This article was paid for by a contributing third party.

AI’s Next Phase – Thriving Through Implementation
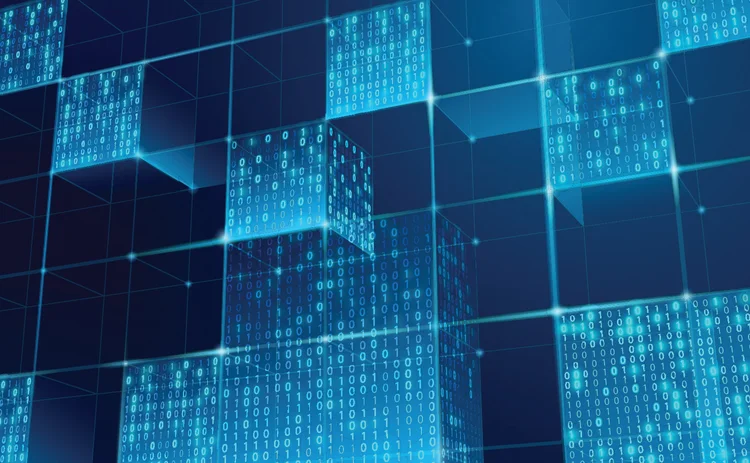
Banks and asset managers experimenting with AI have come through the early stages with enthusiasm, but, as with the peloton in the Tour de France, they are beginning to feel some strain in their legs. For many, arduous new terrain still awaits before reaching the finish line. As one contributor to the WatersTechnology/SmartStream discussion described it: “The struggle at the moment remains being able to articulate a business case for AI; hence the reason people are doing more proof of concepts to trial it—to help define the business case, to then move forward with its applicability within the industry.” We are at the foot of the Alps, staring up at the real climb.
The industry has already cast a wide net across both functional areas and AI’s various gradations, from robotics processing automation for fraud detection, document digitalization and help-desk chatbots, up to clustered machine learning algorithms for coding and automated commodities trading on predictive analysis of geography and weather patterns. Some in the discussion described targeted pockets of innovation, while others said AI has permeated multiple corners of the enterprise, be they client-facing, trade settlement operations or investment research. As companies contemplate further investment or moving proof of concepts into full production, the evaluative criteria seem familiar, too: What are the cost savings in play? What new revenue will be generated? Are there additional efficiencies to capture, and will they incur new regulatory costs?
But speakers also agreed on some wrinkles unique to AI. Specifically, while some still prefer the “short, quick wins” achievable in robotics, the real value—and likewise, the real technical challenge—lies in AI being exposed to troves of information that would otherwise lie untouched, acquiring signals and then creating the proper funnel and guardrails for what AI does with it. The next phase, therefore, isn’t just about traditional metrics; it is about ambition, timeliness and navigating ethical and liability questions. And at the center of this lies the effective marshalling of data.
Battling Incrementalism
The obvious place to start is measuring institutional ambition, and, interestingly, the gathering had a strong chorus of voices urging the business to be bolder. As one voice in that chorus said, today’s trap is in creating a false choice: one is short, more cost-effective and certain in outcome; the other is more strategic but far more involved and open-ended. “For some of our stakeholders, it’s still about that uncertainty,” she explained. “They look at the decision as ‘I know this will happen; I’ll choose the short-term option.’ That’s where you lose the battle.”
Many said this drive to deliver small gains was understandable, but it also shades AI’s potential, particularly given the investment already put in to big data, data lakes and related infrastructure in recent years. For instance, one firm is trying to reduce errors and identify outliers in large index datasets, which would be impossible to run without AI due to their sheer size. Another attendee, whose insurance firm already uses AI for fraud detection, argued that the greatest benefit is in manipulating data not being used at all, “looking at things that get discarded or ignored completely. Logs and other activities are just by the wayside,” he said. “It’s integrating those with your core datasets to get the patterns that you can’t see, and that a human wouldn’t be able to see because the data is too obtuse currently. That’s where we see the largest strides to be made.”
Indeed, this opinion leaned most into the “known unknown” problems firms face—whether in processing efficiencies or business intelligence—that require more intuition of the algorithm or neural network in play. The meeting considered yet another example: one of a potential corporate recovery team application. “When you have a client that fails, at the end there is a ‘lessons learned’ analysis we do, and it sits on a PDF scanned in from 10 years ago,” the event heard. “There’s no application right now where we can query: ‘We’ve got a deal with an oil firm in this particular country in front of us. AI, find the things that have gone wrong with this kind of investment structure in the past—in oil, in this region, or factors that might be linked to it.’ That’s the kind of thing I think we should be aiming for, even if it turns out to be quite difficult. But the message I’m getting from senior management is that they’re really not looking beyond what they see as an incremental move upon ‘normal’ IT.”
