Taking the Measure of Big Data
Strapline: Interview With
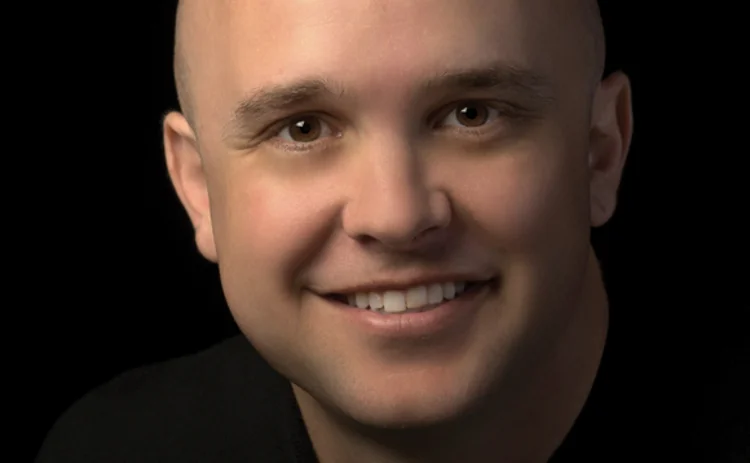
With the concept of “big data” making waves in the reference data world, particularly the questions about how to harness the powers of cloud computing resources and the Apache Hadoop open source data management standard, financial services firms looking at big data problems are likely also looking for outside help.
The financial services and institutions unit of chip-maker Intel works with partners who devise solutions to operational issues such as big data, notably independent software vendors (ISVs) such as Oracle and SunGard. Part of this work is making sure Intel chips are suited for these solutions and are used in them.
One big data problem is monitoring and detecting trade patterns in high-frequency trading at a co-location facility, says Daryan Dehghanpisheh, director of the financial services and institutions unit at Intel, raising this issue as an example. A firm might then want to catalog these trades and send the data to a cloud for analysis in near real-time. “That’s going to stress the computational capabilities, the memory system and the I/O system,” says Dehghanpisheh. “How do you write software to manage and minimize those stresses, so you can get the speed and time advantages back in your favor for whatever technique or trading element you’re looking at?”
The big data issue, as Dehghanpisheh sees it, is really “moving from causal-based analysis into a predictive analysis, so your data in real-time becomes more useful, so you can find the nuggets you’re looking for.”
Another challenge of big data is the additional sources of data that are making the volume of data much larger than before. Dehghanpisheh points to increased uses of search and monitoring of social media communications concerning companies and their securities, as a major source of new data, and says his group at Intel is looking at ways to address that.
“Financial firms are used to storing massive amounts of tick data and back-testing strategies. What happens when you throw in a mix of human behavior, like indexing Google searches?” he asks. “They may be able to store search characteristics and histories, but still have to figure out a way to search and analyze it. That changes the scope and scale.” Firms have a similar issue with figuring out handling of Twitter data, he notes. “Everyone is trying to store Twitter feeds and figure out what they have,” says Dehghanpisheh. “This is a change to the type of data we try to analyze and the speed at which that data is generated.”
Processing Speed and Time Become Factors
Dehghanpisheh also points out big data is not just defined by the size of data involved, and this creates another challenge Intel’s financial services unit seeks to address. “If you say big data is anything crossing a terabyte of data, that doesn’t do it justice,” he says. “You could have a data set or table that exists for quite a while and it’s become large. Serving it becomes fairly easy.”
The speed of data growth is the other characteristic that defines big data, Dehghanpisheh explains. “The big characteristic of big data is the time scale—how fast your data grows is what a big data problem is,” he says. “My team’s perspective is to look on a sub-second level. If data is growing at a certain size, or we’re analyzing a certain amount of data below that 1 second threshold, we’re getting pretty close to real time. The human brain can only recognize something through its neurons in about 0.002 seconds. But in that time, the machine world can generate, consume or analyze a tremendous amount of data, far more than what the human brain can potentially hold. So we had to break it down from a time perspective. That can work with you and against you in generating the data, analyzing the data and using it.”
In financial services, “time is money,” of course, as Dehghanpisheh puts it, and often there isn’t an unlimited amount of time to search an unlimited amount of data. As a result, Intel, firms and the industry are trying to build systems that are more scalable. “Data growth as we know it is not slowing down,” he says. “It becomes very hard to create systems that can model it and deal with it.”
Only users who have a paid subscription or are part of a corporate subscription are able to print or copy content.
To access these options, along with all other subscription benefits, please contact info@waterstechnology.com or view our subscription options here: http://subscriptions.waterstechnology.com/subscribe
You are currently unable to print this content. Please contact info@waterstechnology.com to find out more.
You are currently unable to copy this content. Please contact info@waterstechnology.com to find out more.
Copyright Infopro Digital Limited. All rights reserved.
As outlined in our terms and conditions, https://www.infopro-digital.com/terms-and-conditions/subscriptions/ (point 2.4), printing is limited to a single copy.
If you would like to purchase additional rights please email info@waterstechnology.com
Copyright Infopro Digital Limited. All rights reserved.
You may share this content using our article tools. As outlined in our terms and conditions, https://www.infopro-digital.com/terms-and-conditions/subscriptions/ (clause 2.4), an Authorised User may only make one copy of the materials for their own personal use. You must also comply with the restrictions in clause 2.5.
If you would like to purchase additional rights please email info@waterstechnology.com
More on Data Management
Chief data officers must ‘get it done’—but differ on what that means
Voice of the CDO: After years of focus on data quality, governance, and compliance, CDOs are now tasked with supporting the business in generating alpha and driving value. How can firms put a value on the CDO role?
In a world of data-cost overruns, inventory systems are a rising necessity
The IMD Wrap: Max says that to avoid cost controls, demonstrate the value of market data spend.
S&P debuts GenAI ‘Document Intelligence’ for Capital IQ
The new tool provides summaries of lengthy text-based documents such as filings and earnings transcripts and allows users to query the documents with a ChatGPT-style interface.
As NYSE moves toward overnight trading, can one ATS keep its lead?
An innovative approach to market data has helped Blue Ocean ATS become a back-end success story. But now it must contend with industry giants angling to take a piece of its pie.
AI set to overhaul market data landscape by 2029, new study finds
A new report by Burton-Taylor says the intersection of advanced AI and market data has big implications for analytics, delivery, licensing, and more.
New Bloomberg study finds demand for election-related alt data
In a survey conducted with Coalition Greenwich, the data giant revealed a strong desire among asset managers, economists and analysts for more alternative data from the burgeoning prediction markets.
Waters Rankings 2024 winner’s interview: S&P Global Market Intelligence
S&P Global Market Intelligence won two categories in this year’s Waters Rankings: Best reporting system provider and Best enterprise data management system provider.
How ‘Bond gadgets’ make tackling data easier for regulators and traders
The IMD Wrap: Everyone loves the hype around AI, especially financial firms. And now, even regulators are getting in on the act. But first... “The name’s Bond; J-AI-mes Bond”