This article was paid for by a contributing third party.

AI Adoption Across Capital Markets

Executive Summary
“Every aspect of learning or any other feature of intelligence can be so precisely described that a machine can be made to simulate it.”
Conceived at a conference at Dartmouth University in 1956, the concept of artificial intelligence (AI) began with this simple, incredibly bold argument. Henry Simon, one of the conference attendees, went further in 1965, predicting that machines will “be capable, within 20 years, of doing any work a man can do.” Such pronouncements were commonplace; early adherents of AI did not lack ambition.
AI’s very nature begs important questions, from capability to deployment. Take these two examples: is it even possible to accurately crystallize every learned activity? Are perfect simulacra really the objective of AI or should these tools be able to do whatever humans can do, but differently? Beyond that, AI opens up an entirely new realm of moral, ethical and even existential dilemmas to consider. AI’s momentum has ebbed and flowed, with the scientific community buckling under the weight of that very human element: great expectations. Even after beating chess champion Garry Kasparov, bettering Jeopardy contestants and creating robots capable of doing box jumps and backflips, AI as predicted by Simon is far from being a reality.
AI technologies have nonetheless come a long way. If the issues once seemed better suited to engineering laboratories and philosophy theses than to boardrooms, that is certainly not the case today. The concept is firmly in the popular consciousness. Across many industries and within the public sector, AI applications are now seen as change agents and cost reducers, with IBM’s Watson one of the most ubiquitous characters in advertising. For many companies, few investments are made into new processes today without at least contemplating whether AI can assist them. Yet the same, previously mentioned dilemmas manifest themselves in business contexts too. What are the risks associated with delegating responsibility for a person’s tasks to a machine? Can those risks be managed? How vulnerable is the broader business model to AI-driven disruption?
These questions are particularly acute for financial services, which is catching up to the promise of AI after a slow, skeptical start. In some ways, these considerations are similar to those in the adoption of any new technology: AI must be integrated into the stack thoughtfully, and special attention must be paid to pairing it with—or replacing—existing analytics. New compliance must be built around it, and proving the effectiveness of its application and metricizing its performance—effectively, by creating reliable data—must be undertaken.
But, in other ways, adopting AI is more of a greenfield exercise. AI today represents an umbrella for a number of subset technology groups, and depending on the nature of the task and their internal commitment to the technology, firms must choose which one is suitable. Most financial technologies are also viewed as laissez-faire and plug-and-play; by contrast, some in the industry see ample reason to regulate AI. With that in mind, how can a mutually benefitial outcome
be achieved?
Survey research conducted by WatersTechnology in April 2018 engaged respondents at investment banks, asset managers, exchanges, regulators and technology firms to gain a clearer sense of progress in AI’s capital markets adoption. The results reflect an interesting moment for the technology. It is clear that AI is well defined and firmly on the radar, in particular as it relates to specific core functions. Outside of these, however, many admit they are searching for the right application of AI—they like the square peg, but for the moment still have not managed to adapt to the round hole. This whitepaper takes a look inside this emerging, if qualified, optimism.
Note that percentages in some graphs may not equal 100% due to rounding.
The AI Framework
AI remains an unproven and newfangled concept to many in financial services. The bar to value-added AI in institutional finance is higher than in some other client‑facing industries where, for example, chatbots can replace human service and making significant savings for the enterprise.
The computational complexity of risk and portfolio management, and the increasingly algorithmic nature of trade execution mean AI is already competing with proprietary infrastructure running sophisticated software, often tailored to the business. These technologies may seem able to perform tasks well beyond the capabilities of typical human intelligence—and are certainly able to perform them more efficiently, and so AI will be tasked with emulating more advanced tasks and behaviors and in a more closely observed way within the financial context than elsewhere.
According to the survey, this has created a variety of levels of familiarity with the technology across financial firms.
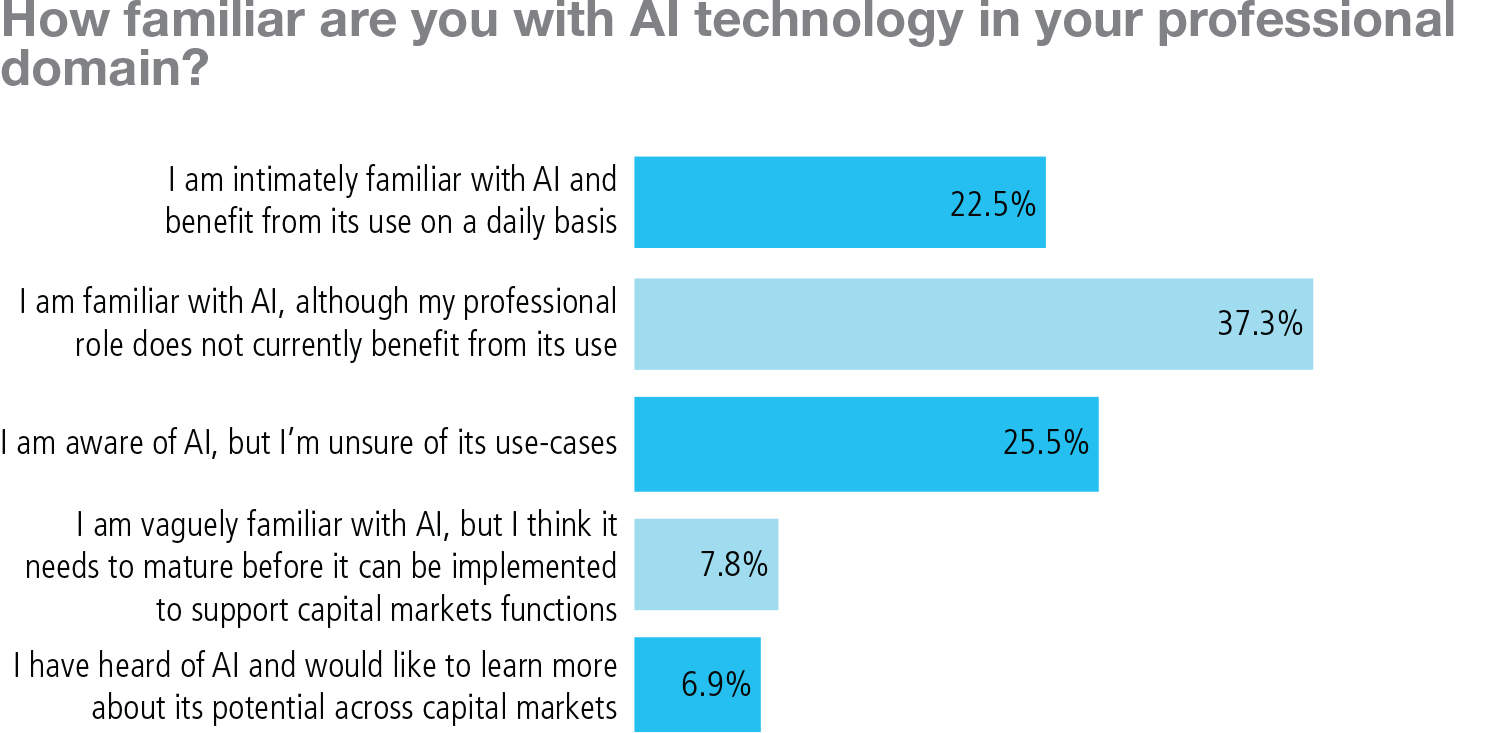
AI adoption faces an initial constraint, which is the foundational progress and limitations of AI itself. Attendees of the Dartmouth University conference more than 60 years ago imagined a version of ‘strong’ AI that could attain the cognitive strength of the human brain and ultimately become self-aware. No effort has come close to that outcome. Therefore, more recent developments have focused on versions of ‘lesser’ AI that can meet some of the foundational criteria required to move this advanced vision of AI closer.
