Max Bowie: The Next ‘Big’ Thing: Big Service
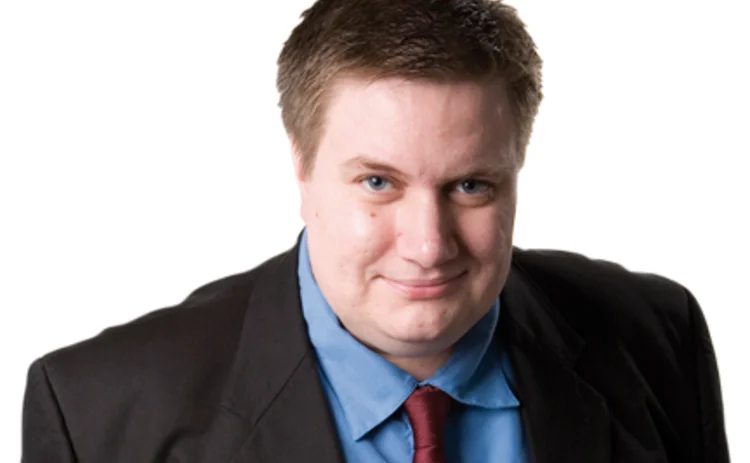
In Setting the Table, Danny Meyer, owner of Union Square Café in New York, describes an early example of big data in action, and its evolution. He outlines his father’s business running tours of Europe, and how he gained a vast knowledge of people and places, food and drink to satisfy the most discerning tourist’s palette, and built a mental cross-referencing database of flavors to pair the most complex and diverse plates and wines, all of which formed the basis for creating one of the city’s most consistently highly-rated restaurants.
But, just as it takes more than a premise and a price feed to make profitable trades, it took more than that to make and keep the restaurant successful. For example, as a trading strategy in the financial markets evolves by taking note of changing market conditions, Meyer’s staff is constantly collecting and recording data about customers and their preferences through feedback cards accompanying the check, by engaging customers in conversation when they make a reservation, or from their interactions with the wait staff. By knowing whether a diner is a new customer or a regular, what table they prefer, their taste in wine, and what kind of experience they had on previous visits, the restaurant can tailor its service to provide the best experience for each—and make them a more loyal customer and maximize that relationship.
Though big data is a recent catchphrase in financial markets for collecting, processing and analyzing enormous quantities of information that are not officially “market data,” but may nevertheless impact prices and markets, the concept of incorporating a wide variety of different yet impactful datasets isn’t new. Even before the latest generation of news and social media sentiment analysis, a class of traders grew up trading on news and their prediction of how the market will react. Before electronic news feeds, speculators and news barons used the telegraph, carrier pigeons and semaphore towers to communicate quickly across distances so they could take advantage of market-moving news in markets relying on slower methods of communication.
Besides news about commodities or companies, traders realized that macroeconomic and geopolitical events also impact markets in general and specific sub-sets of securities or derivatives. This became so competitive that in the US, announcements of market-moving government figures and reports are strictly controlled, governing the precise time that reporters can transmit stories from so-called “lockup” rooms.
Then over time, the type of news that could have an impactful effect on a company’s price expanded, and hence so did the sources from which traders needed to capture data. The impact of weather on crop yields made weather data invaluable to commodities traders. A gaffe by a CEO in the society pages—or nowadays, a careless blog post or tweet—could spell disaster for a company’s stock price. And so, not only did traders and aggregators need to capture and process unstructured data like new stories, but they also began to trawl the web for sources that others either hadn’t yet discovered or hadn’t learned how to decipher and understand meaningfully.
Even before the latest generation of news and social media sentiment analysis, a class of traders grew up trading on news and their prediction of how the market will react.
Indicators
Other new data sources include analysis of public sentiment expressed via Twitter as a leading indicator of price movement, and behavioral analysis of historical investor activity in response to price movements, such as that provided by vendors such as now-defunct Titan Trading Analytics—itself a lesson that technical innovation must always be done with customer service in mind.
Capturing, formatting, storing and retrieving—then actually analyzing—this data has created a new cottage industry of high-performance database tools, while others still argue about the merits of big data architectures, and whether they can deliver the results they promise for traders. After all, firms may ultimately get the most value not from trying to apply big data to split-second deals, but using it in the way originally intended—to understand that split-second activity and for whom existing inputs are most valuable—and ultimately providing better customer service so that clients don’t turn to a broker or venue because it’s fastest or cheapest, but because it’s the best.
Only users who have a paid subscription or are part of a corporate subscription are able to print or copy content.
To access these options, along with all other subscription benefits, please contact info@waterstechnology.com or view our subscription options here: http://subscriptions.waterstechnology.com/subscribe
You are currently unable to print this content. Please contact info@waterstechnology.com to find out more.
You are currently unable to copy this content. Please contact info@waterstechnology.com to find out more.
Copyright Infopro Digital Limited. All rights reserved.
As outlined in our terms and conditions, https://www.infopro-digital.com/terms-and-conditions/subscriptions/ (point 2.4), printing is limited to a single copy.
If you would like to purchase additional rights please email info@waterstechnology.com
Copyright Infopro Digital Limited. All rights reserved.
You may share this content using our article tools. As outlined in our terms and conditions, https://www.infopro-digital.com/terms-and-conditions/subscriptions/ (clause 2.4), an Authorised User may only make one copy of the materials for their own personal use. You must also comply with the restrictions in clause 2.5.
If you would like to purchase additional rights please email info@waterstechnology.com
More on Emerging Technologies
Project Condor: Inside the data exercise expanding Man Group’s universe
Voice of the CTO: The investment management firm is strategically restructuring its data and trading architecture.
BNP Paribas explores GenAI for securities services business
The bank recently released a new web app for its client portal to modernize its tech stack.
Bank of America and AI, exchanges feud with researchers, a potential EU tax on US tech, and more
The Waters Cooler: Broadridge settles repos in real time, Market Structure Partners strikes back at European exchanges, and a scandal unfolds in Boston in this week’s news roundup.
Bloomberg rolls out GenAI-powered Document Insights
The data giant’s newest generative AI tool allows analysts to query documents using a natural-language interface.
Tape bids, algorithmic trading, tariffs fallout and more
The Waters Cooler: Bloomberg integrates events data, SimCorp and TSImagine help out asset managers, and Big xyt makes good on its consolidated tape bid in this week’s news roundup.
DeepSeek success spurs banks to consider do-it-yourself AI
Chinese LLM resets price tag for in-house systems—and could also nudge banks towards open-source models.
Standard Chartered goes from spectator to player in digital asset game
The bank’s digital assets custody offering is underpinned by an open API and modular infrastructure, allowing it to potentially add a secondary back-end system provider.
Saugata Saha pilots S&P’s way through data interoperability, AI
Saha, who was named president of S&P Global Market Intelligence last year, details how the company is looking at enterprise data and the success of its early investments in AI.