How Ally found the key to GenAI at the bottom of a teacup
Risk-and-tech chemistry—plus Microsoft’s flexibility—has seen the US lender leap from experiments to execution.
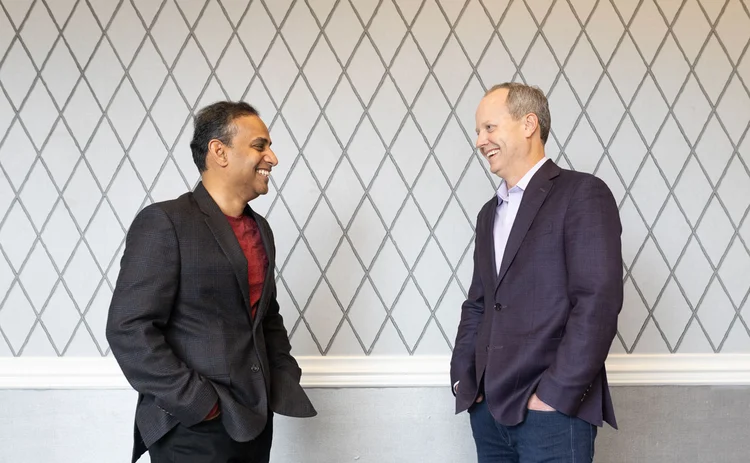
Over the past year, a daily ritual has been observed on the 26th floor of Ally Financial’s smart new office in Charlotte, North Carolina. It’s the VIP floor—quiet and carpeted—where the wide hallways belong to executive assistants, and sunlight filters through the blinds that protect cavernous boardrooms and offices. Here, at around 2pm, you will often find Ally’s head of risk and its head of technology sitting down together for a cup of tea.
The two execs had worked together on projects before, but “we weren’t friends back then”, jokes Sathish Muthukrishnan—the bank’s chief information, data and digital officer. He draws a grin from Jason Schugel, Ally’s chief risk officer.
Artificial intelligence (AI) brought them together. Like every other financial institution, big and small, Ally has been making a new push to see how it can benefit from the advent of generative AI (GenAI). Like only a few, though, it had a firm-wide instance in production by the end of last year—using large language models (LLMs) to summarize customer calls—and now has more than 400 on the launchpad.
Where other banks talk a good game, but complain privately about the regulatory, risk and third-party obstacles that are holding them back, Ally seems unflustered. The way Muthukrishnan and Schugel tell it, regulators have shown a keen interest, but have frequently been encouraging. The risks are being controlled by pushing new AI-driven use cases through the bank’s existing product approval process, and Microsoft—the bank’s main AI partner—has proved willing to listen and even to adapt.
One of the biggest things was just stepping back and recognizing that GenAI was relevant to a lot of different risk areas—it’s not just model risk ... For us, we wanted to make sure we were thinking about it holistically
Jason Schugel, Ally Financial
The latter claim, in particular, will be a surprise to some. Risk managers elsewhere in the industry see Google, Microsoft and other tech titans as unsympathetic to concerns about the copyright risks that might be incurred by users of GenAI, and unhelpful when fussy, heavily regulated banks ask for help validating their models. The vendors’ attitude is sometimes described as “take it or leave it”.
Muthukrishnan recognizes the outline of that story, but says it played out differently for Ally.
“Based on guidance from our risk partners, we said: ‘These are the risks we are seeing and taking as we explore your product, and how can we change the contract?’ Initially, the stance was ‘one contract fits all’. But it’s no longer that. They have modified the contract. They’re listening to their customers. And it has helped us move forward with them.”
Ally didn’t just ask for changes. It also argued that accommodating those changes would benefit Microsoft.
“We started to expose the limitations of using their LLM, especially within a regulated institution. We were articulating that it could be a big win for them if people saw a tightly regulated financial institution use their LLM to get technology into production. And we saw them turn around and address some of the concerns we had raised,” says Muthukrishnan.