These steps—which in most cases have taken years or often decades to achieve—include the ability to interact, predict or adapt to evolving inputs, and to analyze and move within physical space. From those conceptual goals, AI technology subgroups have grown naturally: natural‑language processing and robotics processing automation (RPA), including chatbots and virtual assistants; machine learning, neural networks and deep learning, which use data representations rather than defined tasks to process information; and robotics that are increasingly humanoid in their mobility.
Aside from Boston Dynamics’ creation Handle and other similarly incredible robots, real-life applications of AI are determined by characteristics of applicable AI subsets—and the growing subsets within those subsets. Navigating these various options requires familiarity and a level of institutional support for moving traditional processes—however slow, clunky or expensive they may be—into a future state that is more ‘bleeding edge’. Even the more obvious AI applications have required time to marinate and mature over recent years, to the point they can be acknowledged as enterprise‑grade and distinct. That, according to survey respondents, has resulted in a gradient in the levels of readiness for the implementation of AI at financial firms, with one-third currently employing it in live environments, more than half either trialling the technology or discussing the possibility of introducing it and a small minority with no intention of implementing it.
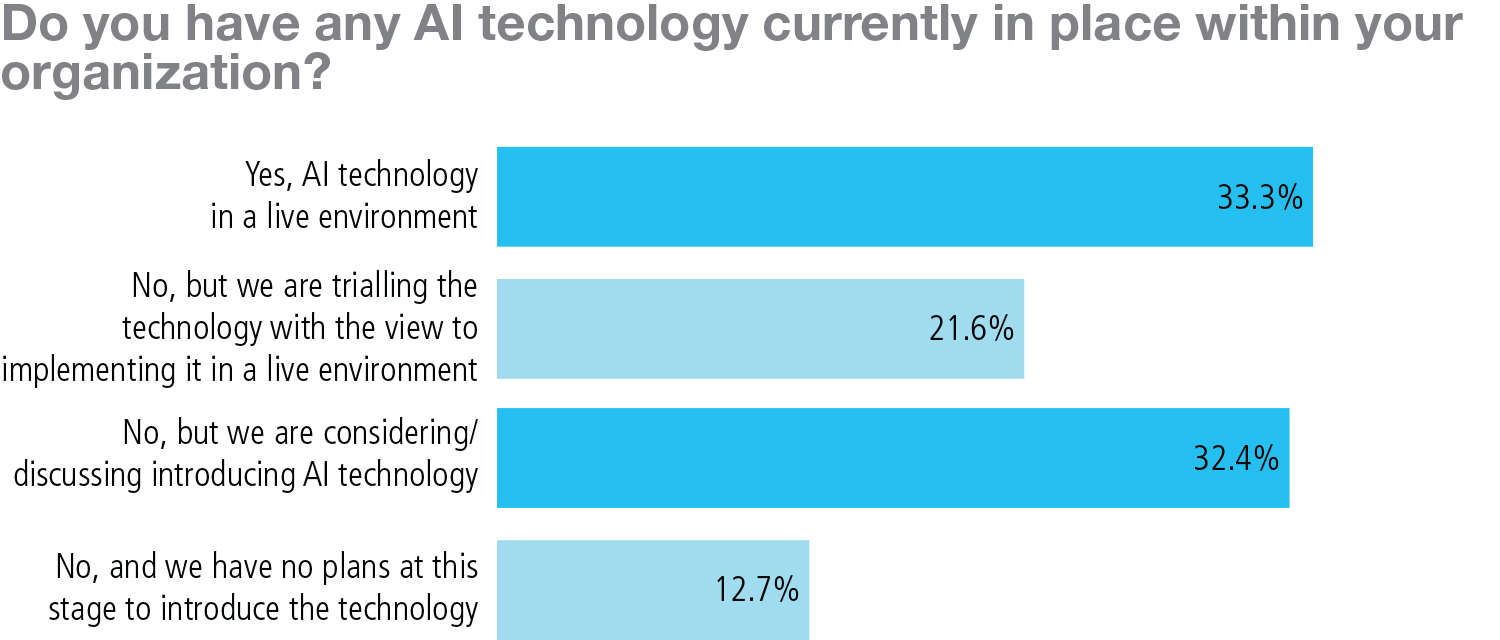
If nothing else, AI platforms are an ongoing source of fascination as banks, investment managers and the financial technology—known as ‘fintech’—community experiment with them in innovation laboratory settings. As the survey findings illustrate, the first steps toward implementation are under way for many. But then comes the hard part: beyond toying around and serious testing, AI frequently requires integration work that demands its own attention, time and investment. For all of their potential, these tools can be a heavy lift. Given all of that effort, the question remains: to what end is this endeavor?
Applications—The Stuff of Dreams
AI’s historical development is one part of the picture. Firms’ own experiences add another contour, and this too can vary significantly, according to the survey results. Mainstay sell-side institutions such as Citibank and Bank of America Merrill Lynch were speaking publicly about AI as early as 1991—introducing new projects that were once “dismissed as the stuff of dreams,” as WatersTechnology then described them. Today, four out of five respondents say that AI will be used within their domain within the next four years if it is not already in use, of which two out of five say it is being used already.
