Shedding Light on Dark Data
Making connections among sources yields predictive information
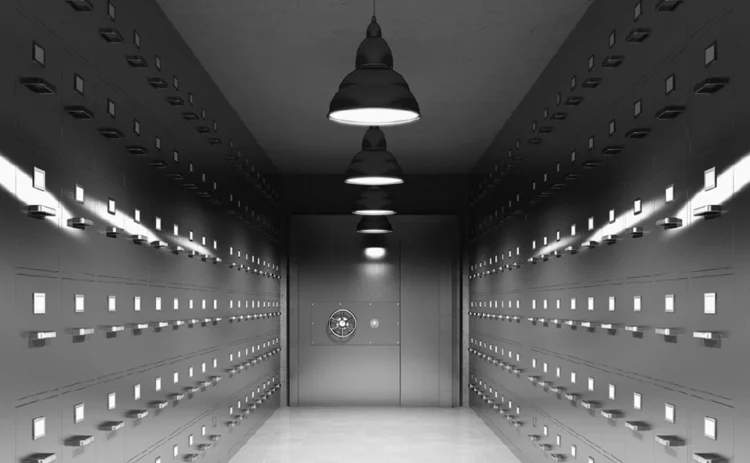
The term "dark data" and what it represents is being raised more often lately by industry service providers and consultancies as an issue of concern. With this increased attention, some are uncertain about what exactly "dark data" means in the context of financial services industry data management.
Dark data shouldn't be understood literally, as though it were about using data for "nefarious purposes," says Charles Fiori, a consultant with long industry experience at firms and service providers
Only users who have a paid subscription or are part of a corporate subscription are able to print or copy content.
To access these options, along with all other subscription benefits, please contact info@waterstechnology.com or view our subscription options here: https://subscriptions.waterstechnology.com/subscribe
You are currently unable to print this content. Please contact info@waterstechnology.com to find out more.
You are currently unable to copy this content. Please contact info@waterstechnology.com to find out more.
Copyright Infopro Digital Limited. All rights reserved.
As outlined in our terms and conditions, https://www.infopro-digital.com/terms-and-conditions/subscriptions/ (point 2.4), printing is limited to a single copy.
If you would like to purchase additional rights please email info@waterstechnology.com
Copyright Infopro Digital Limited. All rights reserved.
You may share this content using our article tools. As outlined in our terms and conditions, https://www.infopro-digital.com/terms-and-conditions/subscriptions/ (clause 2.4), an Authorised User may only make one copy of the materials for their own personal use. You must also comply with the restrictions in clause 2.5.
If you would like to purchase additional rights please email info@waterstechnology.com
More on Data Management
Exchange M&A, US moratorium on AI regs dashed, Citi’s “fat-finger”-killer, and more
The Waters Cooler: Euronext-Athex, SIX-Aquis, Blue Ocean-Eventus, EDM Association, and more in this week’s news roundup.
EDM Council expands reach with Object Management Group merger
The rebranded EDM Council now includes members from industries outside financial services.
As datacenter cooling issues rise, FPGAs could help
IMD Wrap: As temperatures are spiking, so too is demand for capacity related to AI applications. Max says FPGAs could help to ease the burden being forced on datacenters.
Bloomberg introduces geopolitical country-of-risk scores to terminal
Through a new partnership with Seerist, terminal users can now access risk data on seven million companies and 245 countries.
A network of Cusip workarounds keeps the retirement industry humming
Restrictive data licenses—the subject of an ongoing antitrust case against Cusip Global Services—are felt keenly in the retirement space, where an amalgam of identifiers meant to ensure licensing compliance create headaches for investment advisers and investors.
LLMs are making alternative datasets ‘fuzzy’
Waters Wrap: While large language models and generative/agentic AI offer an endless amount of opportunity, they are also exposing unforeseen risks and challenges.
Cloud offers promise for execs struggling with legacy tech
Tech execs from the buy side and vendor world are still grappling with how to handle legacy technology and where the cloud should step in.
Bloomberg expands user access to new AI document search tool
An evolution of previous AI-enabled features, the new capability allows users to search terminal content as well as their firm’s proprietary content by asking natural language questions.