Microsoft has gone on to publicly trumpet Ally’s use of AI. In the tech firm’s earnings call on January 30, its chief executive, Satya Nadella, called out Ally—along with two big non-financials—as an example of the growing use of AI among the biggest US companies.
Is Ally getting special treatment from Microsoft? Muthukrishnan doesn’t think so: “I wouldn’t call it special treatment. I would say they are being terrific partners.”
Over the past year-and-a-bit, the bank has made a host of decisions about how to use this new technology, and how to manage the attendant risks. The execs who led the effort have developed strong views—and are willing to offer some tips to those who want to follow in their footsteps.
One obvious risk mitigant is a decision that only ‘internal’ uses of GenAI will be considered for now—nothing customer-facing. Another step that Schugel believes has helped the bank progress was its decision to express GenAI risk appetite qualitatively—setting a range of conditions that have to be satisfied, such as human oversight for every use case—while some banks have tried, and struggled, to lay down more quantitative guardrails. Approval bottlenecks, meanwhile, are handled in part by developing what Schugel describes as ‘patterns’—sets of controls developed for one GenAI application that can be copied across to other, similar applications.
When it comes to regulation and regulators, Schugel’s belief is that instances of GenAI can be validated using existing US model risk guidance, but there is an important caveat: Ally has been validating the function of the model based on its outputs, rather than pulling apart the underlying third-party LLM itself. He also believes the existing guidance will eventually need to be updated. On interactions with regulators—and there have been plenty of these—Schugel says Ally has been following a simple, crucial principle to “share everything, up front”.
Ally is also taking a sharing approach to its story. After a one-hour, double-header interview at the Charlotte office on March 26, both Muthukrishnan and Schugel offered a reprise to delegates at a Risk.net conference in the city the following day. The quotes in this article are taken from these two conversations.
Beyond model risk
Ally’s GenAI journey began—like many firms—with a backward step. In early 2023, as teams across the bank started looking for ways to use OpenAI’s recently unveiled update to ChatGPT, Schugel intervened. He wanted to be sure the various risks associated with this new technology were properly understood and controlled. The decision was taken to bar use of ChatGPT throughout the bank, with the exception of a ‘lab’ function within technology, where experiments could continue.
“That was not a popular decision, but it was the right one,” Muthukrishnan concedes.
It meant the risk function had blocked access to a potentially transformative new technology. Schugel wanted to find a way to unblock it. Some banks have approached this challenge by treating GenAI as a new class of model, so handed it to model risk teams whose job is to document and validate new models, monitor existing ones, and ensure they are working as intended. Some of these tried-and-true steps are difficult to apply to third-party models of any kind, let alone to an LLM that has been trained on a combination of a vast public corpus of data, and rounds of human guidance. In addition, the model risk process is generally not used to dealing with some of the awkward, hard-to-capture exposures that come with this new technology.
“In my seat, one of the biggest things was just stepping back and recognizing that GenAI was relevant to a lot of different risk areas—it’s not just model risk. I know some of my peers started there and tried to expand from it. But we were thinking about it in terms of technology risks—hallucinations—and operational risks relating to privacy and data. There’s also reputational risk, and there could be compliance risk, depending on how you use it. So, for us, we wanted to make sure we were thinking about it holistically,” says Schugel.
He argues that banks also face strategic risk if they are slow to embrace GenAI—they could be blindsided or left behind by faster-moving rivals. Ally decided it could not afford to be slow.
Rather than treating GenAI as a model risk problem, Schugel chose to approach it as a product risk problem—funneling each instance of GenAI through Ally’s existing new product approval process. This had a number of advantages, chief among them the presence at various stages of the review process of business lines and corporate functions, as well as second-line risk managers who collectively cover the 12 forms of risk—and 30 ‘child’ exposures—that Ally identified as its material risks.
