TOFIS Panel on Machine Learning, AI: ‘Try Everything, Kill What Doesn’t Work’
Panelists at the recent Toronto Financial Information Summit outline the key opportunities and challenges for machine learning and artificial intelligence in the financial markets.
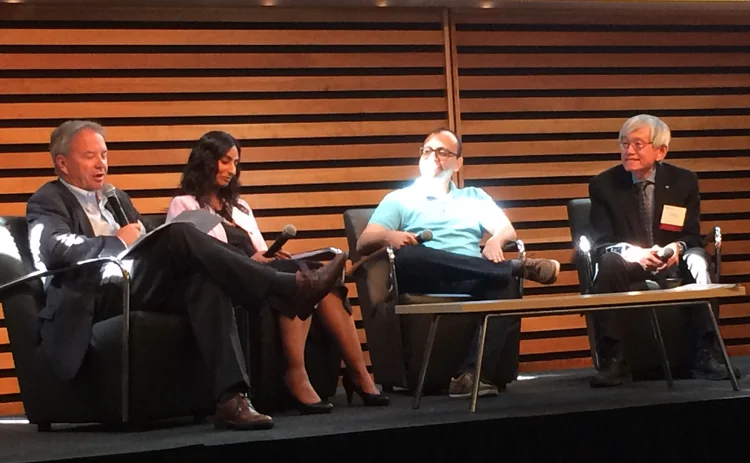
During a panel discussion specifically addressing how firms are using data to drive innovation, an audience poll revealed that the top challenges for attendees’ organizations are resources and access to data/data quality, attracting 56 percent and 31 percent of respondents’ votes, respectively, while 6 percent cited technology as their top challenge.
With resources under pressure, firms don’t have time to invest in long-term exercises, but must act quickly to take advantage of opportunities presented by new technologies and processes—and to discard those that don’t show promise.
“With innovation, you have to play with new technologies, and if you’re going to fail, fail fast—or I’d prefer ‘learn fast’,” said Saba Shariff, VP of digital security and data services at Canadian payments processing and communications service provider Symcor.
“If something doesn’t deliver early, you have to cut it off and move on. Try everything, kill whatever doesn’t work, and move forward with what works,” added Ajinkya Kulkarni, director of AI and machine learning at Scotiabank.
During the same panel, another audience poll revealed the ways that firms are integrating AI and machine learning into their corporate data strategies: 41 percent of those who responded said they are using AI and ML to support customer experience efforts, such as learning more about customer needs; 28 percent said they are using these tools to support revenue generation;22 percent said they are using them to achieve efficiencies, such as employee optimization; and 6 percent said they use them for risk management.
More specifically, 35 percent said they are using machine learning for compliance and fraud detection; 30 percent said they use it to support alpha generation; 15 percent each said they use it for risk management and transaction cost analysis/optimization; and 5 percent said they use it for marketing purposes.
On another panel at the same event, Kerry Khoo-Fazari, director of analytics integration and insights at CIBC, confirmed that Big Data and machine learning are making an impact at the bank. “They are giving us the ability to predict what you need, how much you need, and when and where you need it,” she said.
“Big Data and machine learning are at the heart of everything we do,” said James Rulli, business development executive at Data Capital Management, speaking on the same panel, adding that DCM uses these tools to determine entry and exit strategies for its trading.
However, others sounded notes of caution. “Machine learning and Big Data are not a silver bullet. Set your expectations realistically… and the rewards will be handsome,” said Eugene Wen, VP of group advanced analytics at Manulife, on the second panel, while Emre Konukoglu, quantitative research portfolio manager at the Canada Pension Plan Investment Board, warned that until legal frameworks are established to govern the reliability of data inputs and avoid market manipulation through sabotaging datasets such as social media, “it may be better not to put too much faith in these.”
Indeed, according to another audience poll conducted during the second panel, 42 percent of respondents are not using any kinds of machine learning techniques in their business, and speakers on both panels emphasized that utilizing new tools requires overcoming both technical and cultural challenges.
“Machine learning is probabilistic—rather than deterministic, where you program something to ‘do this, go there’,” said Chai Lam, director of solutions architecture at RBC Insurance. “A model is only as smart as the data you give it. So if you use a small amount of data, the result is going to be biased. It’s important to make sure that AI doesn’t actually take decisions, but makes recommendations to humans, who make the decisions.”
Leaving the decision making to people is key to getting them on board with—and positive about—automation. “You have data and technology challenges, but you’re also dealing with issues around changes in culture… and mindsets,” Kulkarni said.
With new types of data—often procured from anonymized consumer data—and collection mechanisms rising to the fore, another cultural change is being more mindful of data usage and “data ethics,” panelists said. “We need to ensure that legal, product, and security teams are in the room with us when we’re designing anything new,” said Symcor’s Sharrif.
Only users who have a paid subscription or are part of a corporate subscription are able to print or copy content.
To access these options, along with all other subscription benefits, please contact info@waterstechnology.com or view our subscription options here: http://subscriptions.waterstechnology.com/subscribe
You are currently unable to print this content. Please contact info@waterstechnology.com to find out more.
You are currently unable to copy this content. Please contact info@waterstechnology.com to find out more.
Copyright Infopro Digital Limited. All rights reserved.
As outlined in our terms and conditions, https://www.infopro-digital.com/terms-and-conditions/subscriptions/ (point 2.4), printing is limited to a single copy.
If you would like to purchase additional rights please email info@waterstechnology.com
Copyright Infopro Digital Limited. All rights reserved.
You may share this content using our article tools. As outlined in our terms and conditions, https://www.infopro-digital.com/terms-and-conditions/subscriptions/ (clause 2.4), an Authorised User may only make one copy of the materials for their own personal use. You must also comply with the restrictions in clause 2.5.
If you would like to purchase additional rights please email info@waterstechnology.com
More on Emerging Technologies
Waters Wavelength Ep. 295: Vision57’s Steve Grob
Steve Grob joins the podcast to discuss all things interoperability, AI, and the future of the OMS.
S&P debuts GenAI ‘Document Intelligence’ for Capital IQ
The new tool provides summaries of lengthy text-based documents such as filings and earnings transcripts and allows users to query the documents with a ChatGPT-style interface.
The Waters Cooler: Are times really a-changin?
New thinking around buy-build? Changing tides in after-hours trading? Trump is back? Lots to get to.
A tech revolution in an old-school industry: FX
FX is in a state of transition, as asset managers and financial firms explore modernizing their operating processes. But manual processes persist. MillTechFX’s Eric Huttman makes the case for doubling down on new technology and embracing automation to increase operational efficiency in FX.
Waters Wavelength Ep. 294: Grasshopper’s James Leong
James Leong, CEO of Grasshopper, a proprietary trading firm based in Singapore, joins to discuss market reforms.
The Waters Cooler: Big Tech, big fines, big tunes
Amazon stumbles on genAI, Google gets fined more money than ever, and Eliot weighs in on the best James Bond film debate.
AI set to overhaul market data landscape by 2029, new study finds
A new report by Burton-Taylor says the intersection of advanced AI and market data has big implications for analytics, delivery, licensing, and more.
New Bloomberg study finds demand for election-related alt data
In a survey conducted with Coalition Greenwich, the data giant revealed a strong desire among asset managers, economists and analysts for more alternative data from the burgeoning prediction markets.