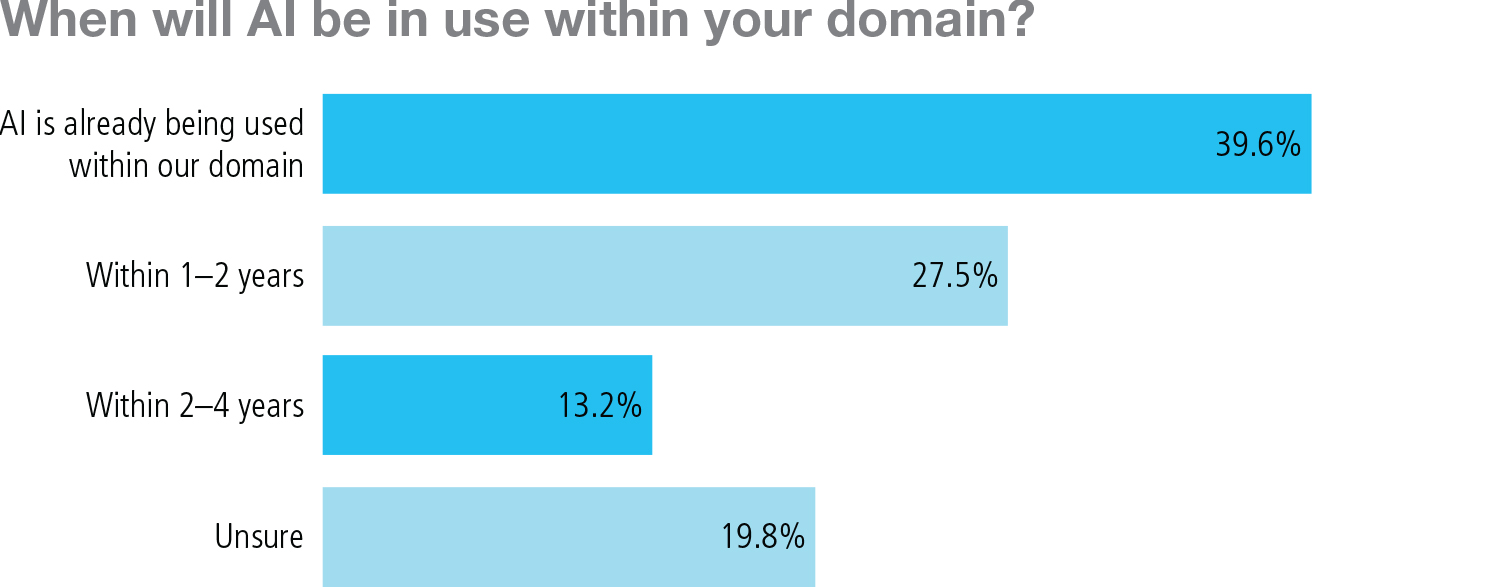
Indeed, those dreams have expanded widely around Wall Street. From front office to back, massive investment managers to hedge funds and proprietary trading shops, and across many of the services provided by the industry’s custodians, fund administrators and utilities, AI is firmly in play. Meanwhile, every vendor looking for a marketing advantage will attach the AI brand to a new product or upgraded solution. Whether these prove successful—or contain a genuine AI component at all—is a different story, and this is where the specifics matter. The distinguishing factor ought to be whether the technology can complete an action, as opposed to simply showing the user what they wish to know. As survey respondents asserted, the nature of the action can vary—although, in terms of perceived added value, automation still reigns above all.
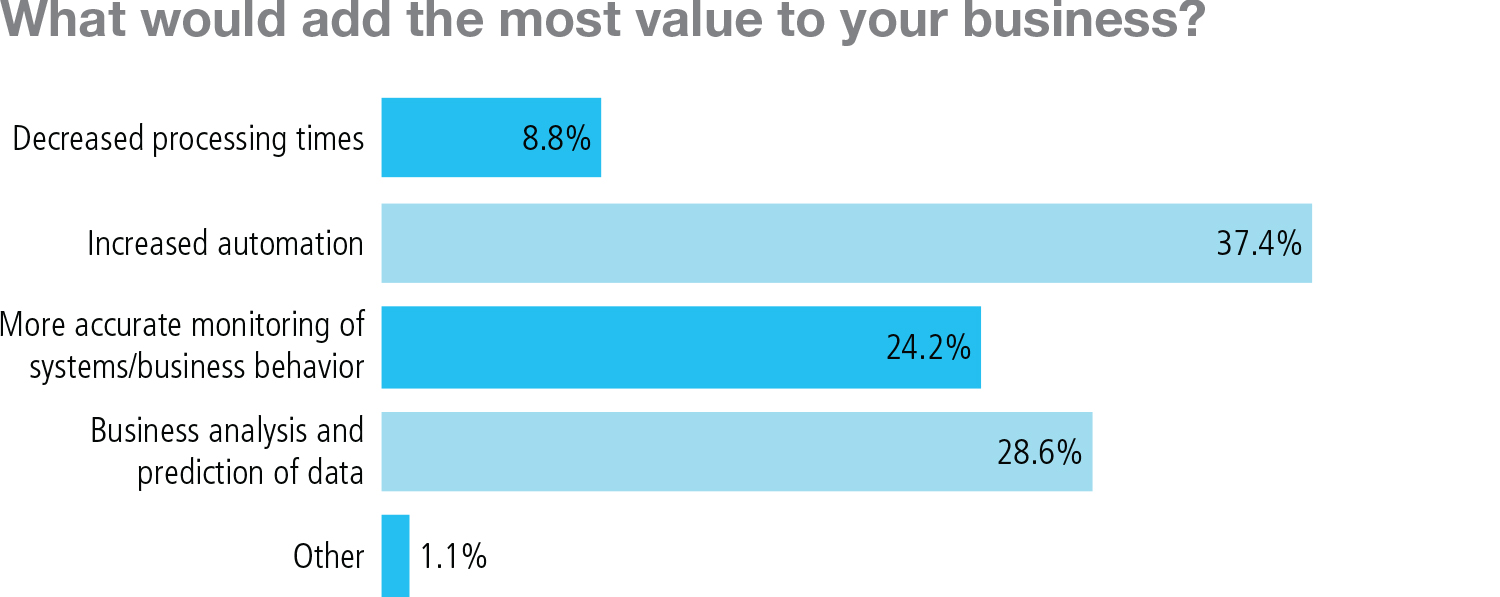
Gray areas remain. Take, for example, a predictive analytics engine that culls Twitter feeds to inform a global macro or event-driven strategy. Of course, the engine is doing something no human could—or would want to—spend their day doing. But, given the relative inaccuracy of such engines — there are very few ‘real’ and actualized Arab Springs, for example— it is highly unlikely and possibly perilous for a firm to run a completely automated trade-off of them without some kind of human intervention and oversight. At that point, is it really AI? Or just a novel way of aggregating information? The matter is ripe for debate.
Likewise, firms have discovered that AI needn’t be playing an advanced or crucial enterprise role to still be undertaking valuable human-like work. Three established and emerging areas in which financial service AI is most frequently being adopted include:
- RPA (bots). RPA deploys software bots to handle static, typically simple data management-related tasks. Particularly useful to the institutional buy side and large investment banks, RPA has demonstrated its potential to handle a wide range of operational functions: trade processing and support, reconciliations and case management, various aspects of fund administration, and client portfolio rebalancing, among others. It is a kind of bedrock AI that can supply enormous benefits of scale across the trading and data management life cycles.
- Statistical machine learning. Also known as classical machine learning, this is probably the most diverse and best-known set of applications, and moves closer to the front-office and pre-trade functions. Spanning from know-your-customer and fraud detection checks to cyber defenses, machine learning can shore things up operationally. But it is more about enabling trading desks and business units to glean information faster and ultimately increase margins. This can be achieved through tactical trading signal discovery—as in the previously mentioned predictive analytics example—or expanded on a strategic level via algorithms that can analyze millions of client data points across portfolio management, robo‑advisory, risk, underwriting and counterparty credit analysis. Because of these stakes, firms have tended to keep these implementations closer to their vests, but one obvious accomplishment stands out: much of the passive investment revolution that has swept the industry in recent years was managed by these AIs.
