Banks and Bourses Increasingly Combine Cloud, AI for Data Projects
The combination of the two technologies is bearing fruit for firms struggling with legacy architectures, but education and talent remain tough obstacles to overcome.
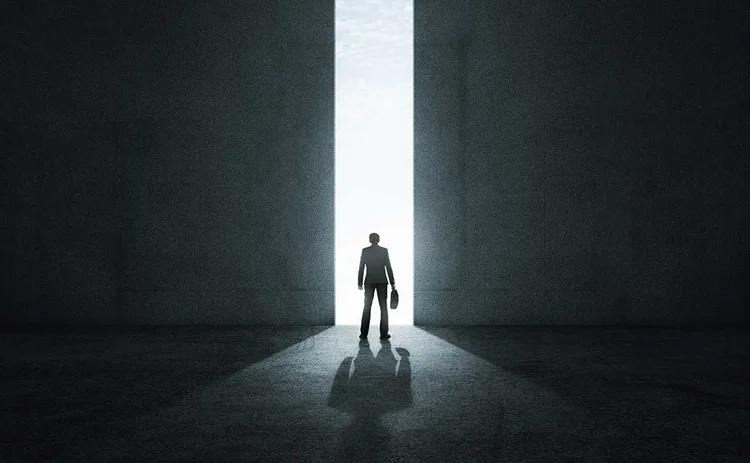
Silos, spreadsheets and silence are the enemies of modern data specialists. Many are hoping the advent of professional-grade emerging technologies will provide the key to organizing the vast amount of data within trading firms—perhaps once and for all.
These three aspects of data management—fiefdoms and closed verticals within technology estates, poor governance and disparate storage, and the inability of systems to talk to one another—are some of the most problematic areas for any business of scale within trading. Institutional firms struggle to bear the weight of their legacy infrastructures, not to mention the difficulty of pulling data from complex, fragmented systems, in many different formats.
Planning and implementing transformative projects to resolve these problems is a massive undertaking by any measure, one that takes multiple years to achieve. In many cases, firms are incrementally introducing automation and robotics to reduce some of the overhead costs of old systems, but others are using more sophisticated technologies such as artificial intelligence (AI) and the cloud to accelerate their data and technology transformation programs.
HSBC, for example, is implementing a large-scale project that entails using machine-learning technology to measure the quality of its data across five different dimensions—accuracy, completeness, uniqueness, validity, and consistency—and uses granular details to link correlated data together. It is no small task, as the firm is pulling information from multiple systems across several business lines and jurisdictions. The data will be viewable on data quality dashboards, where the user can view critical data elements and identify the real value of the data that the system aggregates. Chuck Teixeira, chief administrative officer and head of transformation at HSBC’s global banking and markets business, explains that its data management teams are leveraging AI to index and tag data from trillions of transactions and external sources to build a reusable golden source of data.
“Part of the challenge other banks and we have is that we have lots of data pools, but the problem is, if you don’t tag that data and index it, how do you find it again? So that is part of what we have built, a reusable data asset. And this has been a significant undertaking over the last year,” he adds.
In the second phase of its transformation project, HSBC will start to migrate the data to a cloud-based data lake in June to be able to utilize it for a variety of use cases. One of its principal objectives is to leverage new technology to accelerate operational processes and create new capabilities—such as building a client intelligence utility on the cloud. The platform will use the cleansed data, captured from trade lifecycles and external sources, to better understand the needs and requirements of its clients. It will ultimately act as a single part of a more comprehensive client services project, Phoenix, in which HSBC intends to collaborate with AI partners. The objective of this project is to develop more advanced algorithms and capabilities that will analyze and evaluate vast amounts of data from various sources, to predict client needs and improve user experiences.
“We will partner with firms to create an ecosystem and leverage the skills and experience from other technology firms to help build out our infrastructure,” says Teixeira.
Life-Long Learning
One of the major obstacles in any such undertaking, along with technical work, is the educational aspect of it—particularly when systemically important institutions such as stock exchanges plan a shift to the cloud.
