AFTAs 2019 Winners' Circle: AxiomSL: Transparency Is the Key
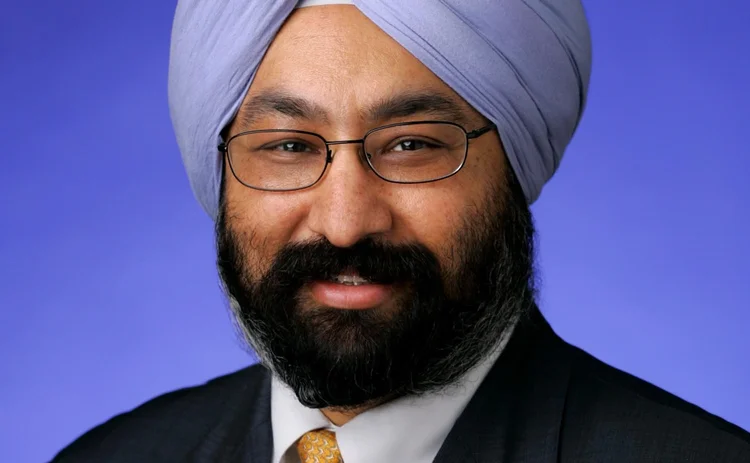
What are the problems ControllerView is currently solving for its capital markets clients?
Harry Chopra, chief client officer, AxiomSL: The first is transparency in the calculations we perform for our clients. Other systems on the market are “black box”—you don’t know how your risk-weighted asset numbers are being calculated. Business users have no clue as to what’s going on. We provide a completely transparent mechanism through visual business rules so that users know exactly how their numbers are being calculated.
With respect to the transparency you describe, does ControllerView allow users to drill down into the methodologies underpinning the calculations so that they are comfortable that the numbers are reliable and accurate?
Chopra: Absolutely. We also add lineage to the transparency in the calculations so that users know exactly where the numbers are coming from. We also help firms optimize their collateral. If they’re going to take a large haircut, we have algorithms that help them understand how they can improve their tier-1 capital ratios.
So this is not just about firms ensuring that they are complying with their regulatory mandates? This is also about them operating as efficiently as possible with respect to their capital ratios?
Chopra: Correct. And the third element is performance. Typically, these calculations can take up to five to seven days, whereas with ControllerView they can be done in a few hours. With Basel IV, banks have to do myriad calculations to understand what’s on their balance sheets, and they need to get these calculations right. So speed, accuracy and transparency will become even more important.
How does ControllerView manage those complex calculations in relatively short timeframes? What is the really clever stuff that AxiomSL is doing under the hood that allows capital markets firms to manage these calculations on an intra-day basis?
Chopra: The technology is built on Java and is totally database architecture-agnostic. We allow banks to run multiple processes in parallel, which means we can scale on a horizontal basis. The other element is that if banks’ data becomes very large, they can scale on the latest non-relational database technology—for example Hadoop—and we also allow them to do in-memory processing. We insulate banks from all the different technology elements—all they have to worry about is their transparency. We take care of everything else.
How is ControllerView delivered? Is it cloud-based or is it only available as an in-house run platform?
Chopra: We offer both models. We took two years to build out an ISO 27001 certification so that we could assure clients in terms of cybersecurity. I was at RiskMinds recently in Amsterdam and the biggest risk from every CRO’s perspective was cybersecurity. We’ve also certified our cloud ops team for SOC 2 with Deloitte, which guarantees that our technology and procedures are in order. We now have about 15 clients in the cloud.
What is unique about ControllerView with respect to the functionality it provides capital markets firms?
Chopra: There are a number. The first is native data ingestion. We can ingest data from any source and no other company can do that because they all have pre-conceived ideas of what a customer’s data should look like. The second is visual business rules and the third is transparency with respect to the calculations we perform. And obviously we can scale horizontally and vertically. Finally, we build all the regulatory templates that have to be filed and we keep track of those regulatory requirements.
What’s on the AxiomSL workbench for 2020? What can your clients expect in terms of new features and functionality?
Chopra: We’re currently working on formalizing our Apache Spark architecture—we’re finding that clients are looking to implement ControllerView out of the box rather than having to configure it. So we’re figuring out how best to offer our clients in-memory processing. We’ve also just identified an appropriate machine learning use-case. The way the system works is that when data is ingested, we have a pretty good idea of what it should look like. We then use machine learning technology to identify outliers in the data, which means we can significantly improve data quality as it flows into the system.
Only users who have a paid subscription or are part of a corporate subscription are able to print or copy content.
To access these options, along with all other subscription benefits, please contact info@waterstechnology.com or view our subscription options here: http://subscriptions.waterstechnology.com/subscribe
You are currently unable to print this content. Please contact info@waterstechnology.com to find out more.
You are currently unable to copy this content. Please contact info@waterstechnology.com to find out more.
Copyright Infopro Digital Limited. All rights reserved.
You may share this content using our article tools. Printing this content is for the sole use of the Authorised User (named subscriber), as outlined in our terms and conditions - https://www.infopro-insight.com/terms-conditions/insight-subscriptions/
If you would like to purchase additional rights please email info@waterstechnology.com
Copyright Infopro Digital Limited. All rights reserved.
You may share this content using our article tools. Copying this content is for the sole use of the Authorised User (named subscriber), as outlined in our terms and conditions - https://www.infopro-insight.com/terms-conditions/insight-subscriptions/
If you would like to purchase additional rights please email info@waterstechnology.com
More on Awards & Rankings
Witad Awards 2024: Technology innovator of the year (vendor)—Christy Bremner, SS&C Technologies
Christy Bremner, senior vice president, Institutional & Investment Management (I&IM) division at SS&C Technologies, wins technology innovator of the year (vendor) in the 2024 Women in Technology and Data Awards.
Witad Awards 2024: EDM professional of the year—Neslihan Yegul, S&P Global Market Intelligence
Neslihan Yegul, vice president, head of EDM and thinkFolio at S&P Global Market Intelligence, wins EDM professional of the year in the 2024 Women in Technology and Data Awards.
Witad Awards 2024: Technology innovator of the year (end-user)—Laura Hamilton, Bank of America
Laura Hamilton, global head of corporate treasury, credit risk, climate risk, and financial forecasting technology at Bank of America, wins technology innovator of the year (end-user) in the 2024 Women in Technology and Data Awards.
Witad Awards 2024: Vendor professional of the year (data and operations)—Joanna Davies, OSTTRA
Joanna Davies, head of trade processing at OSTTRA, wins vendor professional of the year (data and operations) in the 2024 Women in Technology and Data Awards.
Sell-Side Technology Awards 2024: Best sell-side front-office platform—Bloomberg
Product: Bloomberg TOMS
Most read
- Chris Edmonds takes the reins at ICE Fixed Income and Data Services
- DTCC urges affirmation focus ahead of T+1 move
- FactSet looks to build on portfolio commentary with AI