- Deep learning (neural networks). At the other end is a still-emerging branch of machine learning that is focused more on data science and a reconceptualization of finance at the modeling and engineering levels than on the wholesale or operational levels. Most often in use at quantitative hedge funds and proprietary trading firms, deep-learning AI combines advances in dataset nuances—in semantic analysis and geospatial imagery recognition, to name two—and neural network infrastructures to complete extremely complex and esoteric exercises for predictive pricing and portfolio construction, such as long short-time memory. Less about enterprise efficiency or streamlining, it suffices to say deep-learning projects are the polar opposite of RPA—tackling the challenges at the extreme of what even the smartest financial engineer or quant could dream up. It is not about getting things done or scaling up an investment trend. This AI completely breaks the mold.
Somewhat unusually, these areas can all be categorized under the same technology umbrella.
Where to Expect Investment
Every iteration of AI is attractive for its potential, and there is no mutual exclusivity to deploying multiple kinds of AI across a stack. It is conceivable for a trading desk to use machine-learning-driven analytics to execute on a portfolio manager’s allocations, which have been developed with deep-learning techniques, and for the resulting trade and positional data to then be reconciled and post-trade processed by RPA bots on the back end. This hypothetical stack utilises three levels of AI across a single trade—a kind of AI ‘holy grail’.
Still, with firms on both the buy and sell sides of investment suffering a squeeze from capital constraints and margin compression, respectively, chief technologists must be particular about their spend. They are therefore focused on areas in which AI can bring maximum benefits quickly and at reasonably low onboarding and integration cost.
The research broadly echoes that message: almost 75 percent of respondents highlight either data analytics or cost reduction and efficiencies as key AI benefits. Risk management comes third with roughly one-quarter, while cash/liquidity management is still viewed as a largely manual undertaking—indeed, many firms struggle to install any kind of automated platform for these functions, let alone one that deploys AI. That is good, if perhaps unsurprising, news for bot-based and machine‑learning AI applications focused on bottom lines and supplementing the analytics estate.
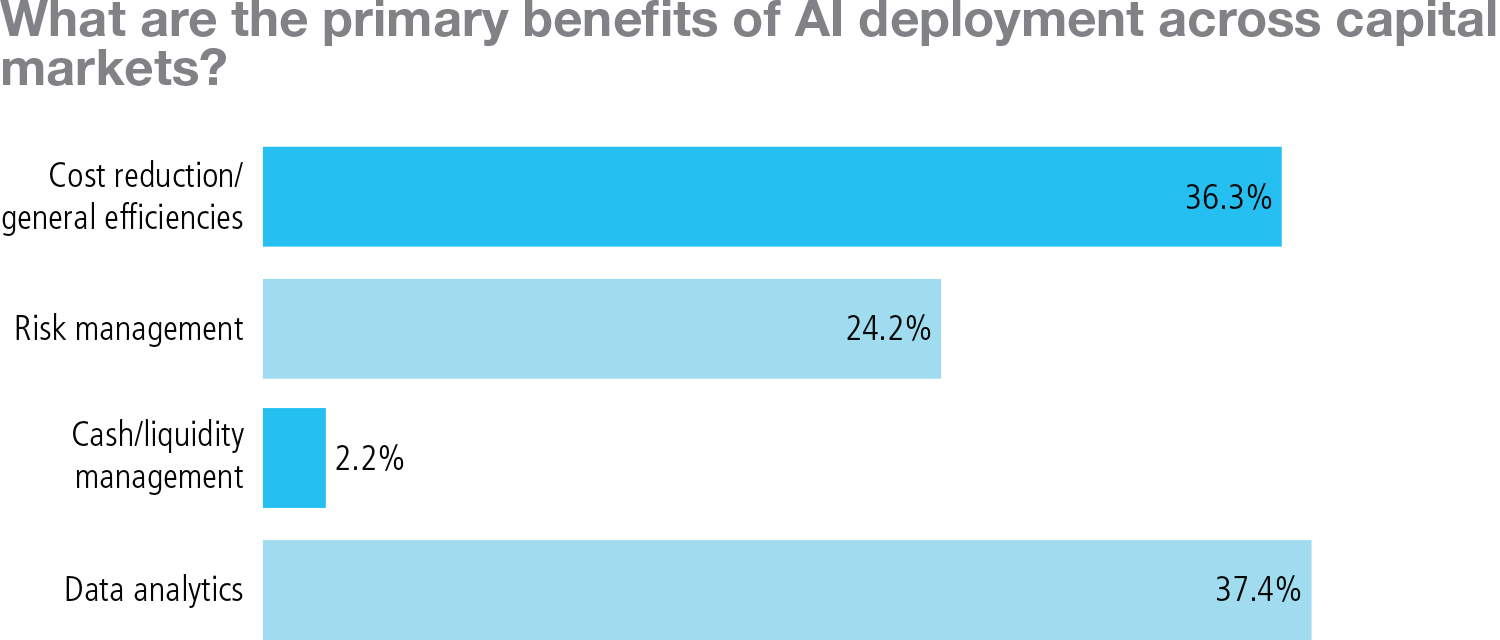
However, two interrelated follow-up questions paint a more subtle picture. First, those surveyed indicate that front-office activities—algo trading, advanced execution and decision support—are most likely to be impacted by AI in the future, with the back office coming next and the coterie of risk, compliance, performance and reporting functions in the middle office coming last.
