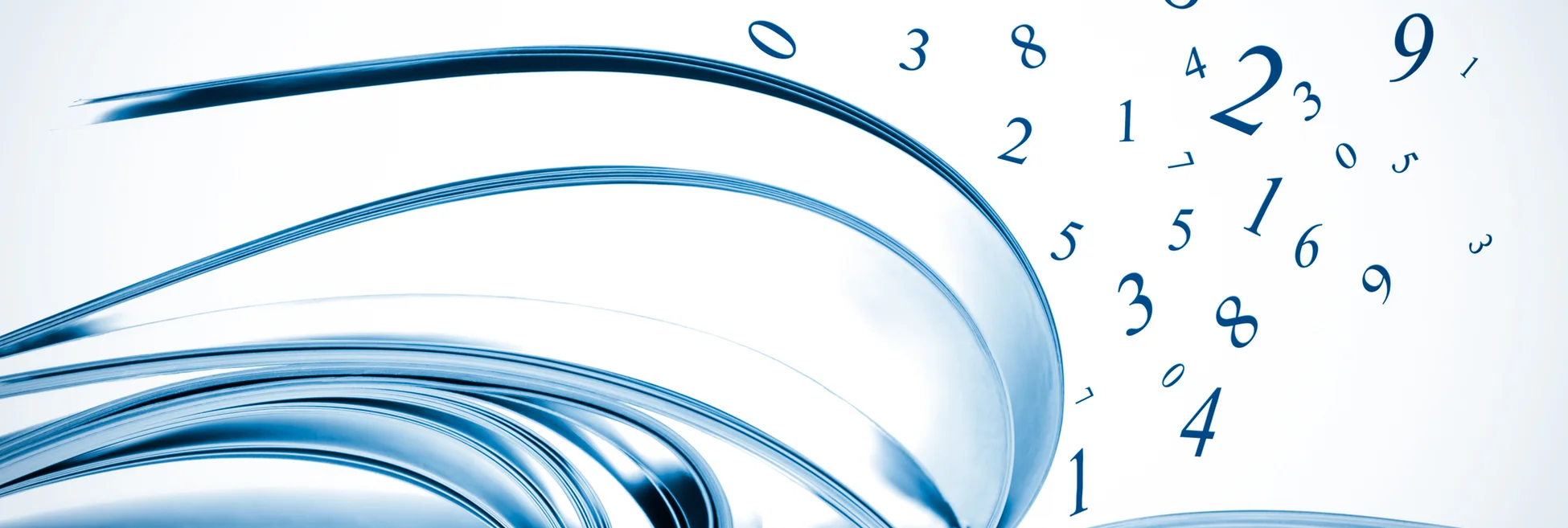
Allianz Global Investors Adopts NLP Signals in Equities
The investment manager's move to tackle unstructured data is starting with sell-side analyst reports.
Allianz Global Investors is adopting natural language processing (NLP) signals across its entire equities division, starting with signals generated by analysing sell-side analyst reports.
Two years ago, the San Diego-based quantitative team at the €535 billion ($588.3 billion) asset management firm started a NLP effort to tackle the kind of unstructured data quants struggle to pipe through their statistical models.
Natural language processing is a subfield of artificial intelligence where computers are programmed to analyse large amounts of text.
“In the past, quant managers have only dealt with structured data, which is about 20% of all the available data globally. The rest is unstructured data and a lot of that is text-based,” says Kunal Ghosh, a portfolio manager at Allianz, about the firm’s decision to start the NLP project.
The firm has been live for three and a half months using a sentiment signal from sell-side analyst reports, balanced for now with other tried and trusted signals. Allianz has contacted a dozen brokers to acquire their research history from 2008 onwards, to train its NLP model on data from the global financial crisis and subsequent recovery.
Once that is complete the firm “will be comfortable” using the sentiment signal as a standalone factor in its models, he says. The extra algo training is expected to take six to nine months.
Allianz is using recurrent neural networks, which remember and learn based on experience—as a child might touch a hot stove and learn that it should not—to analyse both the level and the trend in the sentiment. Ghosh describes the signal as a harbinger of an inflection point in asset prices.
“Sell-side reports have numerical parts and then there is the whole subjective text where the analyst writes down what they feel about the company. Quantitative managers have completely ignored that part. But often an analyst has a gut feeling that a company has reached its worst or best but does not have the numbers to back up their gut feeling,” he adds.
Allianz plans to progress to analysing earnings transcripts and news.