The block was removed, no new bureaucratic layers were created—and uses of GenAI were suddenly exposed to scrutiny from a whole range of experts and stakeholders, rather than being the preserve of model risk specialists.
“It was a stroke of genius,” says Muthukrishnan. “The new product committee has constituents thinking about every risk dimension across the company—12 at the top and then multiple sub-levels. It was a very simple idea, but it was also very powerful. Everybody that is part of the committee understands the risks they are assessing. They understand the process through which they assess the risk. They know the questions to ask. And that was what allowed us to accelerate from experiment to execution.”
Based on guidance from our risk partners, we said: ‘These are the risks we are seeing and taking as we explore your product, and how can we change the contract?’
Sathish Muthukrishnan, Ally Financial
It wasn’t the only thing. Ally’s risk team also laid down a trio of overarching controls. First, GenAI would be applied to internal-facing use cases only. Second, no information that could identify any individual would be shared with an LLM, and no Ally data would be allowed to feed into a model’s training data. Finally, all use cases would be accompanied by both human intervention as well as additional controls focused on training and oversight.
Some demands were also made by the risk function of the technology team—notably, Muthukrishnan recalls being told that Ally would need “a ton of outcomes, so we can validate the model”.
In response, the bank’s IT team built an internal platform—Ally.ai—which allows staff at the bank to interact with multiple, privately hosted LLMs through a single interface that has its own security features built in. Among other things, interactions with the underlying LLMs are logged, which enabled Muthukrishnan to give Schugel the model outputs he wanted for validation purposes.
Once the review process had been decided, the overarching controls agreed, and the platform built, Ally was able to return to the original use case that had been on the launchpad months earlier.
‘Don’t make stuff up’
The plan was to use ChatGPT to summarize conversations between Ally’s customers and its call center staff.
Muthukrishnan explains why: “Our agents have this tough task of referring to multiple screens, drawing on multiple applications, getting different data points, as they interact with customers. All while tracking their conversation with the customers. An average call is between 13 and 15 minutes. That’s a long time for a human to be multitasking, trying to answer the questions, not knowing what the next question might be from the customer, while also keeping track of everything that they’re doing. And at the end of the call, they had to summarize the conversation and file it.”
The summaries are used for training purposes, to ensure Ally has a history of each client’s requests, and to demonstrate to regulators that customers are being treated fairly. If each call could be auto-transcribed and summarised by AI, then agents would have a simpler job—reviewing the summary and approving it, rather than typing it out themselves.
“It’s a 15-second task, instead of a 15-minute task,” says Muthukrishnan. Across the 10,000 calls that Ally’s staff complete daily (on average), those saved minutes quickly add up to some big numbers.
In theory, anyway. The testing phase of the project involved running the ChatGPT summarizer in the background—leaving humans to do their work as usual—and handing 60,000 prototype summaries to the risk function so they could check the model was working properly.
It wasn’t. In some cases, where a call connection temporarily dropped—resulting in a real-world conversation dotted with ‘Hello?’ and ‘Can you hear me?’—the AI hallucinated entire exchanges to fill the airtime. At other times, it struggled to understand accents. In at least one case, a customer was watching the award-winning TV series Breaking Bad while speaking to Ally staff, so the call summary was polluted with drug talk and swearing—not ideal training material.
Ally’s IT team went back to work, fine-tuning their inputs—what’s now called ‘prompt engineering’. The LLM was instructed to assume the persona of a financial institution, serving customers of that institution, and was told not to “make stuff up”, as Muthukrishnan puts it.
This kind of work continued, gradually improving the quality of the model outputs. Initially, only 19% of summaries required no human editing. Today, more than 80% of the summaries clear that bar.
The model made its way through the product approval process, and was eventually presented to Ally’s board last December. It was then cleared for use.
After first spooking some call center staff, the auto-summarizer has now won friends.