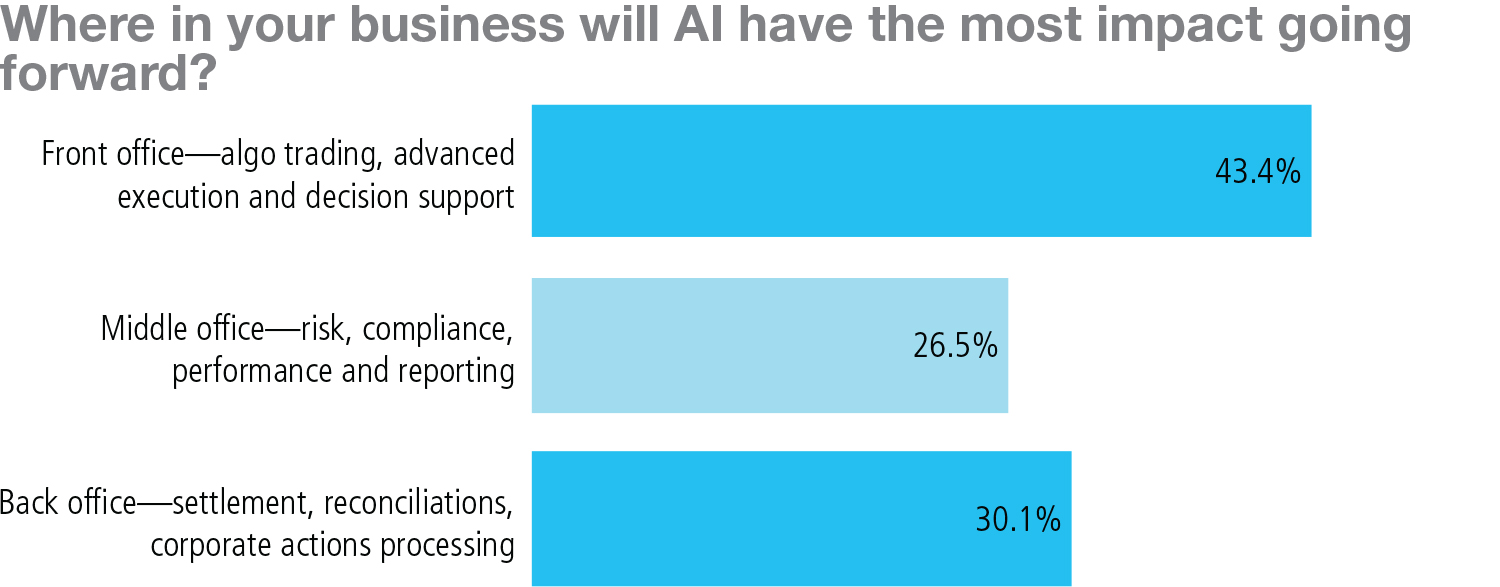
The front office may get an additional bump because so much of trading order management and electronic execution has long since lost the human trader’s touch. This is interesting, given that the middle office has long been a source of technological headache by serving both trading desks and institutional functions in back. If anything, that precarious position should make the middle office more, not less, ripe for AI-fueled enhancement.
Additionally, respondents indicated a difference—consciously or perhaps unconsciously—between AI’s best business cases. Here, cost reduction, reduced processing time and greater efficiencies come second to broadly construed data analysis improvements. AI should make decision-making easier by harnessing better information faster. But it is the third-placed business case—data accuracy and intraday availability in risk, collateralization and cash management, with only 14.5 percent—that piques interest, if only because these challenges are concrete and often cited as the most pressing and potentially most operationally costly computational issues for firms in today’s post-crisis, regulation‑heavy operating environment. Why couldn’t AI help move these areas closer to intraday and real-time? As for the honest 6 percent suggesting a tough path to an AI business case of any kind, they may well be the minority—or they could be in denial—but that too is certainly a large enough number to intrigue.
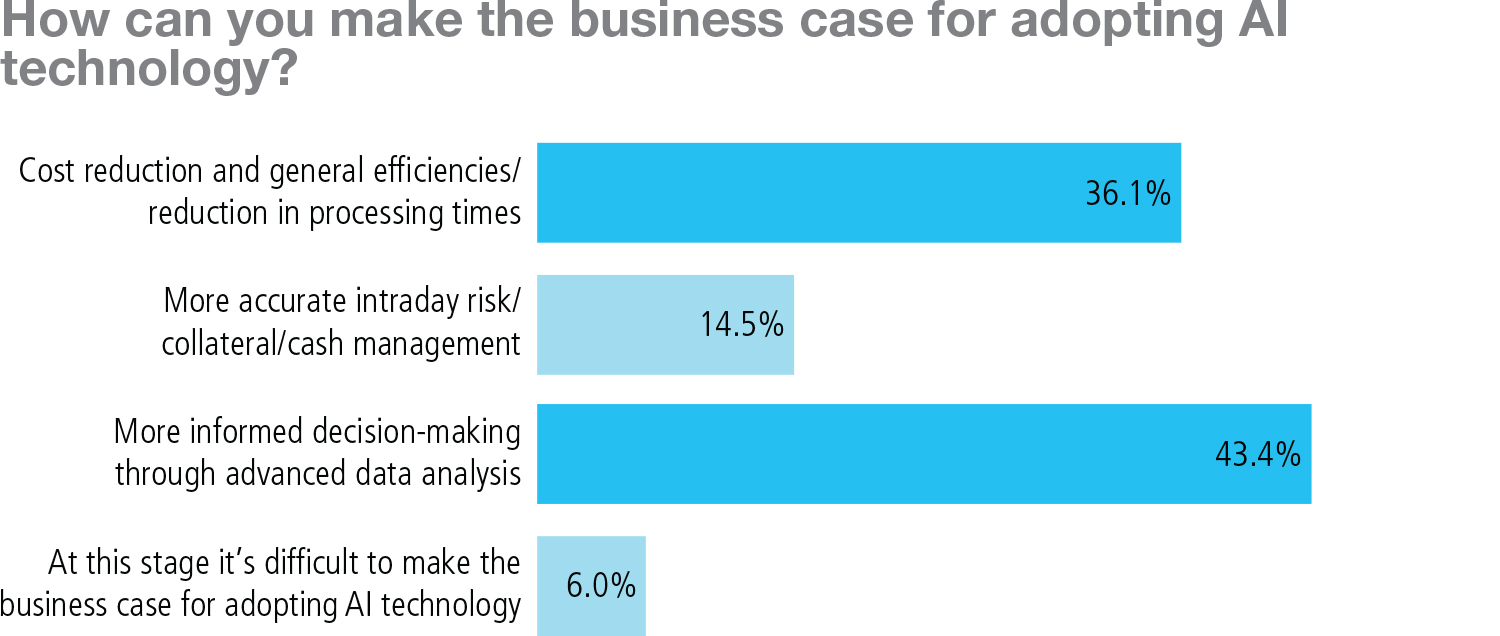
The assembly of these results suggests that firms today still view AI as providing benefits in abstraction—aiming for sensible low-hanging fruit rather than being able to tackle long-standing technological challenges at points of historical friction.
Where the Hesitancies Lie
For all the AI evangelism dominating 2018, the research also revealed some lingering pain points worth considering—and improving upon.
Nearly half of those surveyed said a lack of experience or know-how presented the greatest challenge to AI adoption. That number is one of the largest among any response to the survey, and would also seem to drive the second‑greatest challenge response—that almost one-quarter said the criticality of AI is difficult to prove for business-side sponsors.
