Golden Copy: Learning to Master the Machines
More machine learning efforts are arriving for financial data management, but are they well guided?
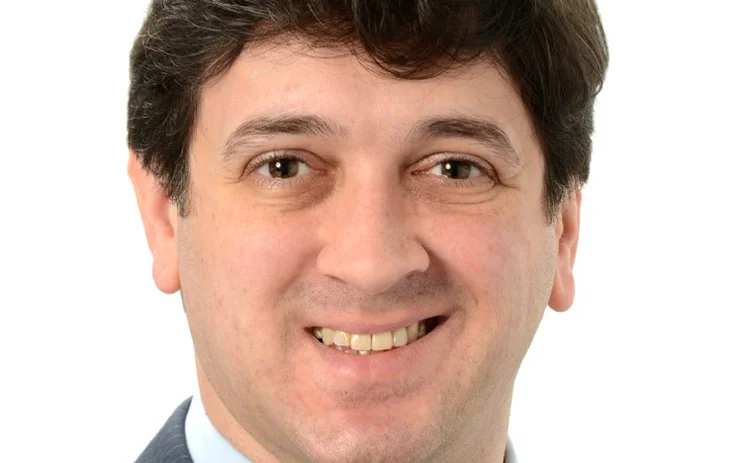
This past week saw two new machine learning efforts for financial data management – Bloomberg's Liquidity Assessment Tool, just launched, and StockViews, a crowdsourcing investment platform that reaped new funding for applying machine learning and artificial intelligence to enhance its crowdsourced research on companies.
We have also seen other machine learning initiatives for financial data in recent weeks and months. In late February, Velocimetrics, a performance measurement and analytics provider, announced that it had added machine learning techniques to its market data quality solution.
Last year, WorkFusion executive Adam Devine shared how the company was applying artificial intelligence to the automation of repetitive data processing tasks. And IIROC, Canada's major self-regulatory organization, has completed a machine learning project to segment market participants.
Also last year, in this column, I identified AltX, Dataminr and Verafin as companies that are making use of machine learning in different ways to yield greater insights from data, whether for portfolio managers or for compliance purposes.
These add up to quite a few machine learning ventures, and could be just the tip of the iceberg within the financial industry. The question that must be asked is whether the hands guiding any or all of these efforts are using machine learning processes effectively to gain more useful insights from data in order to produce intelligence that is indeed actionable.
Often, the rationale for using machine learning is indeed automation of data processing, as WorkFusion does. Automating data processing produces efficiency, but doing so with artificial intelligence or machine learning is the key factor in raising data quality, or at least avoiding the decline in data quality that would inevitably occur in automation without an intelligence factor to reduce errors.
Since last year, judging by the emergence of these recent new ventures, confidence in machine learning and artificial intelligence seems to be continuing its rise. Yet even the efforts begun less recently must still build a track record of effectiveness and value for their users.
Only users who have a paid subscription or are part of a corporate subscription are able to print or copy content.
To access these options, along with all other subscription benefits, please contact info@waterstechnology.com or view our subscription options here: http://subscriptions.waterstechnology.com/subscribe
You are currently unable to print this content. Please contact info@waterstechnology.com to find out more.
You are currently unable to copy this content. Please contact info@waterstechnology.com to find out more.
Copyright Infopro Digital Limited. All rights reserved.
As outlined in our terms and conditions, https://www.infopro-digital.com/terms-and-conditions/subscriptions/ (point 2.4), printing is limited to a single copy.
If you would like to purchase additional rights please email info@waterstechnology.com
Copyright Infopro Digital Limited. All rights reserved.
You may share this content using our article tools. As outlined in our terms and conditions, https://www.infopro-digital.com/terms-and-conditions/subscriptions/ (clause 2.4), an Authorised User may only make one copy of the materials for their own personal use. You must also comply with the restrictions in clause 2.5.
If you would like to purchase additional rights please email info@waterstechnology.com
More on Data Management
Asset manager Saratoga uses AI to accelerate Ridgeline rollout
The tech provider’s AI assistant helps clients summarize research, client interactions, report generation, as well as interact with the Ridgeline platform.
CDOs evolve from traffic cops to purveyors of rocket fuel
As firms start to recognize the inherent value of data, will CDOs—those who safeguard and control access to data—finally get the recognition they deserve?
It’s just semantics: The web standard that could replace the identifiers you love to hate
Data ontologists say that the IRI, a cousin of the humble URL, could put the various wars over identity resolution to bed—for good.
The art of communication: Data pros need better messaging
As the CDO of a tier-one bank puts it, when there’s an imbalance in communication between the data organization and the business (much less other technology heads) “that creates problems.”
Does TP Icap-AWS deal signal the next stage in financial cloud migration?
The IMD Wrap: Amazon’s deal with TP Icap could have been a simple renewal. Instead, it’s the stepping stone towards cloudifying other marketplace operators—and their clients.
T. Rowe Price’s Tasitsiomi on the pitfalls of data and the allures of AI
The asset manager’s head of AI and investments data science gets candid on the hype around generative AI and data transparency.
Waters Wavelength Ep. 298: GenAI in market data, and everything reference data
Reb is back on the podcast to discuss licensing sticking points for market and reference data.
Back to basics: Taxonomies, lineage still stifle data efforts
Voice of the CDO: While data professionals are increasingly showing their value when it comes to analytics and AI adoption, their main job is still—crucially—getting a strong data foundation in place. That starts with taxonomies and lineage.