This article was paid for by a contributing third party.
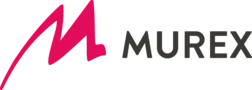
Derivatives pricing with AI: Faster, better, cheaper
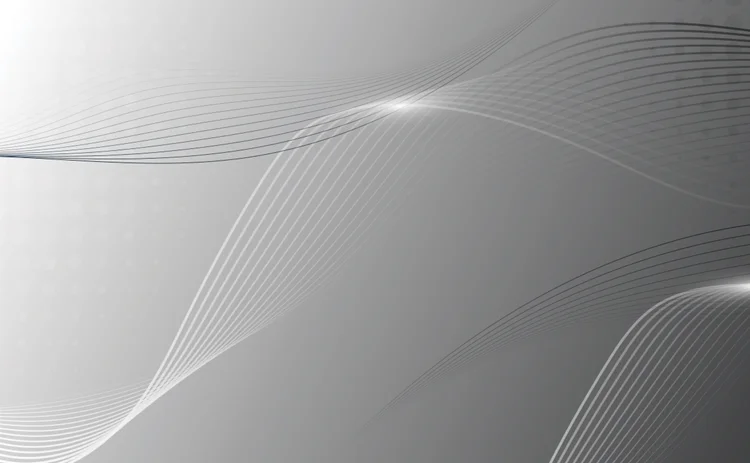
More complex models and higher calculation demands are pushing legacy hardware infrastructure for derivatives evaluation to its limits. Pascal Tremoureux, head of quantitative research at Murex, describes the firm’s mission to replicate derivatives pricing models through machine learning—slashing time and costs in the process.
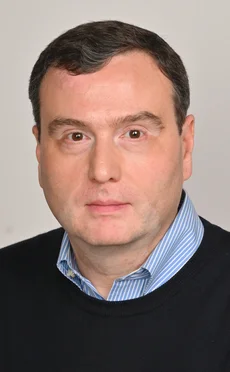
What was the purpose of Murex’s research into machine learning derivatives models?
Pascal Tremoureux: The industry faces substantial challenges directly related to increasing computational demands and hardware costs—for example, in the context of market risk, the Fundamental Review of the Trading Book, valuation adjustments—known as XVAs—and credit risk. It is now crucial to address these challenges directly, and machine learning is a critical opportunity for the industry.
Murex’s objective is to seize this opportunity. Via our platform, MX.3, we aimed to bring complex models into mainstream usage and deliver a comprehensive solution to our clients, accelerating the adoption of such technologies.
To achieve this, we needed a solution that offered a high degree of precision and robustness. These have been the key tenets of our historical approach to model development and evaluation. Leveraging machine learning for analytics also brings new, disruptive capabilities, which allow us to integrate even more complex modeling thanks to massive new speed-factor benefits. We had to address fundamental challenges to do this.
How was the project conceived and what were the key development milestones?
Pascal Tremoureux: We quickly realized a brute-force and generic approach—which we tested—was not effective. It did not produce the necessary level of accuracy. We wanted the solution to reach high levels of precision, as you would expect in a trading environment. It also had to replicate derivatives pricing models without compromising the adaptability to exotic product features, and model and market specificities.
The solution was to develop a neural network architecture based on recurrent neural networks and leverage the concept of latent space. Our whitepaper, Derivatives pricing with neural networks, published in September, describes this in more detail.
To validate the approach, we started with the Phoenix autocallable payoff. A Phoenix autocallable is a structured financial instrument with two barriers, allowing for periodic coupon payments and potential early redemption based on the performance of an underlying asset. This typically involves large combinations of features, priced with a Black-Scholes-Merton model that includes term-structure parameters, namely volatilities and rates.
We have also been looking at other asset classes, such as foreign exchange derivatives and more complex models, like stochastic local volatility.
Can you describe the training process for the neural networks, and the size and variety of datasets used?
Pascal Tremoureux: The training process is essential. We spent a lot of time and effort on this phase.