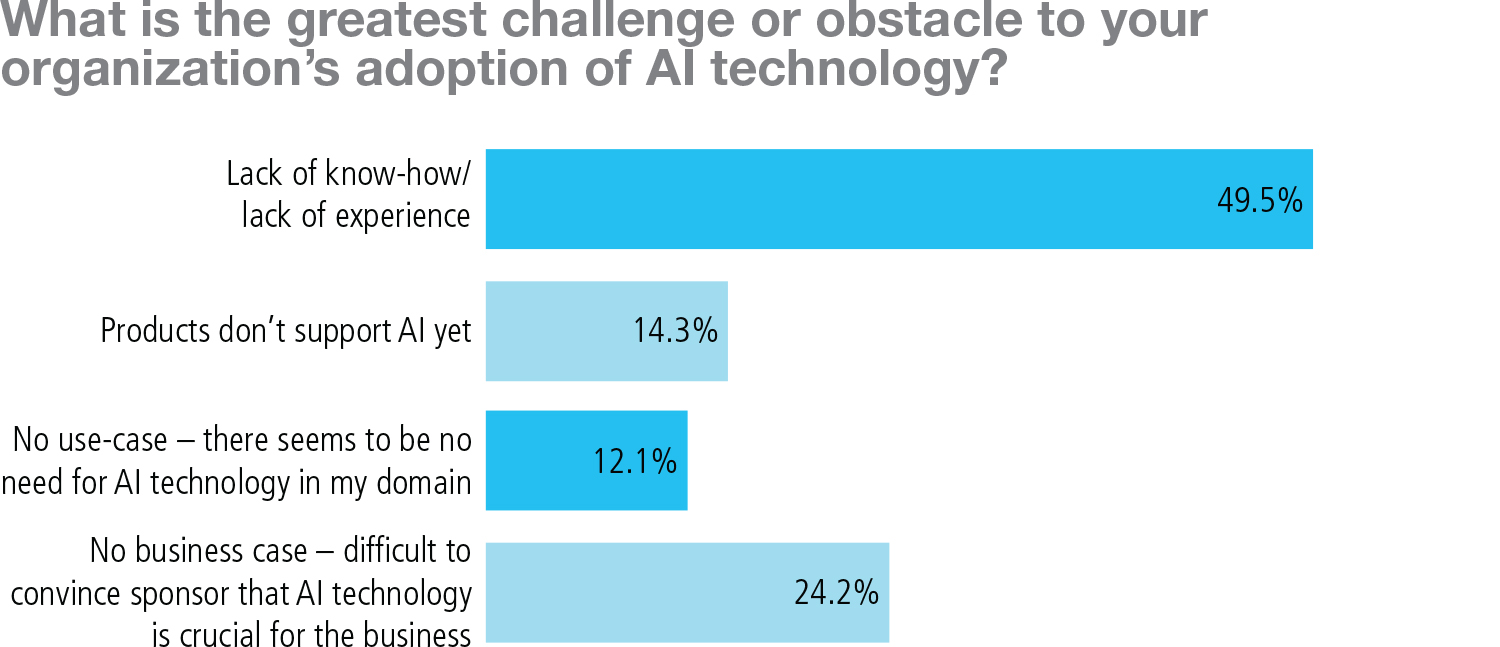
Again, these results point to the importance of metricizing the automation performance and efficiency gains that the different AI subgroup technologies can provide—either in terms of operational cost reduction in the case of RPA or amplification of investment innovation across client segments, as with machine learning. Mission-critical status is difficult to prove; even for conventional technology refresh, this is a reliable—albeit usually poor—excuse not to change the organization. It could be true that, at this early stage, many personnel will be unfamiliar with how AI works—and may even be concerned about the possibility of AI replacing them. As more first-movers take the plunge, however, evidence of the extent to which AI affects organizational evolution should emerge.
There is further hesitancy in proof-of-concept (PoC) work regarding AI. This simple measure of the technology’s penetration reveals a mixed result—about one-third of those surveyed report that a PoC was undertaken and that the experience was positive, and another 27.5 percent plan to run a PoC in the near future. Conversely, 18.7 percent of respondents are unsure of AI’s value after running a PoC—which is somewhat crucial to the aforementioned concerns—and more than one in five say there is no PoC in the cards for their organisations at this time.
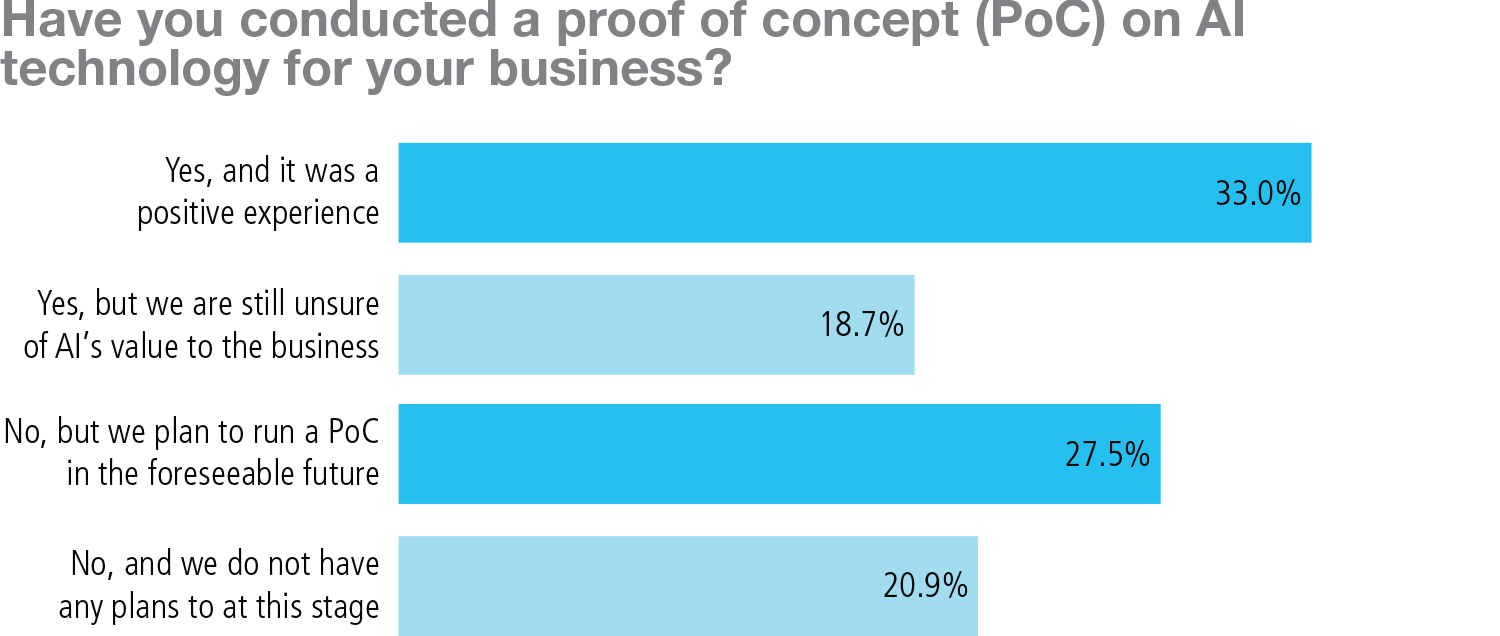
It is encouraging that nearly 80 percent of firms already have or intend to run a PoC on AI, which, taken in the context of the previous question, can reasonably suggest that many PoCs are being run at institutions where more AI knowledge is necessary. With regard to disruptive technologies, many technologists suggest the only way to come to understanding it is to bring it into the environment, play around with it and discover its kinks. That numerous PoCs have turned out inconclusively should not come as a surprise—almost twice as many have had a positive experience—although, when added to the other ‘non-positive’ responses, it gives a sense of the room for progress. More than two-thirds of the industry has yet to realize AI’s benefits.