Time Factors—First Nuggets and Fine-Tuning Models
Ascending from bot-based task automation to machine learning-fueled research brings its own pitfalls, and the trickiness lies in sequencing and timeliness. The temptation, one speaker said, is that firms know “they need that first nugget of something you can prove works to generate interest and be able to sell it.” But this initial output can also skew expectations, if not torpedo the project over time.
“Until your model is playing well, it won’t give you information on whether your decision can be taken, and our experience is that this journey, and the positioning of those outcomes, takes time,” posited a speaker from a firm using AI to predict seasonal oil output. “It took us a good eight months to come to an AI-driven model with a prediction within 18% of a human analyst’s estimate. You can call initial results a beginning, but we knew we had to go through and refine those models, and by the time you reach that outcome, you also need to be conscious of the time elapsed and whether the initial outcome or purpose is still valid.”
From initial buildout to attenuation to measuring against original objectives, it was agreed that data lies at the heart of these more complex implementations, and again, no matter how great the AI, issues can percolate below and quickly stress the entire data estate. “It is a common problem we’ve all heard: ‘Is our market data in a form where we can use it from day one?’” another attendee asked. “In most cases, I don’t think so. There is a lot companies do in-house to cleanse that market data today. That’s a huge effort companies invest to look at data quality, the data we need, the data history, and the gaps in that history. That is the bigger problem. Until we get those building blocks correct, I don’t believe AI would be able to give us efficient outcomes, because then we’ll only be investing our time on remodelling and defining AI’s parameters.”
The good news is that, unlike AI—and for reasons often secular and apart from AI—many progressive institutions have invested heavily in transformative data initiatives and chief data officers, and are better prepared than ever to support impactful AI-backed endeavors. The fact remains, though, that this will simply make things easier, rather than simple or fast. And clearly, firms are keen to observe the difference.
Frontiers in Explainability and Bias
Likewise, a final critical challenge highlighted at the session was the ethical and legal context surrounding AI, gaining visibility as it has among boardrooms and, increasingly, regulatory authorities. Examples were raised of sophisticated fraud detection and similar AI-backed systems that were successfully developed and enthusiastically supported by banks, only for them to be shelved. “They simply determined: ‘We’re not going to get this past the regulators’,” said one person familiar with a recent case. “‘It works perfectly for our purposes’, they said. ‘It’s much better than the current process, but it was a neural network with a non-explainability problem. We’re not going to go live with it, because the regulators just won’t like it’.”
Others pointed out that negotiating with vendors who may be reluctant to expose proprietary AI methodology, or even assume liability for it, could prove an internal point of contention as well. Still more trickiness appears in coralling the use of AI-generated metadata, guaranteeing customers’ right to have personal identifiers forgotten—as privacy directives have increasingly demanded—and correct biases that machines have already learned. All of these considerations must be teased out, and implementing proper data governance around them is a significant undertaking in itself. In an era of bombshell headlines highlighting data misuse and algos operating in the dark, they are rapidly becoming essential pieces to be planned for on day one, rather than tossed in as an afterthought—when it may be too late.
Final Thoughts
This year’s event nicely detailed the contrasts—and occasional contradictions—engendered by AI’s next financial services wave. Popular fascination could not be higher with AI, but still many say they are dabbling too much on the edges. Technologists conjure myriad creative uses for AI’s higher forms to add value within their enterprises, not only on automation but also for genuine, scalable insight. Yet they also acknowledge that the hit rate for these projects is unpredictable at best, and is hard to cost-justify in the short term until we know more about what works, how well and for how long. Meanwhile, the milieu around AI only grows more complicated as valid concerns are raised about what it should do, and how closely it can be explained and controlled.
“Because there is a nervousness about AI within our organisation, like elsewhere, we’re very much focused on a combination of AI and human interaction,” as one participant summed it up. “That’s the sweet spot. It’s about reducing the amount of human intervention, so that a human can really focus on the ‘value’ piece.”
In 2019, that may not be quite enough, even if it may just be the limit of what is practically doable for many firms today. What’s clear is that the next leap—the most persuasive, timely, and suitable AI applications—will fit hand-in-glove with the data sources, processes, and governance frameworks humming alongside them.
Sponsored content
Copyright Infopro Digital Limited. All rights reserved.
You may share this content using our article tools. Printing this content is for the sole use of the Authorised User (named subscriber), as outlined in our terms and conditions - https://www.infopro-insight.com/terms-conditions/insight-subscriptions/
If you would like to purchase additional rights please email info@waterstechnology.com
Copyright Infopro Digital Limited. All rights reserved.
You may share this content using our article tools. Copying this content is for the sole use of the Authorised User (named subscriber), as outlined in our terms and conditions - https://www.infopro-insight.com/terms-conditions/insight-subscriptions/
If you would like to purchase additional rights please email info@waterstechnology.com