Max Bowie: A ‘Search Party’ to Find Missing Alpha in Financial Data
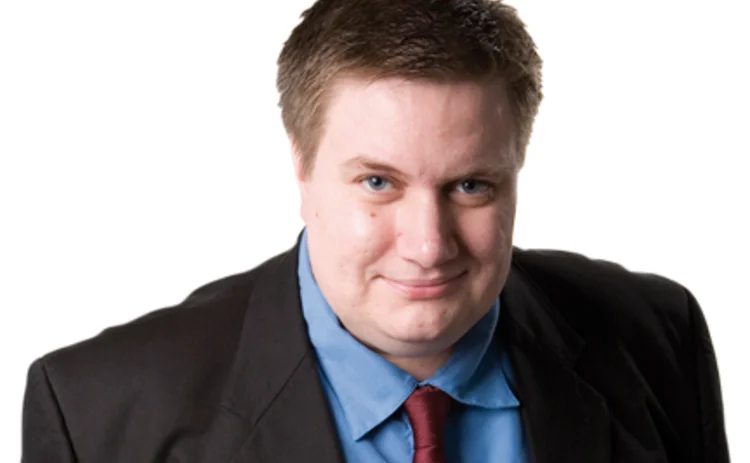
The market data industry is no stranger to high volumes of data, as rising message rates driven by a combination of higher-frequency trading, market volatility, decimalization and other market forces threaten to overwhelm the data and trading infrastructures of trading venues and market participants alike. While many efforts have focused on bolstering the capacity of their infrastructures, an equal amount of effort has been spent on analytical tools to process and filter these increased volumes of data to derive useful insight—i.e., the more data you have, the more tools you need to make it useful. Or, mo’ data, mo’ problems.
To get the most out of structured data, firms are employing new database technologies to process, store, correlate and retrieve Big Data faster than traditional databases. Meanwhile, non-price data volumes are also increasing as firms try to derive value from semi-structured and unstructured data, and look for ways to leverage data hidden within their enterprise, and harness public information available on the internet.
For these functions, a new breed of search tools is emerging to provide broader information search and discovery. For example, Epam’s InfoNgen business developed an appliance for internal content discovery and aggregation in 2009 to capture data held on internal servers, in email messages, broker research and other documents, or the internet, which could then be combined with other data. Only a few years ago, complex-event processing engines emerged as a lightweight way to monitor multiple data streams to identify optimal trading circumstances. But now, the influx of new data sources that firms believe will deliver a trading edge is prompting interest in more heavyweight data-processing solutions, such as IBM’s Watson supercomputer, which can be used to process vast volumes of data for investment analysis or to gain a better view of risk associated with clients, for example. But Watson also features natural-language processing capabilities that enable it to understand unstructured data, while the platform is also self-learning, so the more data you give it, the better results it generates. Or, mo’ data, mo’ money.
Natural-language processing serves dual purposes: On one hand, it allows computers to understand unstructured data—such as text content like news, research, blogs or social media posts—rather than merely numerical values, thus providing context and nuances to figures that may not tell the whole story. On the other hand, it can also allow traders to query today’s vast amounts of data using plain English, rather than having to remember combinations of codes or shortcuts. For example, Thomson Reuters designed its Eikon terminal with this in mind: that tomorrow’s traders would be more familiar with web searches than with the clunky function keys of olde. Its latest iteration, version 3.0, incorporates natural-language search for querying the terminal’s data and analytics. So if you type “Apple Samsung 2001 to 2012 market cap and macd” into Eikon, the system will display moving average convergence/divergence charts for both symbols on a single graph.
Thomson Reuters isn’t alone. Wolfram Research released its Wolfram Alpha online “computational knowledge engine” in 2009, while Austin, Texas-based financial search startup 9W Search enables financial advisers, analysts and researchers to search and compare company financial data from public sources filings, and other data sourced from Edgar Online. Another newcomer to financial search is Quandl, which started 2013 having indexed 2 million freely-available financial and economic time-series datasets, statistics and indicators from exchanges, clearinghouses, trade organizations, media outlets, central banks, regulators and government bodies—and recently doubled its data coverage—and provides for free what other vendors charge to deliver, making it both appealing and disruptive.
The influx of new data sources that firms believe will deliver a trading edge is prompting interest in more heavyweight data-processing solutions.
Now imagine if these services were all made available within the app store of a web-based terminal and tied together with a unified search mechanism that links and cross-references their data. Because that’s where I believe the financial data desktops of the future are headed, with search as a central component.
Only users who have a paid subscription or are part of a corporate subscription are able to print or copy content.
To access these options, along with all other subscription benefits, please contact info@waterstechnology.com or view our subscription options here: http://subscriptions.waterstechnology.com/subscribe
You are currently unable to print this content. Please contact info@waterstechnology.com to find out more.
You are currently unable to copy this content. Please contact info@waterstechnology.com to find out more.
Copyright Infopro Digital Limited. All rights reserved.
As outlined in our terms and conditions, https://www.infopro-digital.com/terms-and-conditions/subscriptions/ (point 2.4), printing is limited to a single copy.
If you would like to purchase additional rights please email info@waterstechnology.com
Copyright Infopro Digital Limited. All rights reserved.
You may share this content using our article tools. As outlined in our terms and conditions, https://www.infopro-digital.com/terms-and-conditions/subscriptions/ (clause 2.4), an Authorised User may only make one copy of the materials for their own personal use. You must also comply with the restrictions in clause 2.5.
If you would like to purchase additional rights please email info@waterstechnology.com
More on Data Management
As NYSE moves toward overnight trading, can one ATS keep its lead?
An innovative approach to market data has helped Blue Ocean ATS become a back-end success story. But now it must contend with industry giants angling to take a piece of its pie.
AI set to overhaul market data landscape by 2029, new study finds
A new report by Burton-Taylor says the intersection of advanced AI and market data has big implications for analytics, delivery, licensing, and more.
New Bloomberg study finds demand for election-related alt data
In a survey conducted with Coalition Greenwich, the data giant revealed a strong desire among asset managers, economists and analysts for more alternative data from the burgeoning prediction markets.
Waters Rankings 2024 winner’s interview: S&P Global Market Intelligence
S&P Global Market Intelligence won two categories in this year’s Waters Rankings: Best reporting system provider and Best enterprise data management system provider.
How ‘Bond gadgets’ make tackling data easier for regulators and traders
The IMD Wrap: Everyone loves the hype around AI, especially financial firms. And now, even regulators are getting in on the act. But first... “The name’s Bond; J-AI-mes Bond”
Waters Wavelength Ep. 293: Reference Data Drama
Tony and Reb discuss the Financial Data Transparency Act's proposed rules around identifiers and the industry reaction.
Industry not sold on FIGI mandate for US reg reporting
Banks’ and asset managers’ tortured relationship with Cusip numbers remains tortured, as they tell regulators to keep the taxonomy in play.
Waters Wavelength Ep. 292: Fencore’s James Crosby
James Crosby joins the podcast to talk about the evolution of buy side tech and data decisions.