This, too, will change with time, but one reason for the delay is proven in the response to a question regarding the sourcing of AI. More than 70 percent of respondent firms reported that their AI will come from an external provider or a mix of external and internal resources. This should immediately help to alter the perception of AI as a kind of ‘black box’, but it also implies obvious issues around vendor selection, stack integration and wrapping compliance around the solution. For many large institutions and significant implementations, these matters can take years to complete.
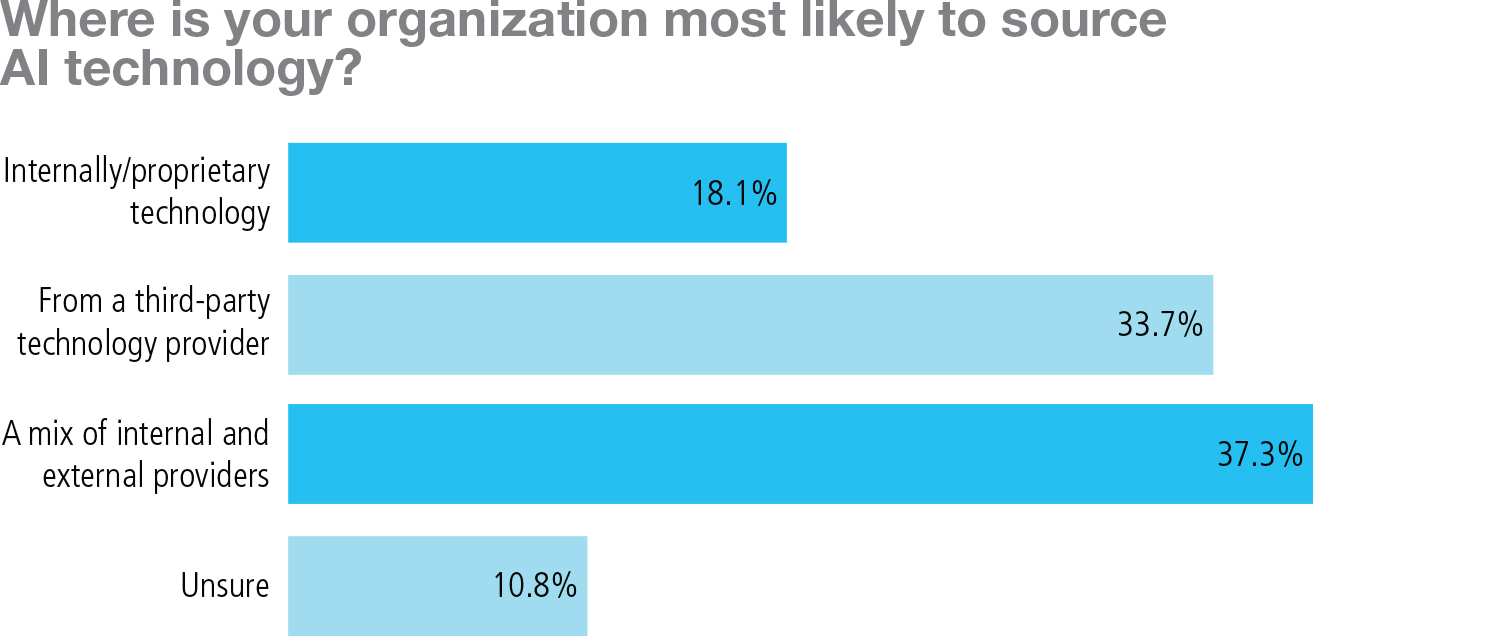
Regulated AI?
Inarguably, the most interesting response from the survey was to the final question around the need for AI technology to be regulated to reduce risks it might pose. Although technologically disastrous market events and the rise of fintechs has somewhat altered opinion on the issue in recent years, innovation in financial services is not traditionally viewed through a regulatory lens—at least not by default.
This is certainly not the opinion held by senior executives in the fintech community, who tend to view financial regulation as an impediment to recruiting the best tech talent, who often bring a libertarian perspective to their teams and to the work, and who can examine some of the world’s game-changing fintech and rightly conclude that “it was invented and thrived because the approach was ‘hands-off.” This is not the case with AI.
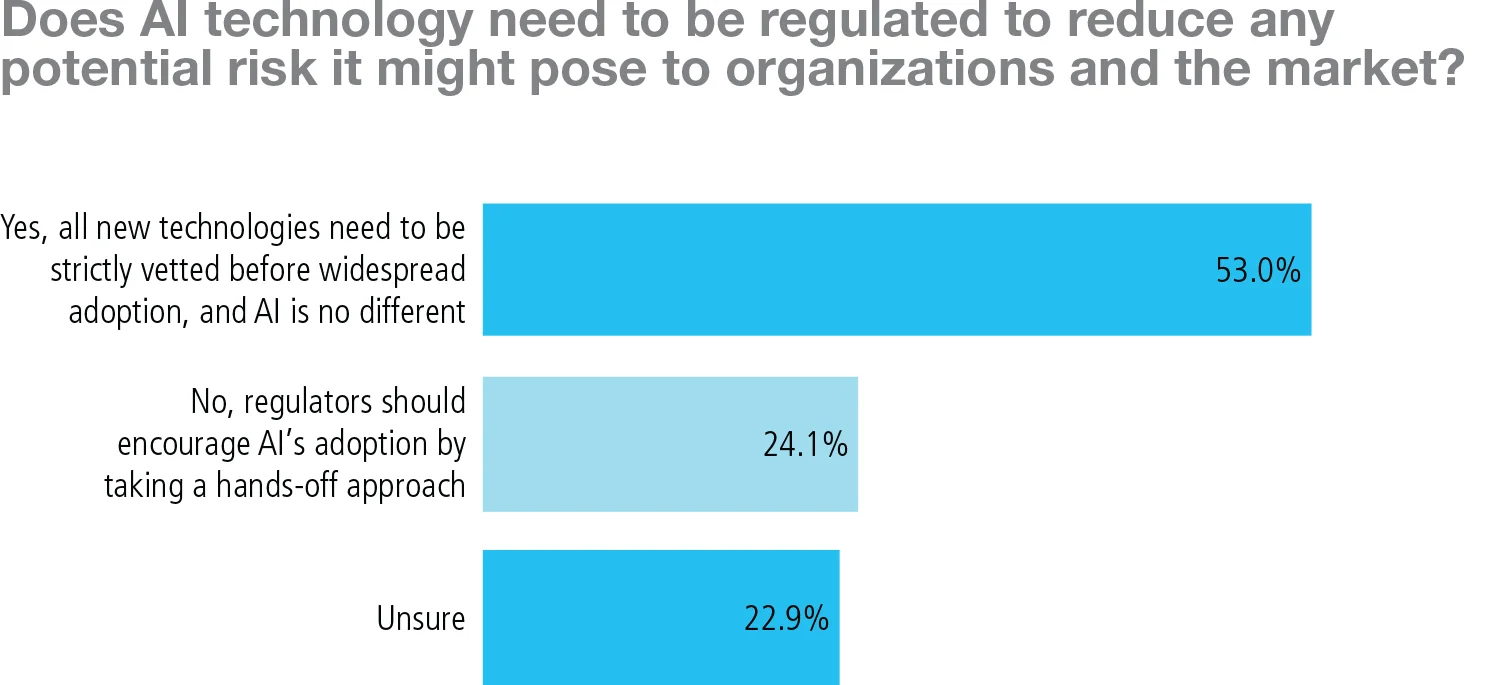
Over half of respondents said the technology should be “strictly vetted” by regulators, more than twice the number that said it should not (24.1 percent). Upon instant reaction, for an industry that has tirelessly coped with ever‑deepening regulation for a decade, this is surprising.