Muthukrishnan says: “Many of them say it has given them the freedom to have a singularly focused conversation with the customers—they no longer have to take notes when speaking to them—and they also believe the summarizer is capturing more of the conversation than they would have captured as a human. It picks up things they might have forgotten. And now we have all this data, it gives us the ability to go back and train our customer care associates appropriately and consistently, saying: ‘This was a better call, this was the right conversation to have, these were the right actions to take.’”
Were those associates right to be spooked, though? Now that Ally can use AI to do parts of the job, will it be cutting staff?
Muthukrishnan says that’s not the plan—but, as the firm grows, it may now need to hire fewer additional agents to handle the extra volume of calls: “We see it as an augmentative technology. We don’t see it as a replacement technology.”
There are lots of things that Ally is now looking to augment. As of late April, Muthukrishnan says there were more than 400 use cases in the review-and-approval process, with around 70% of them based on GenAI, rather than other forms of machine learning and artificial intelligence. And the number of use cases “keeps increasing”, he adds.
Five by five on 11-7
From a purely risk management perspective, Schugel says the first use case was a hit. For one thing, the summaries were always a form of risk control—a resource that was used to train new staff, and to address regulator queries about fairness. If those summaries are now more complete and more consistent, then it should lead to an improvement in the quality of Ally’s training, and in its ability to answer regulator questions.
As well as doing old things better, Ally can do new things, too. Summaries are auto-tagged and categorized—by the overarching Ally.ai platform, rather than by the underlying LLM—so they can be sorted and searched more effectively. The firm could interrogate them, en masse, to look at trends among clients—the questions they’re asking, the help they’re looking for—although it has not started to do so yet.
Schugel says: “It’s made an existing control stronger. Looking at it through a risk management lens, that was actually the exciting part.”
In addition, the risk function learned a lot about GenAI, and about how to address some of the attendant risks. Schugel highlights model documentation as an area that had to be tightened up—a common shortcoming in the development of a new model, he says—and also monitoring, where Ally’s risk managers had to revise the metrics they were using to assess the performance of the tool.
And, of course, there were those early tangles with Microsoft. The two execs do not rip the lid off those conversations, but they offer some insight into the ground covered. Broadly, two classes of concern are mentioned: those relating to copyright and intellectual property (IP); and those relating to documentation and validation.
Our agents have this tough task of referring to multiple screens, drawing on multiple applications, getting different data points, as they interact with customers ... [With AI, producing a call summary is] a 15-second task, instead of a 15-minute task
Sathish Muthukrishnan, Ally Financial
The copyright and IP risks associated with third-party LLMs are obvious: if you are using someone else’s model to generate new content, do you own that content, or do they? And if the content was generated on the basis of training data that is found to have breached someone else’s copyright, are you in breach as well?
Schugel says Ally and Microsoft are clear on ownership—any outputs generated by the bank, using ChatGPT, belong to Ally. He is more hesitant on copyright, saying, when asked on stage, that he would “prefer to move on”.
In the prior day’s interview, Schugel alludes to “things you can do from a contracting basis that can also limit your risk”, and when asked whether indemnification is one of those things, says: “I don’t want to share that level of detail. But, yeah, I would say those discussions are happening.”
Documentation and validation issues with third-party models are not unique to LLMs. Banks have long struggled to extract the information their regulators expect—often commercially sensitive information—from model vendors. But the problem is magnified when the model in question has billions of parameters.
Ally did not set out to fully validate that underlying model—it sought instead to understand the model, and ensure it was comfortable with its design, its training and, of course, its performance.
“The summarisation use case was really our first introduction to validating the model behind that, and so really kind of working with [Ally’s tech team] to say: ‘This would be the level of documentation that we would expect. These are the insights we need to get comfortable with it,’” says Schugel.
When it came to Microsoft, “we needed to understand how they thought about it, how they cared for it”, he adds.
The end result passed muster for Ally. And Schugel says the process also satisfies the model risk guidelines published by US bank regulators in 2011—a 21-page ‘letter’, known as SR 11-7. At a high level, those guidelines make three demands of the validation process: banks must evaluate the conceptual soundness of all models, analyze their outcomes, and monitor their performance on an ongoing basis.