“There are three groups of people who influence a stock’s pricing or mispricing: sell-side analysts, company management and investors. Sell-side analysts’ mouthpiece is sell-side reports, company management’s mouthpiece is earnings transcripts. And investors don’t have a mouthpiece, but their actions reverberate through the news,” Ghosh says.
The firm is reaping rewards already from using sentiment scores in its best styles team, which runs a long-only equity factor investing strategy with over $35 billion.
There, Allianz derives an indicator of buy-side sentiment from fund holdings data, short-selling data and through the pricing of securities lending. Recently the firm has added company sentiment scores to the signal based on earnings call transcripts, boosting the efficacy of the combined signal by up to 15%.
According to Michael Heldmann, who heads quantamental strategy, Allianz is realistic about what it hopes to gain.
“I think a lot of the original alpha that you see in very long-term backtests for NLP signals has already diminished. Everybody that tries to get some pure alpha out of these things, [finds] it’s very difficult. What we are doing is different from that. We’re not trying to find real alpha, we’re aiming at a better definition of [risk premia].”
To generate the signal, Allianz analyses roughly 10,000 stocks daily or even intraday, which would not be possible without AI. The group uses an NLP algorithm to convert words and sentences into numbers, then uses supervised machine learning to derive either a sentiment number, a direct forecast of earnings surprises for the next earnings period, or more directly, a stock market return.
“You would need hundreds of analysts to listen to all earnings calls. But you can create an automated process that looks at earnings call transcripts right when the call happens and summarises it into a handful of numbers that capture context and sentiment. Those numbers can then be used to forecast the market’s reaction to the call,” Heldmann adds.
The best styles team is working to incorporate more NLP signals in its risk modelling, specifically around one-off events like Brexit. “A lot of people failed already at predicting the outcome of the Brexit referendum. We are not trying to forecast something like whether there’s going to be a hard Brexit. But if there’s an article that says, these five companies will heavily suffer if there is a hard Brexit, for example, that can indicate those companies have a higher exposure to the event and forward-looking risk management should take that into account,” he adds.
Further reading
Only users who have a paid subscription or are part of a corporate subscription are able to print or copy content.
To access these options, along with all other subscription benefits, please contact info@waterstechnology.com or view our subscription options here: http://subscriptions.waterstechnology.com/subscribe
You are currently unable to print this content. Please contact info@waterstechnology.com to find out more.
You are currently unable to copy this content. Please contact info@waterstechnology.com to find out more.
Copyright Infopro Digital Limited. All rights reserved.
As outlined in our terms and conditions, https://www.infopro-digital.com/terms-and-conditions/subscriptions/ (point 2.4), printing is limited to a single copy.
If you would like to purchase additional rights please email info@waterstechnology.com
Copyright Infopro Digital Limited. All rights reserved.
You may share this content using our article tools. As outlined in our terms and conditions, https://www.infopro-digital.com/terms-and-conditions/subscriptions/ (clause 2.4), an Authorised User may only make one copy of the materials for their own personal use. You must also comply with the restrictions in clause 2.5.
If you would like to purchase additional rights please email info@waterstechnology.com
More on Data Management
Moral models: The ethics of data management
The IMD Wrap: You may be managing data efficiently, but are you managing it ethically? And is that something you should be concerned about? Yes, says Max, you should.
Footsteps in the dark: Private markets’ data quality “problem”
Data quality in the private markets is poor. But is this a quirk of the market or a problem that needs fixing?
Deep in the heart of Texas, market data vets sweat the talent gap
The secrets to unlocking all of market data’s questions and the antidote for all its ills were discussed in Texas last week. Unfortunately, we promised not to tell—not all of them, anyway.
Fidelity’s quantum exploration unites theory and proof
The asset manager and Amazon have teamed to put a quantum twist on machine learning.
Interop after acquisitions remains daunting for buyers
Executives from a variety of vendors speaking at WFIC explained why desktop interoperability is important and why progress has been slow.
GenAI could connect ‘constellation’ of vital information technologies
At WFIC, Six Group’s Marion Leslie highlighted the opportunities and risks that the technology holds.
TRG Screen cuts 20 jobs following review and restructure
The review follows an eight-year period of acquisitions and buy-in from a new private equity owner last year.
Why don’t you write a book? Several notable data professionals are doing just that
The IMD Wrap: Max talks to some data architects-turned-authors who are literally sharing volumes of knowledge.