However, considering the knowledge deficit regarding AI, perhaps it is not. Firms today simply aren’t willing to assume new risks—even technological risks—where they lack domain expertise to lean upon. They see that the US Securities and Exchange Commission and its Division of Economic and Risk Analysis has frequently made waves in the past couple years, discussing turning its own new machine-readable reporting and machine-learning investments into market surveillance, for example.
As pointed out by a pair of Sidley Austin attorneys earlier this year for an op-ed in The Hill, there is an acute sense that US-based legislation is piecemeal and inconsistent on what standards, requirements and consequences should be in place to allow AI to operate effectively and what would lead to it running amok. This question extends to the subsets of AI as well—should deep learning be overseen in the same way as bots completing simple tasks or cleansing confidential client data?
To understand the extent to which AI is now fully in the popular consciousness, one needs only to look to some of the world’s most famous scientists and technologists—skeptics such as Elon Musk, Jack Ma, Bill Gates and the late Stephen Hawking, among others—who have colorfully warned of the threat that (strong) AI poses to human civilization.
However revolutionary and beneficial AI may prove to be, it is not merely a technological advancement. It is also a battle for human hearts and minds. Therefore, while many firms are seeking internal credibility for their AI applications, they are also seeking regulatory cover.
Conclusion: Qualified Optimism
With a number of unexpected insights into the evolving relationship between machines and financial services, this research has proven to be a fascinating study into what could be called ‘qualified optimism’ around AI. Much has changed in the six decades since the conception of AI, but some of the original issues remain perfectly crystallized in this new frontier for AI. From considering the suitability of AI and choosing the right technology, to the practical requirements and implications of adoption and the conceptual conundrums we all must now ponder, its influence is here to stay.
Finally, survey participants were asked a simple question: can AI give you an edge?
Nearly 80 percent said yes, although slightly more than half of these admitted they don’t yet know how to achieve this.
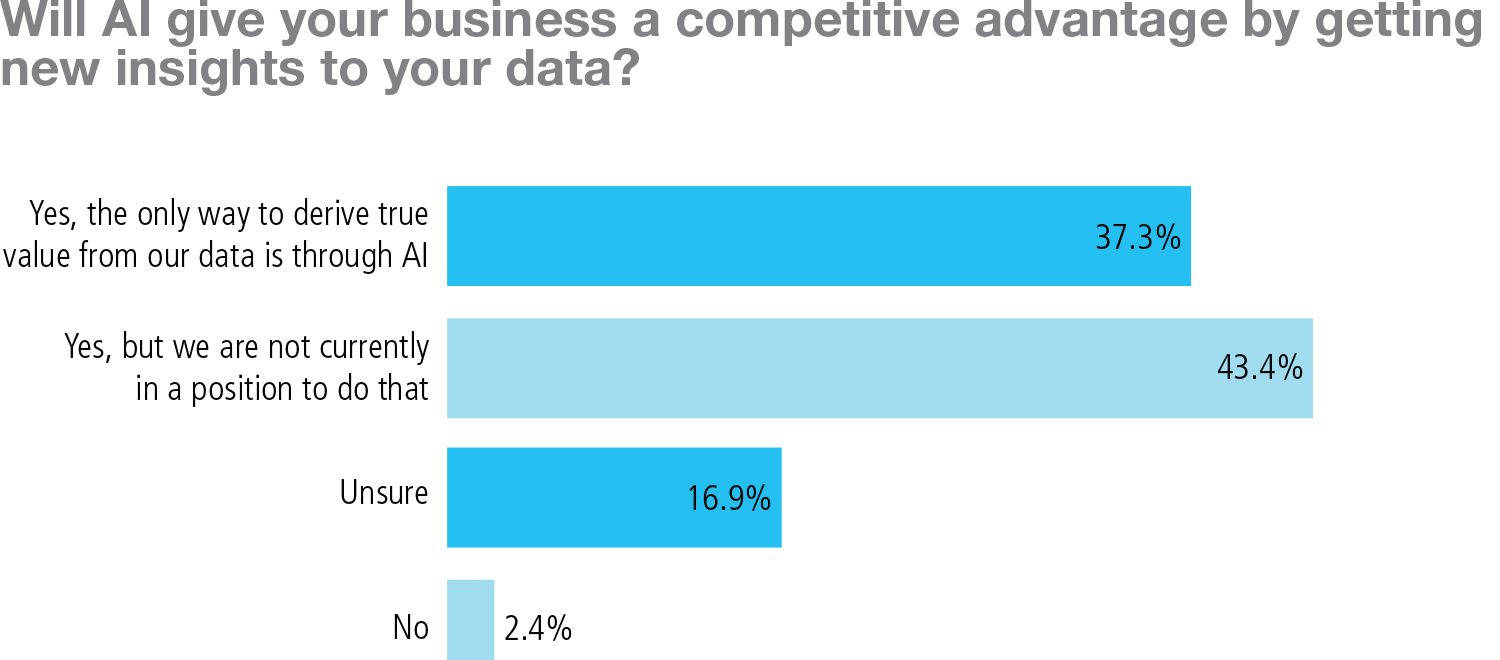
In the short life of AI, this has proven to be an age-old problem. But, with each passing year, each foundational step forward and each novel application, that readiness grows closer.
Sponsored content
Copyright Infopro Digital Limited. All rights reserved.
As outlined in our terms and conditions, https://www.infopro-digital.com/terms-and-conditions/subscriptions/ (point 2.4), printing is limited to a single copy.
If you would like to purchase additional rights please email info@waterstechnology.com
Copyright Infopro Digital Limited. All rights reserved.
You may share this content using our article tools. As outlined in our terms and conditions, https://www.infopro-digital.com/terms-and-conditions/subscriptions/ (clause 2.4), an Authorised User may only make one copy of the materials for their own personal use. You must also comply with the restrictions in clause 2.5.
If you would like to purchase additional rights please email info@waterstechnology.com