“For that first model—the prompted summarization use case that we had—we were able to go in and validate all the steps we took, and were able to form an opinion on it, and get comfortable with it. And we did that using the steps outlined in 11-7,” says Schugel.
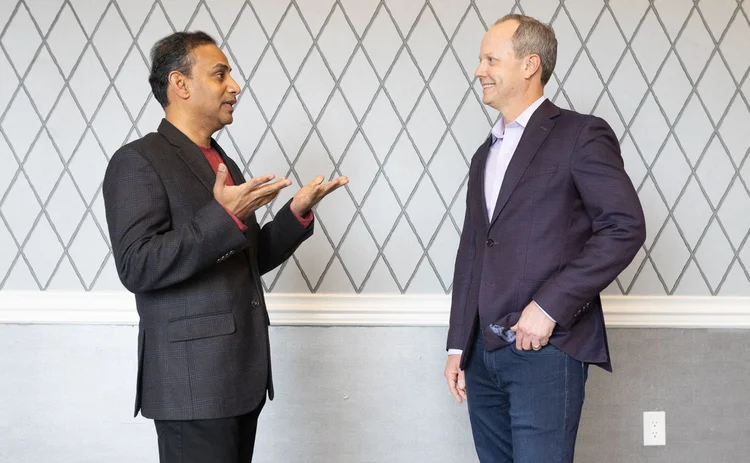
The process was different to the approach that Ally would take with a classical pricing, valuation or risk model, though.
“If you try to validate an open-source model or something like that, that’s extremely difficult. So, we really have focused our attention more on understanding it—you know, understanding where the hallucinations are, where there could be other weaknesses, and training people around where that could be. You still need to do work around the underlying model, but getting to a true validation conclusion is very difficult,” says Schugel.
Are Ally’s regulators happy with that?
It’s hard to know. Schugel says that “they don’t always give you the ‘yes’ or ‘no’”, but Ally made sure its regulators knew what its plans were in advance.
The bank may have had no explicit verdict, but there were some implicit signals. Muthukrishnan and Schugel had some meetings with regulators together, discussing some of the occasions on which technology plans had run into risk management realities.
“One of the big things they want to see is effective challenge, so, as we were running through this with them, I think they appreciated the back and forth. They smiled every time there had been some kind of friction,” says Schugel.
The explosion of interest in AI generally, and GenAI specifically, has raised regular questions about the suitability of model risk rules that—at least in the US—were written more than a decade ago, with conventional models in mind.
Some banks claim SR 11-7 cannot be applied to LLMs. Regulators disagree, says Schugel—but that doesn’t mean the current guidance will remain in place forever.
“We’ve heard directly from the author of 11-7 that the expectation would be that these new models do fit within there. But that guidance has been there since 2011—that’s before GenAI—so we would absolutely agree that, at some point, there will be enhancements or new regulations,” he says.
And while it might take some time for regulation to catch up, supervisory practices—notably examinations—are already changing.
For that first model—the prompted summarization use case that we had—we were able to go in and validate all the steps we took, and were able to form an opinion on it, and get comfortable with it. And we did that using the steps outlined in 11-7
Jason Schugel, Ally Financial
“On the examination side, they’ve definitely touched on it—they’re utilizing existing exams to throw in some ability to look at it, and have started to get under the cover a little bit,” says Schugel. “They hear from us, but also want to go a little bit deeper. So, we definitely have seen that, but I absolutely think there’s more to come.”
In the meantime, Schugel’s teams have plenty to work on—there are those 400-plus AI use cases, for one thing. And because those cases all have to make their way through the product approval process, it’s not just the risk function that will be involved in vetting them.
