.
Observations and lessons to learn from the move to T+1
The next few years will see other jurisdictions around the world look to North America for guidance on transitioning to shorter settlement cycles.
Expanded oversight for tech or a rollback? 2025 set to be big for regulators
From GenAI oversight to DORA and the CAT to off-channel communication, the last 12 months set the stage for larger regulatory conversations in 2025.
DORA flood pitches banks against vendors
Firms ask vendors for late addendums sometimes unrelated to resiliency, requiring renegotiation