It was key for us to set the conditions for a production-grade model within an integrated solution that can be used through a light inference—whatever the market conditions and product specificities. We didn’t want a solution that required further recalibration or retraining.
We constructed the first training set by sampling a wide spectrum of volatility levels, forward market rates and very diverse payoff features, as well as a wide range of feature specificities.
We then used our financial and quantitative expertise to make further refinements. We focused on zones where model specificities play an important role and can be highlighted by market parameters.
The result was a very large training set with several billion data points, covering the largest possible set of market data, product definitions and specificities.
What were the most surprising findings from the validation and testing of the neural network models on autocallable products?
Pascal Tremoureux: First, we were very happy with our results in computation speed. These results are documented in the aforementioned whitepaper.
Second, thanks to the large number of points considered in the training set and the neural network regression capabilities, we found we obtained very good results during the learning phase with a very reasonable number of Monte Carlo simulations. This was a key outcome to accelerate this phase.
Finally, and interestingly, when diving deep to analyze outliers, we observed that the neural network actually enhances the “smoothness” of prices and sensitivities profiles, reducing the numerical noise of traditional Monte Carlo models. In fact, thanks to the activation functions implemented in the neural network, we have enabled the computation of trustable sensitivities, even by finite differences.
What are the key challenges and associated risks involved with projects like this? How did you tackle them?
Pascal Tremoureux: To begin with, it is essential to choose the appropriate strategy for neural network design. It might be appealing to opt for a “generic” neural network that takes product characteristics, calibrated model parameters and market data as inputs to generate an approximate price as the output. However, this approach often falls short on reliable results delivery. Instead, our approach considers all market data conditions, payoffs and possible variations to implement an adaptive neural network.
You must then define a proper learning set. Because of the fit-for-purpose approach, and due to our constraint—not having to retrain the model after delivering it to the client—we must consider a significant universe of payoff characteristics and market data scenarios.
Also, from day one, it is important to account for model validation requirements. Having a modular and explainable solution will make the work of model validation teams and regulatory approval easier.
Finally, we are integrating these analytics into MX.3. Effective performance depends on the analytics library with artificial intelligence (AI). But it also depends on the trading or risk system’s ability to integrate them efficiently in the context of a large volume of transactions and scenarios, without introducing integration overhead.
What burden does machine learning usage impose on hardware resources?
Pascal Tremoureux: Our solution enables clients to leverage computationally intensive models without the need for expensive hardware. Training these models requires substantial processing power, and Murex takes on the responsibility of performing this task on its infrastructure.
Once trained, the models are deployed and made available to many clients where online execution, limited to inference, is extremely fast, with finite hardware needs on the client side. This approach allows clients to achieve significant economies of scale.
What were your key takeaways from this project? What’s next for Murex’s research in this area?
Pascal Tremoureux: We are very pleased with the results achieved in terms of accuracy and speed. Additionally, developing this knowledge and expertise within our quant team has been instrumental. Consequently, we are leveraging these results to extend their scope of application. Murex continues to explore other machine learning opportunities.
We strongly believe that, to obtain production-grade results that can be model-validated, you cannot simply leverage generic AI skills. Our analytics experience was crucial in obtaining these outcomes.
Download the Murex white paper:
Derivatives pricing with neural networks: time stepping through the latent space
Sponsored content
Copyright Infopro Digital Limited. All rights reserved.
As outlined in our terms and conditions, https://www.infopro-digital.com/terms-and-conditions/subscriptions/ (point 2.4), printing is limited to a single copy.
If you would like to purchase additional rights please email info@waterstechnology.com
Copyright Infopro Digital Limited. All rights reserved.
You may share this content using our article tools. As outlined in our terms and conditions, https://www.infopro-digital.com/terms-and-conditions/subscriptions/ (clause 2.4), an Authorised User may only make one copy of the materials for their own personal use. You must also comply with the restrictions in clause 2.5.
If you would like to purchase additional rights please email info@waterstechnology.com