Muthukrishnan says Ally’s senior execs have an eye on this: “Collectively, as an executive leadership team, we talked about the number of use cases that are coming at us. It’s easy for technology to execute it, but I have empathy for Jason’s organization because they have to look at each of those use cases as a product. They have to assess risk on top of their day jobs. So, their day job hasn’t gone away. And now they have to do all of this.”
The use cases are not all unique, though. Part of Ally’s solution to the potential risk management bottleneck is to identify ‘patterns’ that link the cases, and that imply accompanying, somewhat standardized sets of controls. Technology and risk have worked together—again—to identify these patterns. Schugel’s team then has a short cut to follow when making sure the appropriate controls are in place.
Somewhere along the way—neither man is quite sure when—all of this back and forth between technology and risk management gave rise to their ‘teatime’ ritual. Muthukrishnan and Schugel found that their teams were working together so much that they needed to be in almost constant contact.
For anyone hoping to replicate this risk-plus-tech chemistry, in the belief that GenAI rewards will be found at the bottom of a teacup, Muthukrishnan has some words of warning: it’s not that simple.
“My counterparts at other banks are finding it very difficult to go from experimentation to execution. You need years of sustained investment. You need the foundations to be built already—such as having your data ecosystem together, having your applications run on cloud, a culture that that allows for innovation, and an executive team that sets the right tone. Given the way the economy is, it’s easier to just focus on your core business. We have done both—focus on the core, and continue innovating,” he says.
Only users who have a paid subscription or are part of a corporate subscription are able to print or copy content.
To access these options, along with all other subscription benefits, please contact info@waterstechnology.com or view our subscription options here: http://subscriptions.waterstechnology.com/subscribe
You are currently unable to print this content. Please contact info@waterstechnology.com to find out more.
You are currently unable to copy this content. Please contact info@waterstechnology.com to find out more.
Copyright Infopro Digital Limited. All rights reserved.
As outlined in our terms and conditions, https://www.infopro-digital.com/terms-and-conditions/subscriptions/ (point 2.4), printing is limited to a single copy.
If you would like to purchase additional rights please email info@waterstechnology.com
Copyright Infopro Digital Limited. All rights reserved.
You may share this content using our article tools. As outlined in our terms and conditions, https://www.infopro-digital.com/terms-and-conditions/subscriptions/ (clause 2.4), an Authorised User may only make one copy of the materials for their own personal use. You must also comply with the restrictions in clause 2.5.
If you would like to purchase additional rights please email info@waterstechnology.com
More on Data Management
Fitch claims 20% developer productivity boost using AWS GenAI tools
The vendors have expanded an existing deal to include new Amazon tools that have helped Fitch modernize its infrastructure and applications.
US sovereign wealth fund project highlights the private assets problem
Firms have a growing appetite for private markets, as shown by President Trump’s proposed creation of a US SWF. GoldenSource’s James Corrigan writes that to ensure success, core technology must be built on strong data foundations.
DLT and digital contracts for market data: Has the hammer found the nail?
Waters Wrap: A new platform that a custom-made DLT underpins is coming to market. Anthony examines its merits and, surprisingly, finds a lot.
Bond CT hopeful Etrading unveils free tape prototype ahead of tenders
The vendor hopes to provide the long-awaited consolidated tape for bonds in the EU and the UK, demonstrating its ability to do so through ETS Connect.
Big xyt exploring bid to provide EU equities CT
So far, only one group, a consortium of the major European exchanges, has formally kept its hat in the ring to provide Europe’s consolidated tape for equities.
Orchestrade resists SaaS model in favor of customer flexibility
Firms like Orchestrade are minimizing funds and banks’ risks with different approaches to risk management.
Hyperscalers to take hits as AI demand overpowers datacenter capacity
The IMD Wrap: Max asks, who’s really raising your datacenter costs? And how can you reduce them?
New FPGA component aims to curb co-lo costs
Hardware ticker plant provider Exegy is working on a new FPGA solution that it says will free up costly processing power on firms’ existing co-lo servers.