Indeed, many are having to spend several months explaining to regulators how their data will be secured and managed in a virtual environment.
Two years ago, for instance, Euronext embarked on a project to revamp its data infrastructure to capture all of its data from its Optic trading engine and other applications.
“When we decided to go to the cloud, obviously a key element in the decision was around security. Compliance with regulation requires that we have the capacity to hold back or secure the data. We had to confirm that we will always have access to the data—and only Euronext,” says Nicolas Rivard, chief innovation officer at Euronext.
The exchange is porting the data to its cloud-based data lake on Amazon Web Services to store it in a structured manner. To date, it has migrated all its historical data, going back to 2007. At a later stage in the project, Euronext will use algorithms and AI to leverage the data for multiple use cases, such as market surveillance, compliance monitoring, advanced analytics, intraday alerts and to inform better technology offerings for its clients.
Skills Gap
Although cloud technology has been around for many years, trading firms, for the most part, have yet to decipher or become comfortable with the level of risk involved in moving valuable data or operations to an off-premises infrastructure. Another significant barrier for financial firms is attracting the right talent or expertise to execute such projects. As part of this skills gap, institutional firms must educate both existing teams and recruits on how to operate virtual environments or utilize emerging technologies.
“It’s a completely different approach to IT development, infrastructure and operations, which means that we had to train the IT team. We have to think differently about IT architecture, security, resource allocation. … Everything is code. This provides agility if you adapt and transform the way you used to build and run IT.” explains Rivard.
Only users who have a paid subscription or are part of a corporate subscription are able to print or copy content.
To access these options, along with all other subscription benefits, please contact info@waterstechnology.com or view our subscription options here: http://subscriptions.waterstechnology.com/subscribe
You are currently unable to print this content. Please contact info@waterstechnology.com to find out more.
You are currently unable to copy this content. Please contact info@waterstechnology.com to find out more.
Copyright Infopro Digital Limited. All rights reserved.
As outlined in our terms and conditions, https://www.infopro-digital.com/terms-and-conditions/subscriptions/ (point 2.4), printing is limited to a single copy.
If you would like to purchase additional rights please email info@waterstechnology.com
Copyright Infopro Digital Limited. All rights reserved.
You may share this content using our article tools. As outlined in our terms and conditions, https://www.infopro-digital.com/terms-and-conditions/subscriptions/ (clause 2.4), an Authorised User may only make one copy of the materials for their own personal use. You must also comply with the restrictions in clause 2.5.
If you would like to purchase additional rights please email info@waterstechnology.com
More on Data Management
‘We started late’: Oracle makes case for its market data cloud offering
Executives from Oracle, LSEG, and CJC detailed the ‘eye-opening’ performance and latency of the Oracle Cloud Infrastructure.
From frozen assets to fire sales: The datasets to prevent your investments going up in smoke
The IMD Wrap: As severe weather conditions become more commonplace, Max wonders which datasets will prove most useful for those navigating a changing world.
Opra considers ‘dynamic load balancing’ for options market
The data distributor recently completed a challenging project to build a 96-line feed. This new endeavor could prove just as challenging (but perhaps necessary) for the industry that will use it.
Market data for private markets? BlackRock sees its big opportunity
The investment giant’s CEO said he envisions a far bigger private market business in 2025.
Bloomberg debuts GenAI news summaries
The AI-generated summaries will allow financial professionals to consume more data, faster, officials say.
Substantive Research reveals new metrics for market data negotiations framework
The research firm will make its industry-derived project available for public consumption next month.
As the ETF market grows, firms must tackle existing data complexities
Finding reliable reference data is becoming a bigger concern for investors as the ETF market continues to balloon. This led to Big xyt to partner with Trackinsight.
Artificial intelligence, like a CDO, needs to learn from its mistakes
The IMD Wrap: The value of good data professionals isn’t how many things they’ve got right, says Max Bowie, but how many things they got wrong and then fixed.