Michael Shashoua: Figures, Guts and Glory
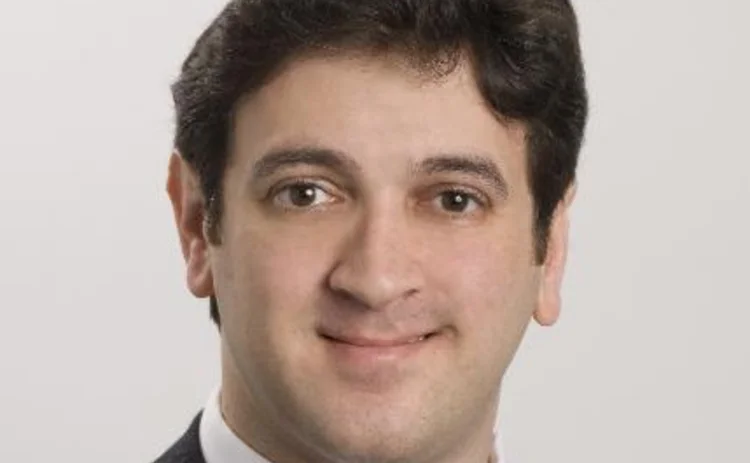
Taking part in my second fantasy football draft last month for the popular interactive competition based on drafting virtual teams of real players from the US National Football League and then tallying scores based on players’ stats during the season, it occurred to me that the process of the fantasy draft has many similarities to securities trading and applications of data and information supporting trading and risk management processes.
In my league’s draft, participants got one minute to make a pick when their turn came around. This resembles the high-pressure, snap-decision trading environment. Between picks, as references, I had my own previously created watchlist of players of interest; a live updated feed of players still available to choose from, ranked by perceived value, including an average of when they were picked in similar drafts; and my own qualitative information added to this mix—a New York Times fantasy football evaluation that had tweet-length comments on individual players, skewing positively or negatively with few middle-of-the-road or non-committal comments.
Key Data
The live feed included a key piece of data—a number for the average position at which that player had been picked in previously held drafts on the system administering our league. So, for example, in a much later round I had the 139th overall pick. Consulting quickly with the live feed, I chose Baltimore Ravens running back Bernard Pierce, who was on average chosen 122nd, but was still available in our draft. At that late stage, not knowing some of the more obscure players, that piece of data was a good reason for this choice—hoping I was getting a “steal” of some sort.
This is akin to reading data and concluding that a security is, in effect, undervalued, and worth buying. However, you cannot discount qualitative analysis. Again, for example, at pick number 42, I was looking for a wide receiver for my roster, and had two choices still available who were close in rank—Roddy White and Larry Fitzgerald. On average, Fitzgerald had been drafted earlier than White had, but as I checked that New York Times analysis, I saw these comments:
• On White: “Top 10 WR once fully healed in ’13, poor defense will force tons of air time in ’14.”
The object lesson is that risk management invariably includes more than binary choices dictated by data.
• On Fitzgerald: “31 and likely final year with the Cards. Hasn’t cracked 1,000 + yards since 2011.”
I picked White. This was like researching a company and finding some piece of information about the product it is developing or the management culture that isn’t necessarily evident in the stock price on a given day. You may say this requires some instinct and gut feeling as well—because one’s interpretation of the facts can be subjective.
And in the securities reference data world, the object lesson is that risk management invariably includes more than binary choices dictated by data. Experience, expertise and market knowledge should—or ought to—play a part.
Late Pick
To illustrate this, let’s revisit the late pick of Bernard Pierce. I had also put him on my watchlist because his teammate Ray Rice was suspended for the first two weeks of the season for off-field issues. So Pierce may have gotten more yards as a result, and thus appeared to have more value than his average draft position showed. Seeing him still available at that late round, I chose him above others that may have seemed like smarter picks if you only looked at raw data.
As you read this, the US football season will already be under way and all these choices may have proved disappointing for unforeseen reasons, but the decision-making processes that led to them, through a combination of hard numbers with qualitative evaluation and knowledge, show how to produce a more useful form of reference data.
Only users who have a paid subscription or are part of a corporate subscription are able to print or copy content.
To access these options, along with all other subscription benefits, please contact info@waterstechnology.com or view our subscription options here: http://subscriptions.waterstechnology.com/subscribe
You are currently unable to print this content. Please contact info@waterstechnology.com to find out more.
You are currently unable to copy this content. Please contact info@waterstechnology.com to find out more.
Copyright Infopro Digital Limited. All rights reserved.
As outlined in our terms and conditions, https://www.infopro-digital.com/terms-and-conditions/subscriptions/ (point 2.4), printing is limited to a single copy.
If you would like to purchase additional rights please email info@waterstechnology.com
Copyright Infopro Digital Limited. All rights reserved.
You may share this content using our article tools. As outlined in our terms and conditions, https://www.infopro-digital.com/terms-and-conditions/subscriptions/ (clause 2.4), an Authorised User may only make one copy of the materials for their own personal use. You must also comply with the restrictions in clause 2.5.
If you would like to purchase additional rights please email info@waterstechnology.com
More on Emerging Technologies
On GenAI, Citi moves from firm-wide ban to internal roll-out
The bank adopted three specific inward-facing use cases with a unified framework behind them.
How a Chinese AI firm shook the tech world
DeepSeek’s AI model is the very ethos of doing what you can with what you have.
To unlock $40T private markets, Hamilton Lane embraced automation
In search of greater transparency and higher quality data, asset managers are taking a tech-first approach to resource gathering in an area that has major data problems.
Waters Wavelength Ep. 304: Nice Actimize’s Lee Garf
Lee joins to discuss changes in communication platforms over the last few years.
DTCC revamps data distribution, collection efforts with cloud, AI
The US clearinghouse is evaluating the possibilities that cloud and AI offer to streamline the processes by which it collects and makes data available to market participants.
Agentic AI and big questions for the technologists
Waters Wrap: Much the same way that GenAI dominated tech discussions over the last two years, the road ahead will feature a lot of agentic AI talk—and CIOs and CTOs better be prepared.
Waters Wavelength Ep. 302: Connectifi’s Nick Kolba
Nick joins the show to give his views on trends in the interoperability space and the FDC3 standard.
AI co-pilot offers real-time portfolio rebalancing
WealthRyse’s platform melds graph theory, neural networks and quantum tech to help asset managers construct and rebalance portfolios more efficiently and at scale.